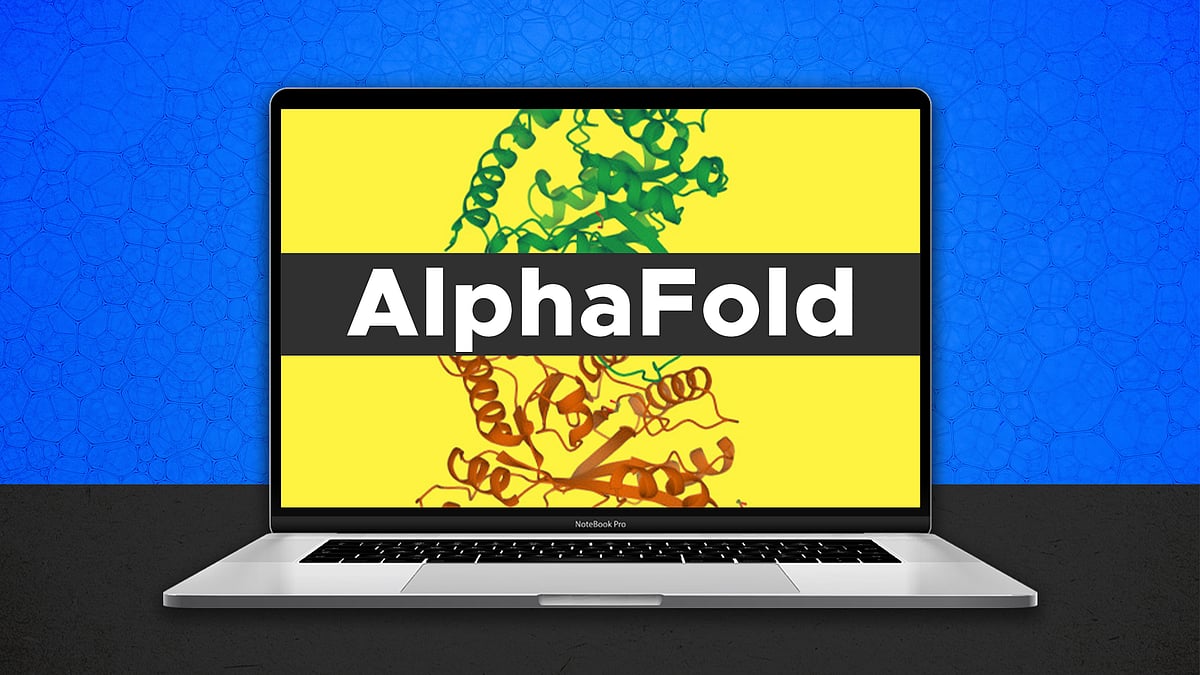
In November 2020, Alphabet’s subsidiary DeepMind got the scientific community excited with its progress in solving the 50-year-old ‘protein-folding problem’.
Across the world, DeepMind’s creation, AlphaFold, made headlines celebrating this breakthrough. The Guardian said DeepMind had cracked a problem that “stumped researchers for half a century”, Nature said DeepMind made a “gigantic leap in solving protein structures”, BBC said “one of biology’s biggest mysteries” had been “largely solved by AI”, and New York Times announced this could “accelerate drug discovery”.
Two years later, we have over 200 million protein structures predicted by AlphaFold. These predictions are finding applications routinely in academia and industries.
But what is the protein folding question and what did AlphaFold solve? What are the implications of AlphaFold?
This piece will attempt to bring you up to speed.
Proteins: Sequences and structures
Proteins are the workhorses of all forms of living beings. They are responsible not only for enabling nearly all reactions that sustain life, but also form several structural components of living beings. Almost every aspect of life – the digestion of your last meal, the synthesis of endorphins that make you happy, plants converting atmospheric carbon dioxide into sugar molecules, fluorescence of a jellyfish, replication of coronavirus within a human cell – is carried out by proteins.
Proteins are linear polymers composed of 20 building-block molecules called amino acids. Each protein has a particular sequence of amino acids. It is similar to a certain number of alphabets arranged in a particular sequence to form a word.
Like the number of letters in a word, the length of a protein can vary greatly from something as small as less than 100 to over 2,500 amino acids. Our understanding of how information encoded in the genes of an organism is translated to protein synthesis in cells, and the advances made in sequencing technologies for DNA, has provided us with an enormous number of protein sequences over the last few decades. The UniProt database (a free database of information about proteins from all organisms) has over 248 million protein sequences.
