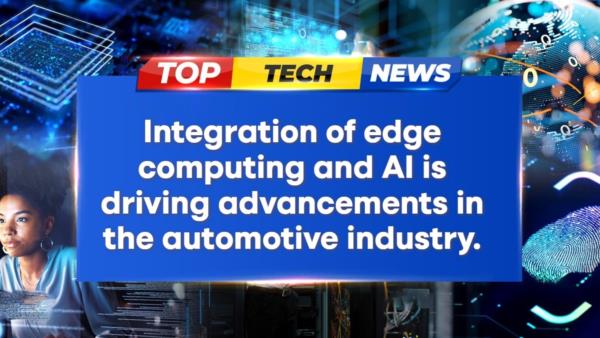
The automotive industry has undergone significant advancements in recent years due to the integration of edge computing and artificial intelligence (AI). These technologies have become increasingly critical as vehicles continue to develop self-driving capabilities, enabling effective decision-making and real-time reactions on the road.
Edge computing plays a crucial role in processing data and commands locally within a vehicle's systems. This not only improves road safety but also enhances transportation efficiency. When combined with 5G, edge computing enables real-time communication between vehicles and infrastructure, reducing latency and allowing autonomous vehicles to respond faster. AI algorithms further enhance the capabilities of vehicles by enabling them to interpret visual data and make human-like driving decisions.
The integration of edge computing and AI is transforming vehicles into true self-driving machines, bridging any gaps in low-latency 5G technology and enabling companies to pioneer advanced autonomy. Today, autonomous vehicles are experiencing a shift in computing from centralized servers to decentralized edge devices. This evolution in edge computing has significantly reduced latency, enabling vehicles to interpret sensor data and react to changing road conditions in real time.
By leveraging decentralized nodes and real-time analytics, autonomous vehicles can process visual data, detect obstacles, adjust to unexpected events, and make critical driving decisions without any delay. This progression paves the way for fully autonomous cars that can navigate roads independently.
One notable player in the autonomous driving field is Tesla. The company's thorough collection of real-world driving data provides a unique advantage in refining their AI algorithms for autonomous driving. As more drivers gain access to Tesla's Full Self-Driving (FSD) beta software, the impressive results of Tesla's algorithms in real-world driving conditions become increasingly evident. The vast amount of visual data collected during these drives aids in the learning process of Tesla's AI, improving its reliability and effectiveness in real-world situations.
Edge computing and AI applications are not limited to the automotive industry alone. They are revolutionizing the maintenance and reliability sectors across various industries. Intelligent sensors and devices can now collect real-time data on temperature, vibration, stress levels, and other metrics at the edge, enabling highly effective predictive maintenance workflows.
Rather than relying on scheduled maintenance or standard inspections, edge technologies allow for intelligent monitoring of equipment conditions. Potential problems and failures can be predicted before they occur based on data patterns and analytics, preventing costly unplanned downtime and enhancing proactive maintenance capabilities.
Bringing condition monitoring and predictive analytics to the edge provides instantaneous insights compared to centralized cloud computing. Industries across the board are rapidly adopting these intelligent systems to achieve new levels of efficiency and reliability. It is expected that with the increasing number of devices operating at the edge and sharing real-time data insights, the technology landscape will continue to evolve.
While there are still challenges to overcome in the realm of autonomous vehicles, the future holds great promise. It is estimated that autonomous driving could generate between $300 billion and $400 billion in the passenger car market by 2035. Additionally, it is predicted that there will be over 33 million driverless vehicles on the road by 2040.
Automakers are taking different approaches to developing autonomous vehicle technology. Tesla, for example, is focused on vision-based systems powered by AI, while General Motors and its subsidiary Cruise emphasize lidar sensors in combination with cameras and radar. Ford and Volkswagen are collaborating on self-driving cars that combine lidar, radar, and cameras with detailed road maps. Waymo, Google's self-driving unit, has been testing autonomous minivans equipped with a diverse sensor suite comprising vision, lidar, and radar hardware.
In addition to sensor technology, computing power plays a crucial role in autonomous driving. Automakers like Mercedes and Volvo are partnering with chipmaker Nvidia to utilize their powerful Drive platform, which is specifically designed for self-driving cars.
The automotive industry is vigorously pursuing self-driving technologies through various innovations. While companies continue to innovate, the dream of fully driverless cars is inching closer to reality.
The rapid advancements in artificial intelligence, machine learning, and robotics have far-reaching implications beyond just the automotive industry. As autonomous systems powered by AI encounter more complex real-world variables, the applications of these technologies will transform various sectors.
AI and robotics are increasingly integrating into manufacturing processes, replacing or augmenting human workers to optimize efficiency and output. Intelligent robotic systems can operate tirelessly on assembly lines with precision and consistency, leading to higher productivity and economic growth.
The capabilities of AI and machine learning will also enable transformative changes in sectors like healthcare, agriculture, financial services, and more. AI diagnostic tools can analyze medical scans and data to support doctors in making quick and accurate diagnoses. Farming equipment and robots can automate planting, watering, and harvesting using computer vision, GPS, and AI, reducing the need for human intervention. Fintech applications of AI include algorithmic trading, personalized banking through chatbots, and detecting fraudulent activities by analyzing patterns.
As edge computing brings increased processing power to devices, combined with rapid improvements in AI, the potential applications are endless. According to Gartner, by 2025, 75% of all enterprise data will be processed at the edge, a significant increase from just 10% in 2018. This highlights the immense potential for edge computing to bring about unprecedented changes across industries.
The future of autonomous vehicles holds tremendous promise, although there are still challenges to address. Significant strides have been made with the FSD beta, showcasing functionalities such as automatic lane changes, navigating complex intersections, and detecting traffic lights and stop signs. However, achieving full vehicle autonomy in all conditions remains an ongoing endeavor.
Industry experts have varying predictions for when fully autonomous cars will become commonplace. However, most agree that we will observe a gradual progression, with autonomous capabilities increasing over time. This includes the implementation of more comprehensive driver assistance features, continued phased testing of self-driving systems, and expansion of regulatory approvals.
Through ongoing research, refined software, accumulated driving data, and rigorous real-world validation, the promise of safe autonomous transportation for all could become a reality.
As these technologies continue to advance, we can anticipate even more seamless integration of intelligent edge devices and AI assistants into our lives. With diligent testing and responsible deployment, autonomous technologies supported by edge computing have the potential to profoundly augment human capabilities and transcend current limitations.
The rapid advancements in artificial intelligence, machine learning, and robotics have made their mark on various sectors, including the automotive industry. As autonomous systems encounter more complex real-world variables, the potential applications of edge computing and AI are expanding, transforming industries and revolutionizing the way we live, work, and interact with technology.