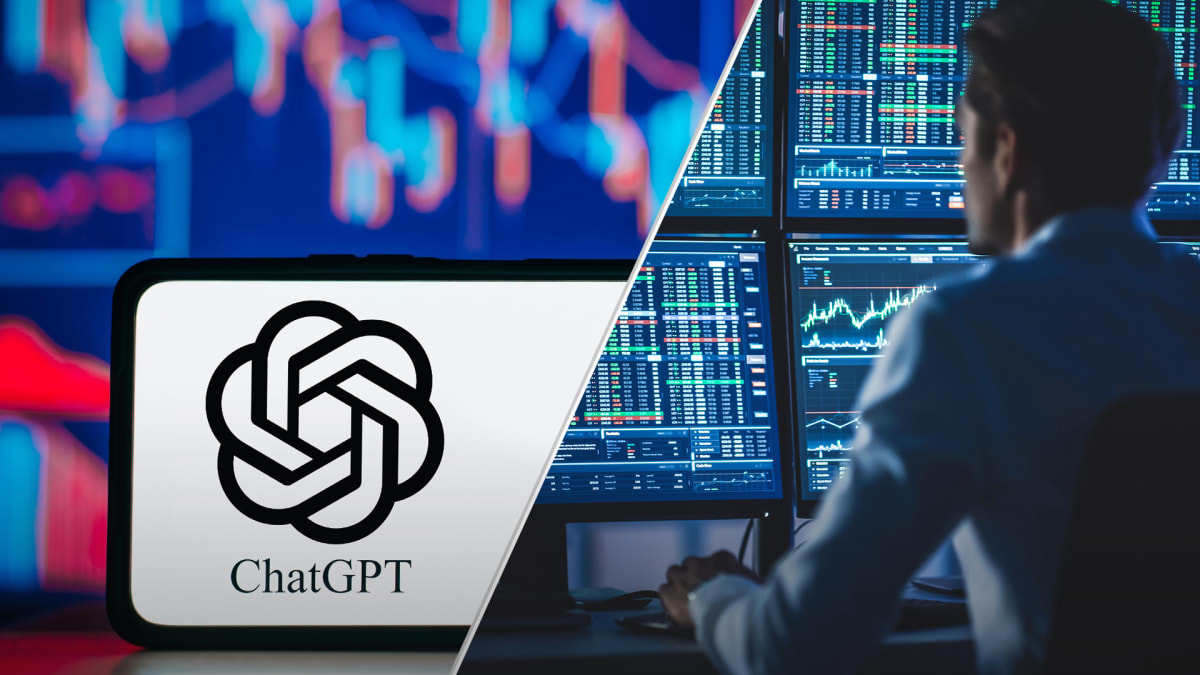
OpenAI CEO Sam Altman has been saying for months that the benefits that artificial intelligence could lead to far outweigh the risks.
When he refers to risks, he's discussing the potential of an out-of-control AI model that could wreak havoc on civilization.
"If this technology goes wrong, it could go quite wrong," he said at a May Senate hearing on AI oversight.
DON'T MISS: Artificial Intelligence Isn't Going to Kill Everyone (At Least Not Right Away)
Whenever Altman discusses those benefits, he keeps mentioning two things: solving climate change and curing cancer.
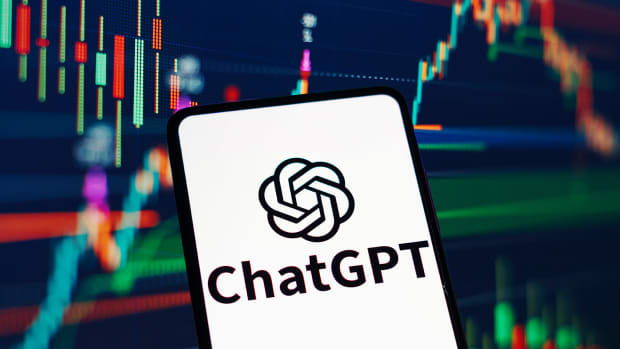
LLMs 'don't really have a positive climate side'
"We are working to build tools that one day could address some of humanity's biggest challenges," he said in his opening remarks at the hearing, "like climate change and curing cancer."