Like the sequence of letters in a word, the sequence of amino acids gives a protein its identity. But proteins are immeasurably more complex than words used in a language. Unlike linear words, they are three-dimensional. The string of amino acids in a protein polymer molecule arranges itself in a 3D space to adopt a shape that is essential for its role in biology. The 3D shape adopted by a protein, and thereby its function is determined by the sequence of amino acids.
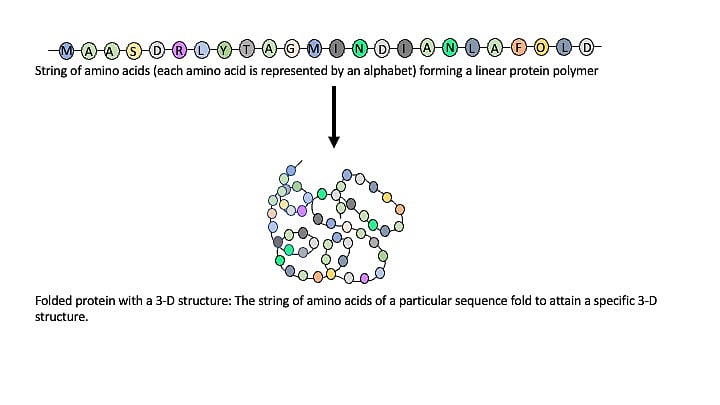
Protein structures that have been experimentally determined can be found in the Worldwide Protein Data Bank, a publicly available database. Figure 2 shows representations of the 3D structures of a few proteins.
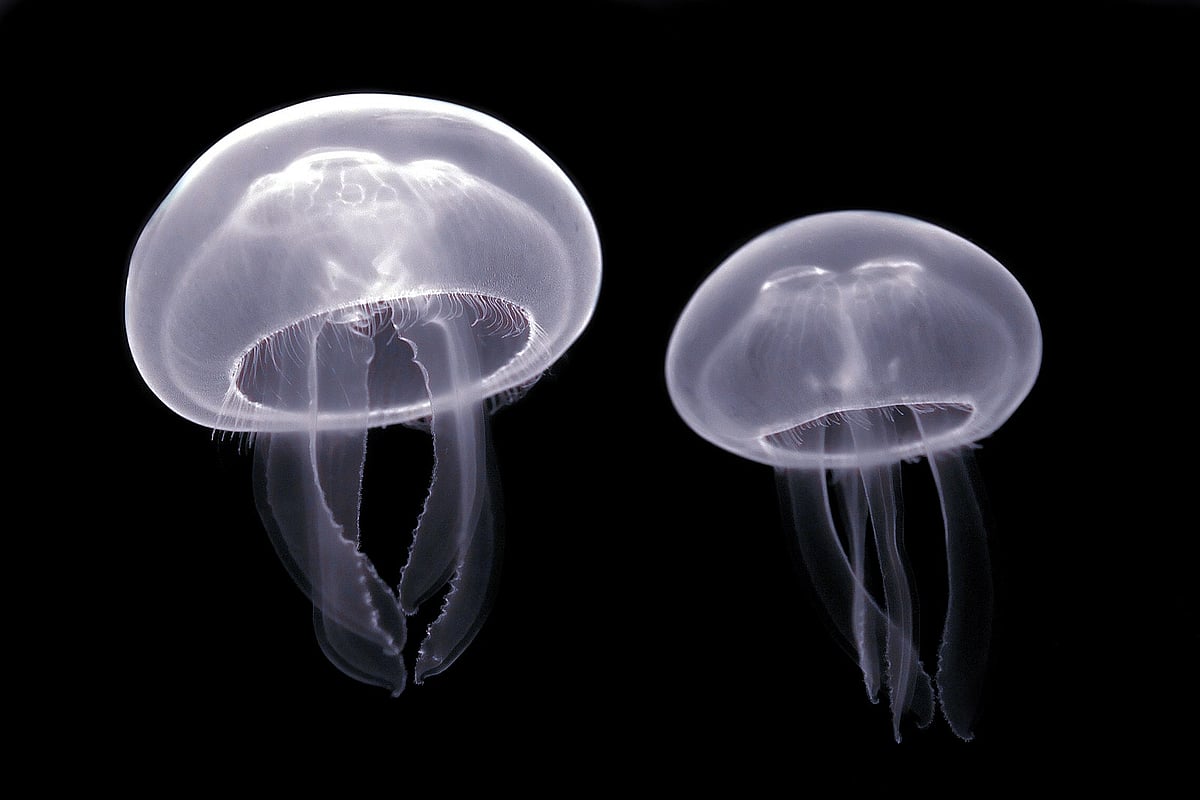
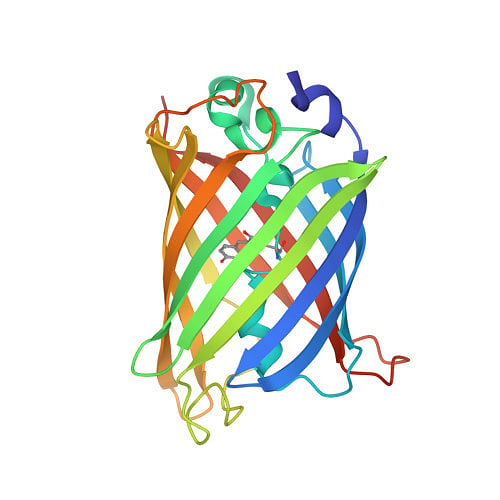
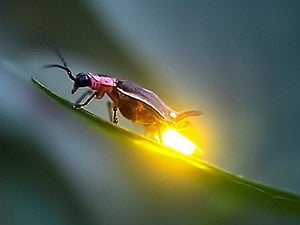
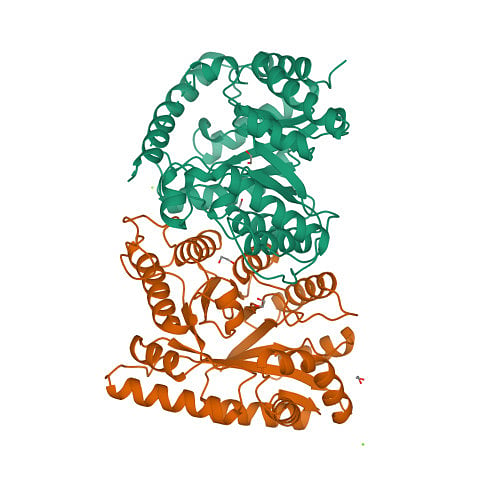
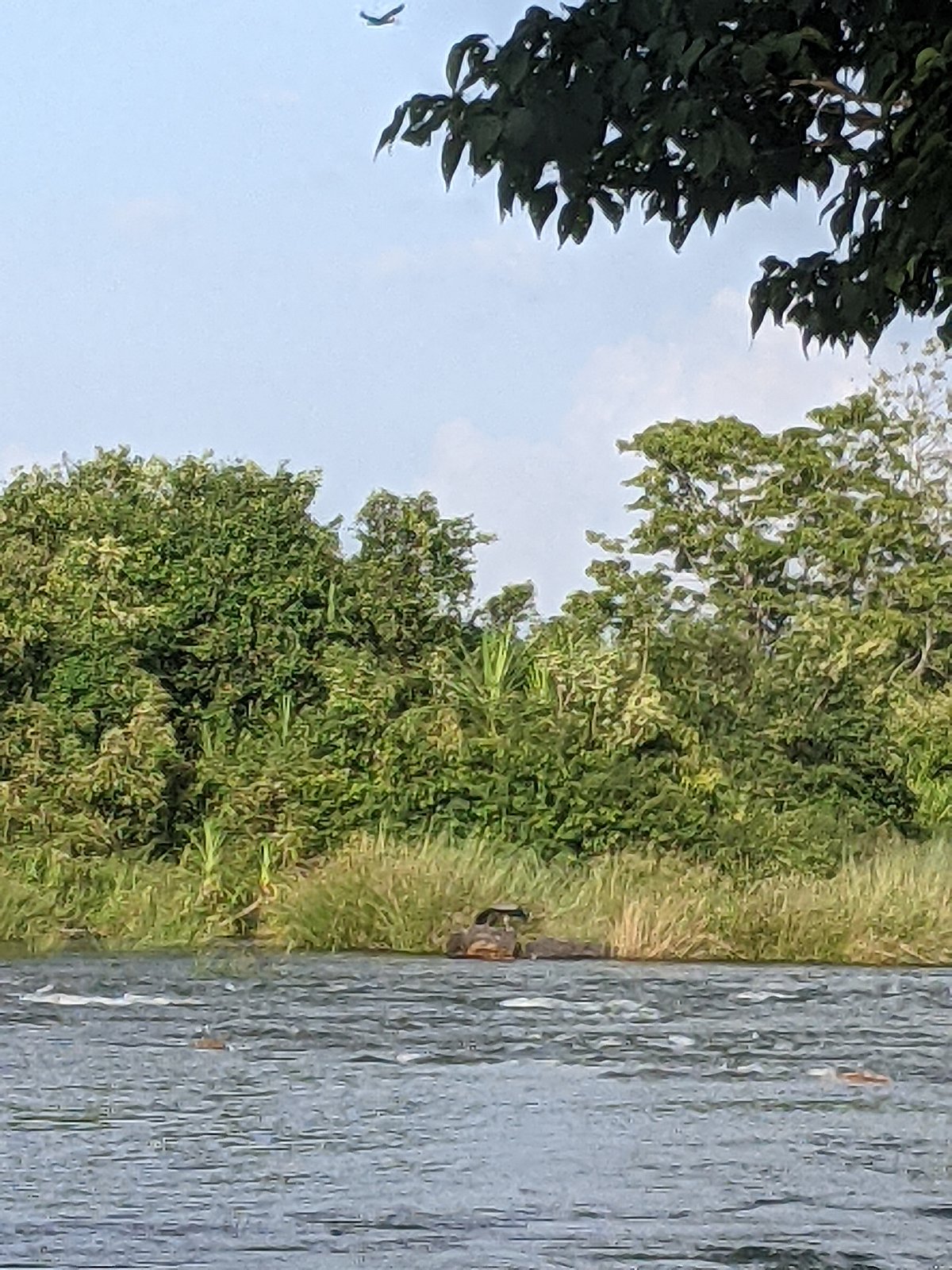
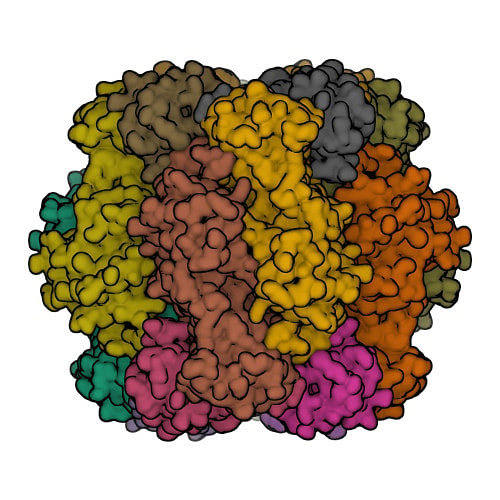
