On July 28, 2022, Google’s DeepMind released the structure of 200 million proteins, literally everything that exists. This is said to be the most important achievement of AI ever, namely a ‘solution’ to the protein-folding problem.
Proteins are composed of a linear chain of amino acids and their 3D structures determine their functions. Structure determination is laborious. One way to know the optimal folded structure of the protein computationally is to sample all its possible configurations, composed of specific angles between peptide bonds. However, this is an impossible task as a typical protein may have about 10300 configurations and even if a million of them were examined per second, the overall time needed will be unimaginable. That helped save about 1,000 million man-years.
DeepMind’s AlphaFold made an important breakthrough in 2020. It accurately predicted the structures of about 100 proteins to atomic resolution, and no other solution came close to this feat. Many believe that the protein-folding problem is over.
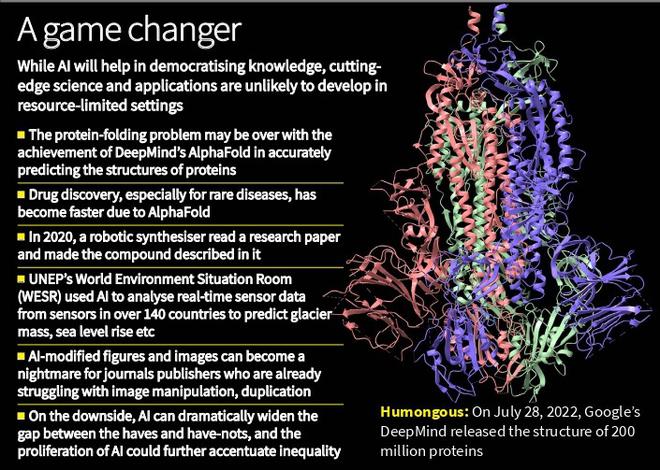
Besides publishing the work in Nature, DeepMind also decided to place the research outcomes — source code, structures of unknown proteins — easily accessible so more discoveries can happen. Already, this has assisted the Drugs for Neglected Diseases initiative (DNDi) in addressing deadly Chagas disease and Leishmaniasis. Since drug discovery has become faster due to AlphaFold, new drugs for rare diseases, which are of little commercial interest to pharma companies, have become possible.
Numerous other benefits
In 2020, a robotic synthesiser read a research paper and made the compound described in it. With giant advances in computational science and 3D protein structures, discovery labs will shrink to ‘AI synthesizers’. Thousands of molecules or processes may be screened for specific functions rapidly. Robots will characterize them to ‘discover’ an optimized strategy, directed by non-human ‘agents’. This could change chemistry.
The UNEP’s World Environment Situation Room (WESR) collects and analyses, using AI, real-time sensor data from thousands of sensors spread over 140 countries to predict carbon dioxide concentration, glacier mass, sea level rise, biodiversity loss, etc. Ultimately, we understand the health of the planet from a holistic perspective.
New risks
Large Language Models that built the likes of ChatGPT can create excellent text, music, and art. But they are not yet good at writing complicated chemical equations or new mathematical formulae to explain phenomena. When AI will eventually get there, when creativity is not exclusive to humans, the age of machines will appear.
For the scientific enterprise, in the era of ‘discoveries’ by ‘agents’ made of silicon, authorship may become meaningless. Those owning ‘agents’ may own knowledge.
Scientists warn that AI products must be used with caution. Tools such as ChatGPT can assist in literature search but cannot provide deep analysis and may miss profound insights central to articles. Intrinsic biases of scientific enterprise can under-represent minority views and could lose original thoughts, due to poor citations. Some journals have suggested authors to declare the use of AI tools in publications and have discouraged ChatGPT from being an author, with exceptions.
As compiling information and presenting them coherently by AI is easy, new paper factories may proliferate. Thankfully, such text can be identified by a new tool. AI-modified figures and images can produce a conundrum of ‘data’, making a nightmare for publishers. However, AI can be an excellent aid in helping authors in better visualisation, effective communication and compiling known facts, if used judiciously.
AI-divide
AI helps in the democratisation of knowledge. But ‘knowledge-to-things’ transformation will need infrastructure and resources. Advanced medicine and cutting-edge science are unlikely to develop in resource-limited settings. This is known historically, but there is a significant difference now. Infrastructure enabling advanced science is increasingly sophisticated and the gap between the haves and have-nots is widening dramatically. Clearly, proliferation of AI could concentrate wealth, breeding inequality.
The ‘AI being’ can write music, poems, and manuscripts faster, and possibly, even better. This could create polymath ‘beings’. It could radically transform workplaces and institutions. How would one evaluate productivity in the AI era? What could be the measure of excellence for individuals and institutions? The AI-divide will be far deeper than the digital-divide.
Act quickly
Governments at all levels must urgently assess the impact of AI on societies. They must form advisory groups and come up with AI and data-governance policy guidelines to direct institutions, industry, and society. Similar efforts must happen in each institution. An interdisciplinary environment is needed for responsible AI development. Surely, early movers will have a greater advantage.
(T. Pradeep is an Institute Professor at IIT Madras. pradeep@iitm.ac.in)