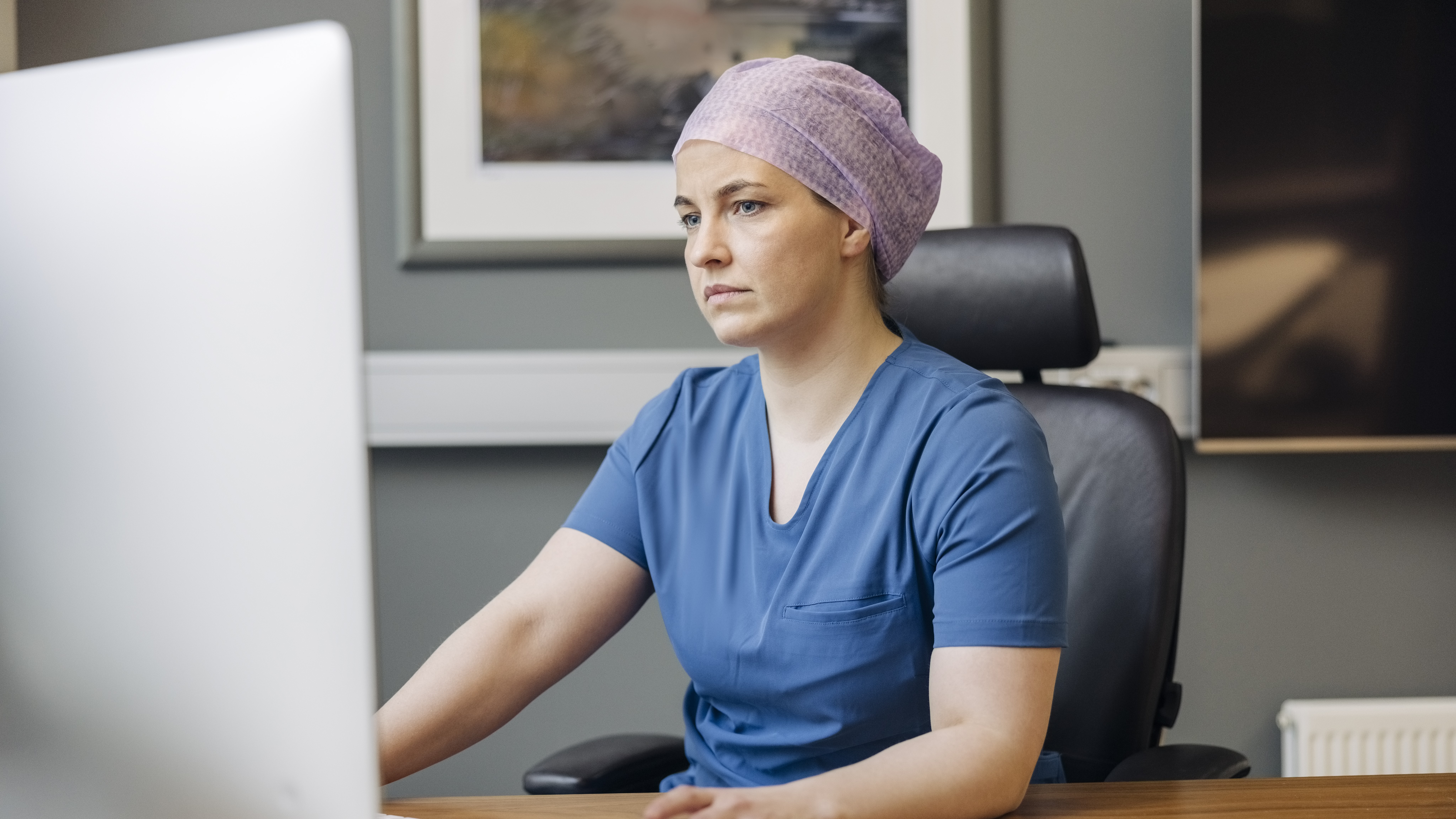
Artificial intelligence (AI) has advanced, but it's still far from perfect. AI systems can make biased decisions, due to the data they're trained on or the way they're designed, and a new study suggests that clinicians using AI to help diagnose patients might not be able to spot signs of such bias.
