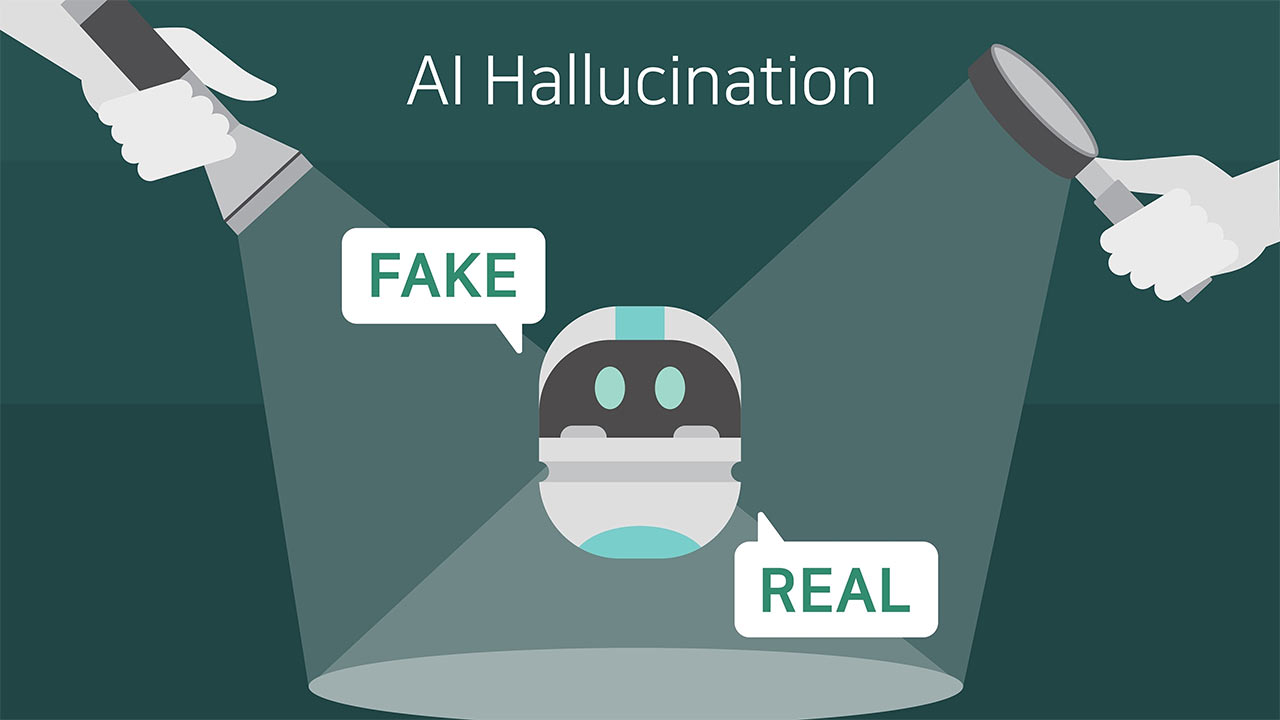
The AI boom has allowed the general consumer to use AI chatbots like ChatGPT to get information from prompts demonstrating both breadth and depth. However, these AI models are still prone to hallucinations, where erroneous answers are delivered. Moreover, AI models can even provide demonstrably false (sometimes dangerous) answers. While some hallucinations are caused by incorrect training data, generalization, or other data harvesting side-effects, Oxford researchers have target the problem from another angle. In Nature, they published details of a newly developed method for detecting confabulations — or arbitrary and incorrect generations.
LLMs find answers by finding particular patterns in their training data. This doesn't always work, as there is still the chance that an AI bot can find a pattern where none exists, similar to how humans can see animal shapes in clouds. However, the difference between a human and an AI is that we know that those are just shapes in clouds, not an actual giant elephant floating in the sky. On the other hand, an LLM could treat this as gospel truth, thus leading them to hallucinate future tech that doesn’t exist yet, and other nonsense.
Semantic entropy is the key
The Oxford researchers use semantic entropy to determine by probability whether an LLM is hallucinating. Semantic entropy is when the same words have different meanings. For example, desert could refer to a geographical feature, or it could also mean abandoning someone. When an LLM starts using these words, it can get confused about what it is trying to say, so by detecting the semantic entropy of an LLM’s output, the researchers aim to determine whether it is likely to be hallucinating or not.
The advantage of using semantic entropy is that it will work on LLMs without needing any additional human supervision or reinforcement, thus making it quicker to detect if an AI bot is hallucinating. Since it doesn’t rely on task-specific data, you can even use it on new tasks that the LLM hasn’t encountered before, allowing users to trust it more fully, even if it’s the first time that AI encounters a specific question or command.
According to the research team, “our method helps users understand when they must take extra care with LLMs and open up new possibilities for using LLMs that are otherwise prevented by their unreliability.” If semantic entropy does prove an effective way of detecting hallucinations, then we could use tools like these to double-check the output accuracy of AI, allowing professionals to trust it as a more reliable partner. Nevertheless, much like no human is infallible, we must also remember the LLMs, even with the most advanced error detection tools, could still be wrong. So, it’s wise to always double-check an answer that ChatGPT, CoPilot, Gemini, or Siri gives you.