The scientific community has long recognised the importance of determining and understanding protein structures. Until now, 13 Nobel prizes have been awarded to breakthroughs in structural biology. But the number of proteins for which experimentally available structures exist (less than 225,000) is less than one percent of protein sequences.
Protein folding problem
An introduction to protein sequence and structure brings us to the ‘protein folding’ problem. Chiefly – what is it?
Take a relatively short protein sequence of 100 amino acids. The number of 3D shapes possible owing to rotation across chemical bonds is estimated to be 1094. This number – 1 followed by 94 zeroes – will increase exponentially with an increase in the length of the protein.
However, most protein molecules predominantly adopt a single stable shape that is essential for its biological activity. If this 100 amino acid-long protein molecule was to sample all possible shapes before choosing the most stable shape amenable to its function, and spent one microsecond on each shape, it would take more time than the age of the universe for all shapes to be sampled. This thought experiment is called the Levinthal paradox.
But all protein molecules know the predominant shape to adopt. Most do so in timeframes of milliseconds or less within a cell. The information to fold to the right shape is inherent in its sequence. This idea – that the sequence of a protein determines its 3D shape or structure – is referred to as Anfinsen’s dogma and was part of Christian B Anfinsen’s Nobel lecture in 1972.
Since the amino acid sequence determines the protein structure, it follows that one could predict the structure of a protein molecule if its sequence is known. This problem – of predicting the particular structure that a protein molecule with a specific sequence adopts – is called the protein folding problem. This question is five decades old and has bedevilled biologists.
