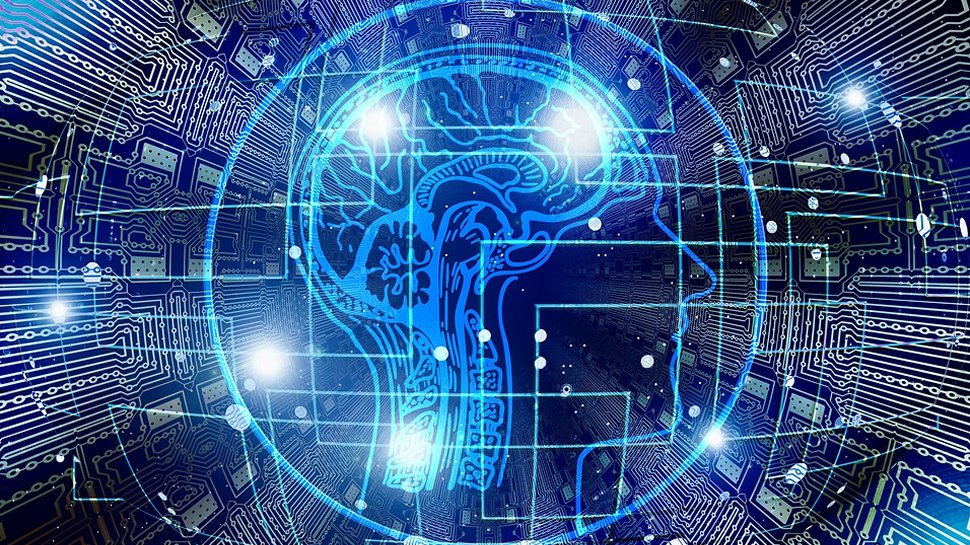
Artificial intelligence will contribute $19.9 trillion to the global economy through 2030 and drive 3.5% of global GDP in 2030, according to new research from IDC’s The Global Impact of Artificial Intelligence on the Economy and Jobs. The report goes on to claim that there is “accelerated development and deployment defined by widespread integration that's led to a surge in enterprise investments aimed at significantly optimizing operational costs and timelines.” Clearly AI is no longer a future, aspirational technology but today an increasingly essential driver of business transformation.
Yet, the rapid pace of AI development means that creating an effective AI strategy can be complex. It demands flexibility, resilience, and a future-ready infrastructure but given so much legacy technology and differing attitudes to what is the best path to take, this is not an easy thing to put into place. While most leaders recognize that the potential is significant, the path to success lies in building an AI strategy that not only aligns with business objectives but also adapts to evolving technology capabilities.
AI models, particularly large language models (LLMs), require significant resources and infrastructure to perform at their best. Organizations, therefore, need a strategy that allows them to quickly integrate new AI models, without causing disruption or push up costs. The solution? A flexible approach that incorporates cloud integration, containerization, and automation.
Start as you mean to go on
The starting point of any AI journey must be identifying a core business problem that AI can help solve. AI is a powerful tool, but without a clear application, it can easily become a costly distraction. Focus on business areas where AI can drive value, whether that’s optimizing customer service, improving fraud detection, or predicting maintenance needs. Ensuring AI is tied to measurable outcomes is the foundation of an effective strategy and makes it easier to sell internally too.
Furthermore, the flexibility to adopt a "bring your own large language model" (BYOL) approach enables organizations to customize AI to their needs. This method, which integrates public models like those from Nvidia or Hugging Face, allows businesses to refine these models with private data, ensuring solutions are aligned with specific business challenges. It’s a powerful way to use cutting-edge technology, while keeping control of sensitive data.
Any AI strategy worth its salt must be built on a flexible cloud infrastructure. The demands of AI are not static; they evolve rapidly as more complex models and data sets come into play. A cloud-first approach allows organizations to manage these changes without costly, time-consuming hardware upgrades.
Hybrid and multicloud environments offer even greater flexibility, giving businesses the power to move workloads between on-premises and public clouds depending on their specific needs. This flexibility is key to managing the dynamic nature of AI development, where rapid iteration and model refinement are essential. Cloud integration also enables easier scalability, allowing enterprises to handle increased data volumes and computational demands, as their AI projects grow.
However, AI’s dependence on data raises important questions around security and governance. As businesses scale their AI initiatives, they will be handling vast amounts of data, much of it sensitive. This is particularly true in sectors such as finance, healthcare, and government, where data privacy is paramount. Organizations must ensure their AI strategy includes robust data governance protocols that protect both the data they’re using and the outputs their AI models produce.
Cloud-based environments offer built-in security features that can help protect data across various platforms. However, understanding your own data and applying AI models to it effectively is a key challenge. Organizations must ask the right questions - where is my data stored? How is it secured? How is it used in training AI models?
Automation can unlock AI’s full potential
Automation plays a crucial role in the successful deployment of AI. Managing AI workloads across multicloud environments can be time-consuming and resource-intensive if done manually. By automating tasks, such as resource allocation and scaling, businesses can deploy AI models faster and more efficiently. This also reduces operational costs, allowing IT teams to focus on more strategic objectives.
AI applications benefit greatly from using containers - small, lightweight environments that package AI models and their dependencies. These containers allow AI systems to be deployed quickly and moved seamlessly between different environments. By using Kubernetes to manage these containers, businesses can achieve the agility needed to stay competitive in an AI-driven world. Kubernetes, in particular, enables organizations to orchestrate complex AI workloads across cloud platforms, ensuring optimal performance.
This becomes even more important when we consider skills shortages. One of the most significant challenges in AI adoption is the lack of specialized talent, both in AI development and cloud management. The fast-paced nature of AI requires teams that can quickly adapt to new tools and techniques. However, the reality is that many organizations lack the in-house expertise to meet this demand.
Automation provides a solution to this challenge by reducing the complexity of AI deployment. Organizations can rely on automated systems to handle many of the operational tasks traditionally managed by highly specialized teams, freeing-up resources, to focus on optimizing AI models and drive business value.
With the rapid evolution of AI technologies, ensuring responsible implementation is crucial. Organizations must focus on compliance, governance, and ethical considerations when deploying AI. Responsible AI is about more than just technology, it’s about ensuring that the outputs generated by AI models are transparent, fair, and free from bias.
This is particularly important as businesses integrate AI into more sensitive areas, such as customer support, fraud detection, or decision-making processes. By building compliance into AI infrastructure from the outset, organizations can avoid potential pitfalls and ensure their AI deployments are both effective and responsible.
The key to future success is building a strategy that can evolve with technology. Flexible cloud infrastructure, automation, and containerization are critical components of this strategy, enabling businesses to adapt quickly to new advancements in AI but it is also about culture. Getting a strategy right is about people as much as the technology. For those who are prepared to embrace it with agility, responsibility, and strategic foresight, the future is undeniably bright.
We've featured the best AI phone.
This article was produced as part of TechRadarPro's Expert Insights channel where we feature the best and brightest minds in the technology industry today. The views expressed here are those of the author and are not necessarily those of TechRadarPro or Future plc. If you are interested in contributing find out more here: https://www.techradar.com/news/submit-your-story-to-techradar-pro