The research, published Tuesday (Dec. 19) in the JAMA, tested a specific AI system designed to help doctors reach a diagnosis. They found that it did indeed help clinicians more accurately diagnose patients, and if the AI "explained" how it made its decision, their accuracy increased even more.
But when the researchers tested an AI that was programmed to be intentionally biased toward giving specific diagnoses to patients with certain attributes, its use decreased the clinicians' accuracy. The researchers found that, even when the AI gave explanations that showed its results were obviously biased and filled with irrelevant information, this did little to offset the decrease in accuracy.
Although the bias in the study's AI was designed to be obvious, the research points to how hard it might be for clinicians to spot more-subtle bias in an AI they encounter outside of a research context.
"The paper just highlights how important it is to do our due diligence, in ensuring these models don't have any of these biases," Dr. Michael Sjoding, an associate professor of internal medicine at the University of Michigan and the senior author of the study, told Live Science.
Related: AI is transforming every aspect of science. Here's how.
For the study, the researchers created an online survey that presented doctors, nurse practitioners and physician assistants with realistic descriptions of patients that had been hospitalized with acute respiratory failure — a condition in which the lungs can't get enough oxygen into the blood. The descriptions included each patient's symptoms, the results of a physical exam, laboratory test results and a chest X-ray. Each patient either had pneumonia, heart failure, chronic obstructive pulmonary disease, several of these conditions or none of them.
During the survey, each clinician diagnosed two patients without the help of AI, six patients with AI and one with the help of a hypothetical colleague who always suggested the correct diagnosis and treatment.
Three of the AI's predictions were designed to be intentionally biased — for instance, one introduced an age-based bias, making it disproportionately more likely that a patient would be diagnosed with pneumonia if they were over age 80. Another would predict that patients with obesity had a falsely high likelihood of heart failure compared to patients of lower weights.
The AI ranked each potential diagnosis with a number from zero to 100, with 100 being the most certain. If a score was 50 or higher, the AI provided explanations of how it reached the score: Specifically, it generated "heatmaps" showing which areas of the chest X-ray the AI considered most important in making its decision.
The study analyzed responses by 457 clinicians who diagnosed at least one fictional patient; 418 diagnosed all nine. Without an AI helper, the clinicians' diagnoses were accurate about 73% of the time. With the standard, unbiased AI, this percentage jumped to 75.9%. Those given an explanation fared even better, reaching an accuracy of 77.5%.
However, the biased AI decreased clinicians' accuracy to 61.7% if no explanation was given. It was only slightly higher when biased explanations were given; these often highlighted irrelevant parts of the patient's chest X-ray.
The biased AI also impacted whether clinicians selected the correct treatments. With or without explanations, clinicians prescribed the correct treatment only 55.1% of the time when shown predictions generated by the biased algorithm. Their accuracy without AI was 70.3%.
The study "highlights that physicians should not over-rely on AI," said Ricky Leung, an associate professor who studies AI and health at the University at Albany's School of Public Health and was not involved in the study. "The physician needs to understand how the AI models being deployed were built, whether potential bias is present, etc.," Leung told Live Science in an email.
The study is limited in that it used model patients described in an online survey, which is very different from a real clinical situation with live patients. It also didn't include any radiologists, who are more used to interpreting chest X-rays but wouldn't be the ones making clinical decisions in a real hospital.
Any AI tool used for diagnosis should be developed specifically for diagnosis and clinically tested, with particular attention paid to limiting bias, Sjoding said. But the study shows it might be equally important to train clinicians how to properly use AI in diagnoses and to recognize signs of bias.
"There's still optimism that [if clinicians] get more specific training on use of AI models, they can use them more effectively," Sjoding said.
Ever wonder why some people build muscle more easily than others or why freckles come out in the sun? Send us your questions about how the human body works to community@livescience.com with the subject line "Health Desk Q," and you may see your question answered on the website!