The prediction of a protein structure from its sequence will be an incredible tool, since the experimental determination of protein structures is an intensive process that takes time, labour and resources. After the extraction and purification of the protein of interest, structure determination involves the usage of specialised techniques such as X-ray crystallography, cryo-electron microscopy (cryo-EM), and nuclear magnetic resonance. The procedure to determine the structure for a single protein can take anywhere between a few months to several years. It’s quite possible that these efforts, often that of an unlucky PhD student, are unsuccessful after several years of hard work.
Structure determination not only helps decipher the shape of a protein but also helps understand biological processes carried out by the protein. For example, the breaking down of food in the acidic environment of the stomach by the enzyme pepsin, or the interactions of surface proteins enabling the entry of a HIV virus into a human cell, or how the protein haemoglobin carries oxygen in blood. All these phenomena can be explained by studying high-resolution structures of the relevant proteins.
This knowledge finds applications such as designing drugs to fight diseases. The drugs designed to fight a microbial infection very often interact with a microbial protein inhibiting its function, which is crucial for the disease to manifest. Finding the right drug is like finding a Lego block that fits snugly in a cavity of the target microbial protein. This process of finding the drug or Lego block is easier when the structure of the target protein is known.
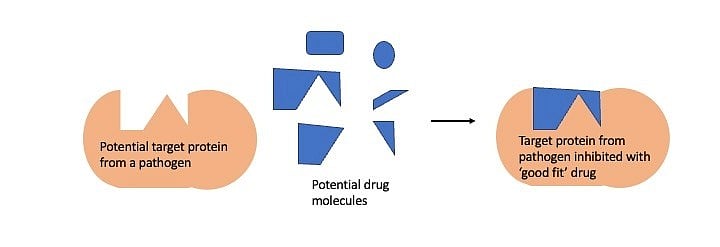
In the absence of structural information, this process of finding potential drugs is a laborious, expensive high-throughput screen involving tens of thousands of potential drug molecules – similar to looking for the right Lego piece in the dark. The availability of protein structure makes the search for the potential drug easier and more targeted.
Understanding the structure and function of a protein could also help us engineer novel proteins for optimising a useful function such as the breakdown of a toxic environmental pollutant, or the catalysis of synthesis of useful molecules.
A competition and a clear winner
Progress in addressing the pivotal question of protein folding in biology has been assessed through a biennial “world championship of algorithms” called the Critical Assessment of Protein Structure Prediction, or CASP.
Set up in 1994, CASP is a double-blind competition where teams from across the world are challenged to predict structures for several protein sequences. The protein sequences chosen by CASP’s organisers are for proteins that have unreleased experimentally determined structures. Around 100 teams submit predictions of structures from their algorithms. These predictions are assessed by CASP teams for their closeness to experimentally determined structures.
The results from the 13th and 14th editions of CASP in 2018 and 2020 saw DeepMind’s AlphaFold programme outperforming all other competitors.
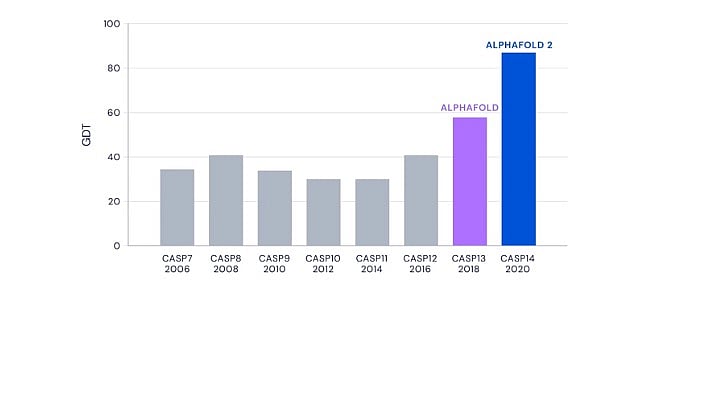
In CASP13, DeepMind’s performance was remarkable with a significant margin over the next competitor. But CASP14 saw the improved AlphaFold blow the competition out of the park. It not only made the best prediction for 88 out of 97 target sequences, but the accuracy of the predictions were unprecedented and unexpected.
CASP uses a metric called Global Distance Test, or GDT, to measure prediction accuracy. GDT ranges from zero to 100 and the value correlates with the percentage of amino acids whose positions were predicted correctly. A GDT score of 90 is informally considered to be comparable with experimentally determined structures.
