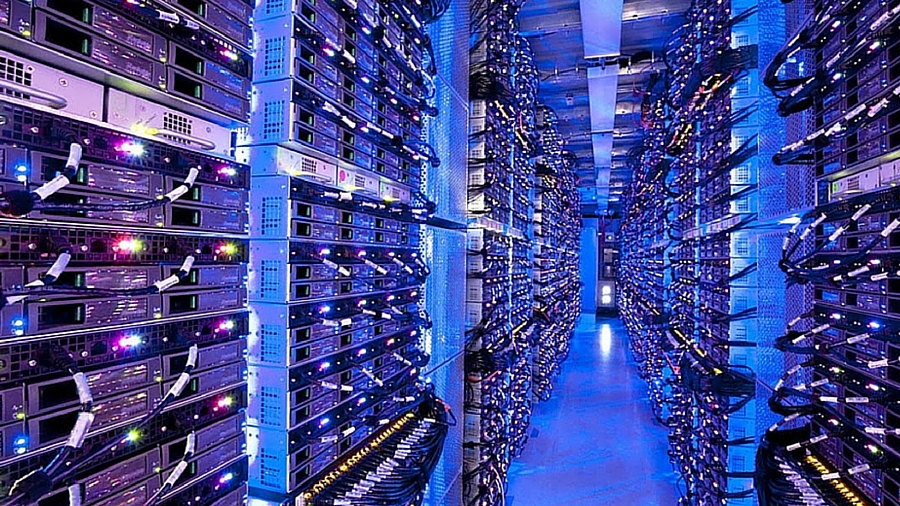
In an era when organizations are collecting ever larger pools of data and deriving information from an increasingly complex array of sources, managing the data supply chain has become a vital function of daily operations in industries as diverse as manufacturing, healthcare, and finance. Data has never been more essential to businesses, but without a strong data supply chain program in place, companies lack the ability to leverage outside information in a useful way.
Defining supply chains of data
To understand the concept of data supply chains, first imagine traditional supply chain management, which includes the handling of the production and flow of goods and services – beginning with raw materials to the delivery of the finished product. Similarly, data supply chains consist of three stages:
- First, starting at the source of origination, supply chains of data track the lineage of the data
- After data is extracted, it can then be enriched, controlled, improved, and made available in a searchable format, allowing the end-user to access it, query it and put it to good business use
- Finally, the data can be consumed as a data product, such as a financial data mart, customer or product 360
Managing supply chains of data
In the past, the data mostly came from operational data systems, either generated from within customer relationship management (CRM) and enterprise resource planning (ERP) systems or other products that serve businesses as transactional systems. Under this model, the data supply chain was less complex and more easily manageable using a single model.
But in the last five to seven years, especially in the wake of the pandemic, companies understand that they cannot survive on just the data they generate internally. This evolution – the explosion in external data sources – is causing organizations to think about their data using two discrete data supply models: direct and indirect. The direct model consists of:
- First-party data: information collected directly through CRM, ERP, end-user devices and other internal systems of record
- Second-party data: data from partners, vendors and consumer devices
- Third-party data: data from sources such as customer purchase histories, intelligence lists, market data, economic data, etc. The indirect model addresses:
- Causal data: data points that could enrich existing data models, such as weather as an indicator of customer sentiment, demographics, social media, trends, etc.
- Synthetic data: simulated and auto-generated data. For example, autonomous vehicle simulators need volumes of data that’s not readily available and need to evaluate real-world scenarios that have not been captured.
Adapting to a new data world
So how should organizations adapt to this evolution? With the needs of the enterprise becoming much more interconnected — not only within the company, but also among suppliers, producers and consumers – enabling the direct model and indirect model to interact with each other requires a modern infrastructure, invariably trending toward multicloud.
Today, company data is distributed across multiple clouds and SaaS applications. Organizations cannot rely on internal data alone to enable better decisions. Rather they must rely more on external data sets from global partners, suppliers and consumers and causal data that interprets the environment surrounding what the organization sells and who they sell to.
To adapt to the data supply chain management evolution, organizations should expand their supply chain of data to leverage all three forms of direct data sets: first-party, second-party, and third-party data. They should also focus on increase the adoption of indirect data sets, both causal and synthetic data. Using both data supply models, organizations can build and leverage modern data architectures and data integration patterns. To get started on the data supply chain management journey, a good first step is developing a data “playbook” that institutes data management best practices while also engaging analytics teams and transformational processes to drive growth. From there, organizations can start to build and leverage more modern data architectures and data integration patterns while maintaining a focus on data that is most critical to the enterprise.