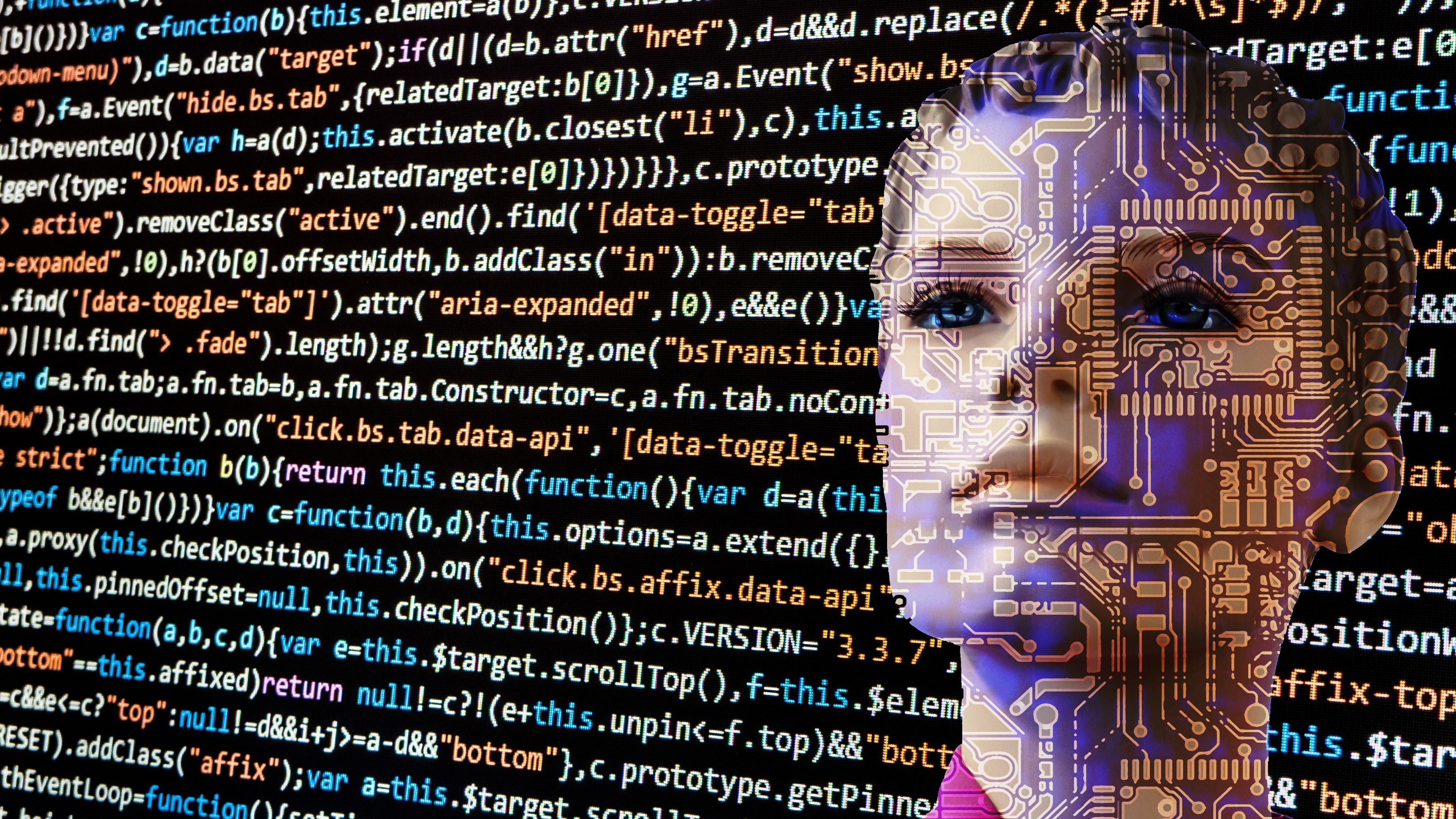
Anyone who has used generative AI for any length of time will be more than familiar with hallucinations. These are when AI systems generate false or misleading information, a flaw often rooted in limitations within their training data or model design. Such inaccuracies can emerge unpredictably and vary widely in their severity - from minor errors to substantial distortions that could significantly skew decision-making processes.
Lamini Memory Tuning aims to significantly reduce hallucinations, from 50% to 5%, a 90% cut. The technology enables the embedding of exact facts into LLMs, reportedly achieving accuracy rates up to 95%, a significant leap from the 50% accuracy offered by previous methods.
By specifically tuning millions of expert adapters, such as LoRAs (Low-Rank Adaptions) on any open-source LLM, Lamini Memory Tuning ensures precise fact retention, ranging from historical events to complex technical data, without the high latency and cost typically associated with such precision.
Mixture of Memory Experts
This method, inspired by mind mapping, selectively activates the most relevant experts from an index during inference, dramatically reducing unnecessary computations.
As an example, the company says when tasked with recalling specific facts about the Roman Empire, the system pulls only the necessary information about Julius Caesar, aqueducts, or legions, avoiding the activation of irrelevant model weights.
The underlying technology behind Lamini Memory Tuning involves a sparse activation framework known as a Mixture of Memory Experts (MoME), which scales to support a vast number of facts limited only by training data size. Lamini says this approach not only enhances model responsiveness but also significantly cuts down on computational demands, making it a viable solution for enhancing the performance of LLMs across various applications.