AlphaFold achieved a whopping median GDT score of 92.4 at CASP14. While it was expected to have made progress since CASP13, AlphaFold’s performance in its second avatar less than two years later caught the entire scientific community by surprise.
Professor Venki Ramakrishnan, a Nobel Laureate, structural biologist, and president of the Royal Society, remarked, “This computational work represents a stunning advance on the protein folding problem, a 50-year-old grand challenge in biology. It has occurred decades before many people in the field would have predicted. It will be exciting to see the many ways in which it will fundamentally change biological research.”
Impacts of AlphaFold
The impacts of AlphaFold on research and development have already begun to unfold. The structures predicted are now routinely used in the methodology of X-ray crystallography for experimental protein structure determination. In 90 percent of cases, solving protein structures by X-ray crystallography requires a ‘seed model’ that might be difficult to obtain for certain protein sequences (This method for solving structures using X-ray crystallography is called ‘molecular replacement’). AlphaFold predictions are now used as “seed models” by researchers across the world when suitable models are not available.
AlphaFold’s impact was also seen in the determination of the structure of a large complex called the nuclear complex. The nuclear complex is a gateway between the nucleus of a cell, which holds genetic material, and the rest of the cell. This complex is made of over 30 proteins in human cells, and there is limited structural information from cryo-EM studies. The predictions from AlphaFold helped refine this cellular structure to an incredible level by supplementing the cryo-EM findings.
AlphaFold is already being used by the pharmaceutical industry in research to find new drugs. Also, several orphaned diseases and infections plaguing poorer regions of the world often receive little attention and funding. Researchers now have access to predicted structures that could help their initiatives to find drugs and cures for neglected diseases.
Needless to say, AlphaFold will find numerous uses in various aspects of fundamental and applied biology research.
Is the protein folding problem solved?
Has the 50-year-old problem found its solution? Will structural biologists with years of rigorous training become redundant?
In late 2020, computational biologist John Moult, who is the cofounder of CASP, declared, “In some sense, the problem is solved.”
“The low-hanging fruit has been picked,” said Mohammed Mohammed AlQuraishi, a computational biologist at Columbia University. “Some of the next problems are going to be harder.”
AlphaFold has indeed largely cracked the prediction of single protein structures from sequence. But biology is complex, and everything cannot be explained with static structures of single proteins. Most protein molecules, while adopting a stable structure for the most part, do respond to changes in their immediate environment (like temperature or viscosity) in the cell by changing their shape governed by the laws of physics and chemistry for atomic interactions. (It is worth noting that AlphaFold relies on existing structures and sequences, and is blind to the physics and chemistry of atomic interactions.) It must also be noted that there are protein molecules which adopt more than one 3D structure to carry out their roles. Proteins also interact with other biological molecules (such as DNA, RNA, lipids, other proteins and small molecules) to execute their functions.
There are also flexible proteins with disordered regions for which AlphaFold does not perform well. Understanding the dynamic nature and interactions of proteins still relies on experimental techniques. In fact, CASP15 in 2022 shifted its focus to the prediction of protein complexes (proteins interacting with other proteins or drug molecules). DeepMind has already released AlphaMultimer – which isn’t remotely as successful as AlphaFold – to predict structures of interacting proteins. It’s an area that several groups will be working on to achieve better results.
Also, the results from AlphaFold are predictions and not experimental determinations. They ought to be viewed in the context of confidence metrics of the predictions, and validations of predictions will be required for several downstream applications.
AlphaFold itself did not participate in the 2022 edition of CASP. Teams that performed well in it had incorporated methodologies used by AlphaFold.
The reliance of AlphaFold on databases also means that predictions with reasonable accuracies for sequences of proteins that are mutated (as seen in diseases or synthetic proteins) and for sequences containing amino acids other than the 20 commonly-occurring building blocks, or modified amino acids, may not be possible.
How does AlphaFold work?
Despite the limitations described above, AlphaFold is a phenomenal step in biology. It brings us to this question – how did AlphaFold manage this feat?
DeepMind utilises publicly available sequence data for over 200 million protein sequences and structural information for over 170,000 proteins and their immense expertise in machine learning to design AlphaFold. For a given target sequence, AlphaFold finds all sequences similar (not identical) to it in the database. The program then aligns them to generate multiple sequence alignment, or MSA. MSA helps identify stretches of sequence that mutate or change in a correlated fashion across the many sequences. This gives hints about amino acids that might be close in a 3D space. The target sequence is also used to identify existing structures with similar sequences.
