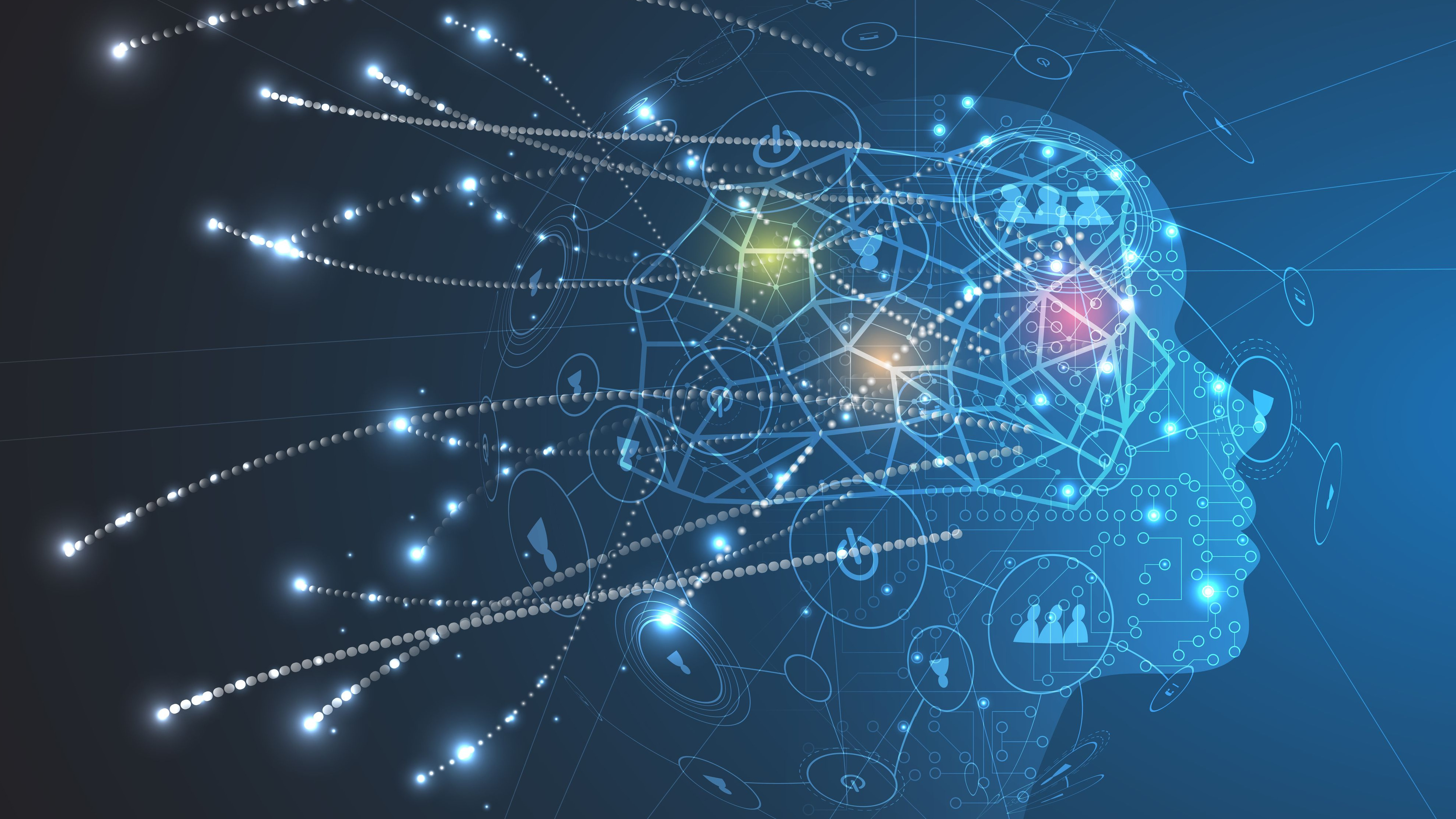
With the capabilities of artificial intelligence (AI) evolving at such a startling pace, one of the most pressing challenges faced by data teams and engineers is how to handle the mass of unstructured and heterogenous data sources.
