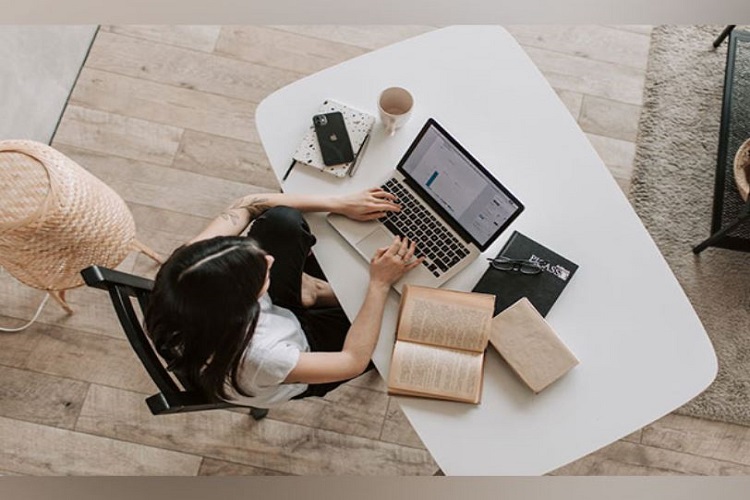
Washington: Researchers found a new way to teach a quantum computer how to understand and predict the behaviour of quantum systems, even with a few simple examples.
The study was published in the journal, 'Nature Communications.'
Quantum neural networks (QNNs)
The researchers worked on quantum neural networks (QNNs), a type of machine-learning model designed to learn and process information using principles inspired by quantum mechanics in order to mimic the behaviour of quantum systems.
Just like the neural networks used in artificial intelligence, QNNs are made of interconnected nodes, or "neurons," that perform calculations. The difference is that, in QNNs, the neurons operate on the principles of quantum mechanics, allowing them to handle and manipulate quantum information.
"Normally, when we teach a computer something, we need a lot of examples," said Holmes. "But in this study, we show that with just a few simple examples called 'product states' the computer can learn how a quantum system behaves even when dealing with entangled states, which are more complicated and challenging to understand."
Product states
The 'product states' that the scientists used to refer to a concept in quantum mechanics that describes the specific type of state for a quantum system. For example, if a quantum system is composed of two electrons, then its product state is formed when each individual electron's state is considered independently and then combined.
Product states are often used as a starting point in quantum computations and measurements because they provide a simpler and more manageable framework for studying and understanding the behaviour of quantum systems before moving on to more complex and entangled states where the particles are correlated and cannot be described independently.
Better quantum computers ahead
The researchers demonstrated that by training QNNs using only a few of these simple examples, computers can effectively grasp the complex dynamics of entangled quantum systems.
Holmes explained, "This means that might be able to learn about and understand quantum systems using smaller, simpler computers, like the near-term intermediary scale [NISQ] computers we're likely to have in the coming years, instead of needing large and complex ones, which may be decades away."
The work also opens up new possibilities for using quantum computers to solve important problems like studying complex new materials or simulating the behaviour of molecules.
Finally, the method improves the performance of quantum computers by enabling the creation of shorter and more error-resistant programs. By learning how quantum systems behave, we can streamline the programming of quantum computers, leading to improved efficiency and reliability. "We can make quantum computers even better by making their programs shorter and less prone to errors," said Holmes. (ANI)