The above information is combined to generate pairwise distances in 3D space between pairs of amino acids using neural network algorithms. This set of distances is then put through another set of neural network algorithms to generate the 3D structure.
In short, AlphaFold finds sequences similar to input. It extracts information using neural networks, and then passes this information to another neural network to obtain a 3D structure.
The overall idea appears simple, even obvious now, to academicians who have been using machine learning approaches to design protein structure prediction algorithms. But the execution of that idea was no simple feat. Demis Hassabis, the chief executive officer of DeepMind, said they came up with 30 new algorithms to make AlphaFold possible.
AlphaFold came to fruition with tremendous engineering expertise for machine learning techniques at DeepMind, several rounds of fine-tuning and optimisation of algorithms, discarding of approaches and trials that ran into roadblocks over several years, and access to vast amounts of computational resources. A 2021 research article on AlphaFold has 14 co-authors, hinting towards a massive collaborative effort within the team. AlphaFold’s development required 128 tensor processing units or TPUs (equivalent to 100-200 GPUs) of computational power. Resources of this nature are rare for academicians.
DeepMind and the creation of AlphaFold
DeepMind’s success at CASP was surprising, not only because of the magnitude of its accomplishment but also because it’s a tech firm, unlike traditional participants from academia.
So, how did a Google subsidiary enter the field of protein folding?
DeepMind was set up in 2010 in London to develop artificial intelligence technology. It was acquired by Google in 2014. Two of its three founders (Demis Hassabis and Shane Legg) have backgrounds in computer science and neurobiology.
The company began by developing algorithms to play games such as Go, chess and Shogi. Among the several programs it developed are AlphaGo (which plays the game Go) and Alpha Zero (which plays any two-player game), both with abilities that far exceed those of the best players in the world. The development of AI algorithms to play games, and the learnings from the process, equipped DeepMind with the expertise to address several questions.
It’s this expertise and the quest to address complicated questions across disciplines that perhaps brought DeepMind to protein folding – a well-defined problem in biology with clear objectives and metrics that could be addressed with AI.
When AlphaFold made its mark at CASP, its implications were apparent to the scientific community. Questions remained on whether the specifics of the algorithm would ever be made public, or if the company would monetise its findings. Following CASP14 in 2020, DeepMind published the details of its methodology in June 2021 in the journal Nature and made the code publicly available on Github.
It must be noted that running AlphaFold still requires considerable computational resources – at least 3 TB of space and a modern NVIDIA GPU. In July 2022, DeepMind made it easier for us to cross this hurdle by making predictions available for all known protein sequences (over 200 million) in collaboration with the European Molecular Biology Laboratory’s European Bioinformatics Institute. Any individual can access AlphaFold’s predicted structures on the protein database UniProt. In an interview, Hassabis said over 500,000 researchers have used AlphaFold within a year of its release.
Final thoughts
It does make one wonder – why would a private tech firm undertake such a project with altruistic motives?
Breakthroughs in science are often a culmination of cumulative efforts by several groups of individuals working in different disciplines over a very long period of time. AlphaFold was developed following decades of work by thousands of researchers that was made freely available to everyone via the protein database. So, the argument follows that DeepMind makes AlphaFold public.
The most significant gain for DeepMind is perhaps the development of expertise and technology, and the validation of its learnings from previous AI ventures. The increment in its abilities owing to AlphaFold development is potentially invaluable. Also, the open sourcing of all predictions will help validate AlphaFold by experimentalists and identify potential areas to improve the algorithm.
Whatever the reasons, it’s commendable that DeepMind decided to make all the information open-source, benefiting a large number of academic researchers and life science related industries.
AlphaFold will have a massive impact in the years to come for biologists, and it has opened more doors to address questions in biology at cellular, organismal and ecological levels with AI. The development of AlphaFold represents the power of interdisciplinary research and collaborations. This highlights the need for opportunities in our educational institutions for youngsters to explore multiple fields, and not be pigeon-holed into their specialisations. An appreciation and curiosity for areas of work beyond one’s realm of expertise, and the ability to visualise the bigger picture by those who have the resources and power in academic institutions and industries, will lead to exciting research and solutions for complex problems.
Newslaundry is a reader-supported, ad-free, independent news outlet based out of New Delhi. Support their journalism, here.