Unlike structured data, that is able to fit neatly into tables and databases, unstructured data is built up from a vast array of formats, including video, text and images. These formats all have their own intricacies, and the heterogeneity of these data sources can add further levels of complexity.
With this in mind, can teams find a way to optimize the collection and analysis of their data to maximize the impact of AI on their business? Given how activity is trending, agent-based systems and agent-agent communication appear to be the golden idea that will take the AI movement to the next level.
Unstructured data’s historical challenge
Historically, unstructured data, like audio, video, and social media interactions, has posed a substantial challenge for companies trying to interpret and convert it into formats that are structured appropriately for analysis and AI applications. For many organizations, the sheer complexity and cost of processing this unstructured data has meant that until recently, it remained heavily underutilized.
As a result, despite unstructured data comprising the majority of available data and possessing significant unrealized potential, organizations have tended to turn towards structured data, such as excel files and Search Engine Optimization (SEO) tags.
In recent years however, technological developments in the use of AI, along with generative AI, have transformed the way in which unstructured data can be interpreted and extracted.
For example, major cloud companies, including Microsoft and Google, have expanded their cloud services to support creating “data lakes” from unstructured data. Microsoft’s Azure AI now uses a mixture of text analysis, optical character recognition, voice recognition and machine vision to interpret an unstructured data set that could include text or images. Thanks to this advancement, businesses can now access this richer resource of data and finally unlock its value.
What are the current issues with unstructured data?
Organizations can now tap into a wealth of rich information that was previously inaccessible.
However, this is still not without its challenges. For example, navigating the varying levels of content quality, scope and detail of this unstructured data can pose a significant hurdle. With unstructured data, there tends to be much more irrelevant noise. If there is too much of this, then it can be challenging for even AI to accurately identify answers while sifting through information.
Additionally, the lack of regulation when it comes to the creation of unstructured data can impact its usefulness. Whilst these larger datasets generally offer greater levels of consistency, it is still a challenge adapting them to be utilized by AI and thus leveraged more effectively by organizations.
Being able to effectively utilize unstructured data typically involves incorporating it into an organization's existing data framework. A comprehensive understanding of the data’s properties, connections, and possible uses is necessary for this integration. A big challenge for many of these unstructured projects is to simply define a clear goal, in order for these models to be trained accurately.
Many organizations still struggle to leverage these existing data assets to generate business value.
So, whilst the previous issue of unlocking and obtaining the data has been largely solved, being able to hypothesize its potential value and applications remains a significant obstacle.
What is expected next for the GenAI movement?
In the future, we should expect human involvement in data sourcing and interpreting to decrease. We are instead likely to see an increase in agent-based systems, along with agent-to-agent communications, which minimizes the need for human intervention in data handling. The boom in generative AI has paved the way for specialized agents, which include:
- “Engineering agents” for code generation
- “Data generation agents” for creating synthetic data for testing
- “Code testing agents” for validating and testing code
- “Documentation agents” for generating documentation for various aspects such as code, use cases, and processes
There is no question that a system where specialized AI agents interact with one another can accelerate development, make it more accurate, and more consistent.
Organizations can now devote greater resources to utilizing data rather than preparing it. It is highly likely that in the near future, we will see these AI agents be offered as a product by service providers. These service providers could take a company’s requirements, then produce a fully tested, spec-compliant code produced by AI agents.
By outsourcing these technical tasks, companies would significantly reduce the length of time taken to complete these kinds of tasks, as well as reducing the need for large in-house development teams. It appears the time is now for companies to consider those specific roles generative AI can play to maximize value from their data programs, and ultimately get far greater results from their investment in these recently expanded areas.
It has been known for some time that generative AI has the potential to revolutionize the way organizations operate. However, implementing it effectively into organizations will still mean navigating its weaknesses before maximum capabilities can be achieved.
Organizations are yet to fully embrace AI-friendly data acquisition and integration. Those that adapt can maximize investment value and change their fortunes for the better.
We've featured the best AI chatbot for business.
This article was produced as part of TechRadarPro's Expert Insights channel where we feature the best and brightest minds in the technology industry today. The views expressed here are those of the author and are not necessarily those of TechRadarPro or Future plc. If you are interested in contributing find out more here: https://www.techradar.com/news/submit-your-story-to-techradar-pro