But the reality is that these large language models (LLMs) are not the right solution. They're actually part of the problem.
"There are a lot of climate-positive applications of AI," Dr. Sasha Luccioni, a leading researcher in ethical AI told The Street. These applications could include analyzing satellite data to detect forest fires, for example. But the kinds of models that can have these climate-positive impacts are "small neural networks. Their carbon footprint is relatively small, but they have great potential."
LLMs -- like ChatGPT -- "need a lot of computing resources, but they don't really have a positive climate side," Luccioni said.
"That's the dichotomy," she said. "We could be doing great stuff for the climate with AI, which we are doing to some extent, but it's kind of being voided by these large language models and the amount of resources they need."
Environmental Impact of AI
Estimating the carbon footprint of popular LLMs, like ChatGPT or Bard, is a near impossibility because of a critical lack of transparency.
"The thing is, with large language models, the majority are closed source and so you don't really get access to their nuts and bolts," Luccioni said. "And so it's hard to do any kind of meaningful studies on them because you don't know where they're running, how big they are. You don't know much about them."
The idea, however, goes back to the base concept that anything that is powered by a dirty electrical grid will result in carbon emissions. Things that require enormous computing power, like ChatGPT, will result in more emissions.
One Danish data scientist, Kasper Groes Albin Ludvigsen, took a stab at estimating the electrical output of ChatGPT back in January when the model ran on GPT-3, which has 175 billion parameters.
His calculations, based on a comparable LLM built by HuggingFace called Bloom, estimate that, in January, ChatGPT used between 1.2 million and 23.4 million kWh.
The average U.S. household, in comparison, consumed 886 kWh per month in 2021.
But even with that rough electrical estimation, trying to figure out the carbon footprint is even more difficult. Each grid is different, and the number of data centers used to run ChatGPT, as well as their locations, could have a big impact on the actual carbon footprint of these models.
"We did a study on Bloom, which is the first open-source language model," Luccioni said, adding that the carbon emissions from a specific model would "really vary depending on where you deploy the model, how many requests you're getting, how many GPUs you need, et cetera, et cetera."
Over an 18-day period, Bloom consumed 914 kWh of electricity and had a carbon footprint of 340 kg. During this time period, the model handled 230,768 total requests.
ChatGPT in January hit 100 million active monthly users, with a total of nearly 600 million visits.
More Artificial Intelligence:
- Why ChatGPT Can't Turn Into Marvel Villain Ultron (Yet)
- Human Extinction From AI is Possible, Developers Warn
- Protesters Say OpenAI CEO Is Dangerously Misled When It Comes to AGI
Beyond electricity consumption, the data centers that power these models use an enormous -- also unknown -- amount of water for cooling purposes. A recent study found that "training GPT-3 in Microsoft’s state-of-the-art US data centers can directly consume 700,000 liters of clean freshwater. The water consumption would have been tripled if training were done in Microsoft’s Asian data centers, but such information has been kept as a secret."
And that's just for the training.
The average US household uses about 300 gallons of water per day, according to the EPA, or roughly 1,135 liters.
ChatGPT is a 'One Size Fits All Solution'
For Luccioni, the broader problem -- beyond a lack of transparency -- is this growing tendency to use bigger, less efficient models like ChatGPT where it doesn't really make sense.
"I think that we're currently seeing a trend of a one size fits all solution," she said. "Everyone's trying to plug in LLMs and see what sticks and so I think we're going to see more energy usage, more compute, just because now everything has to have an LLM behind it, just because it's trendy."
OpenAI did not respond to requests for comment.
Luccioni said there used to be "all sorts of models for all sorts of tests, which makes sense. Nowadays, you have this 'this is going to solve everything' situation. And I think that's something worth rethinking because the models keep getting bigger."
"You can't make them go faster," Luccioni said. "Of course, you can always upgrade GPUs or use more of them, and that's what people typically have been doing. But sustainability-wise, it doesn't make sense."