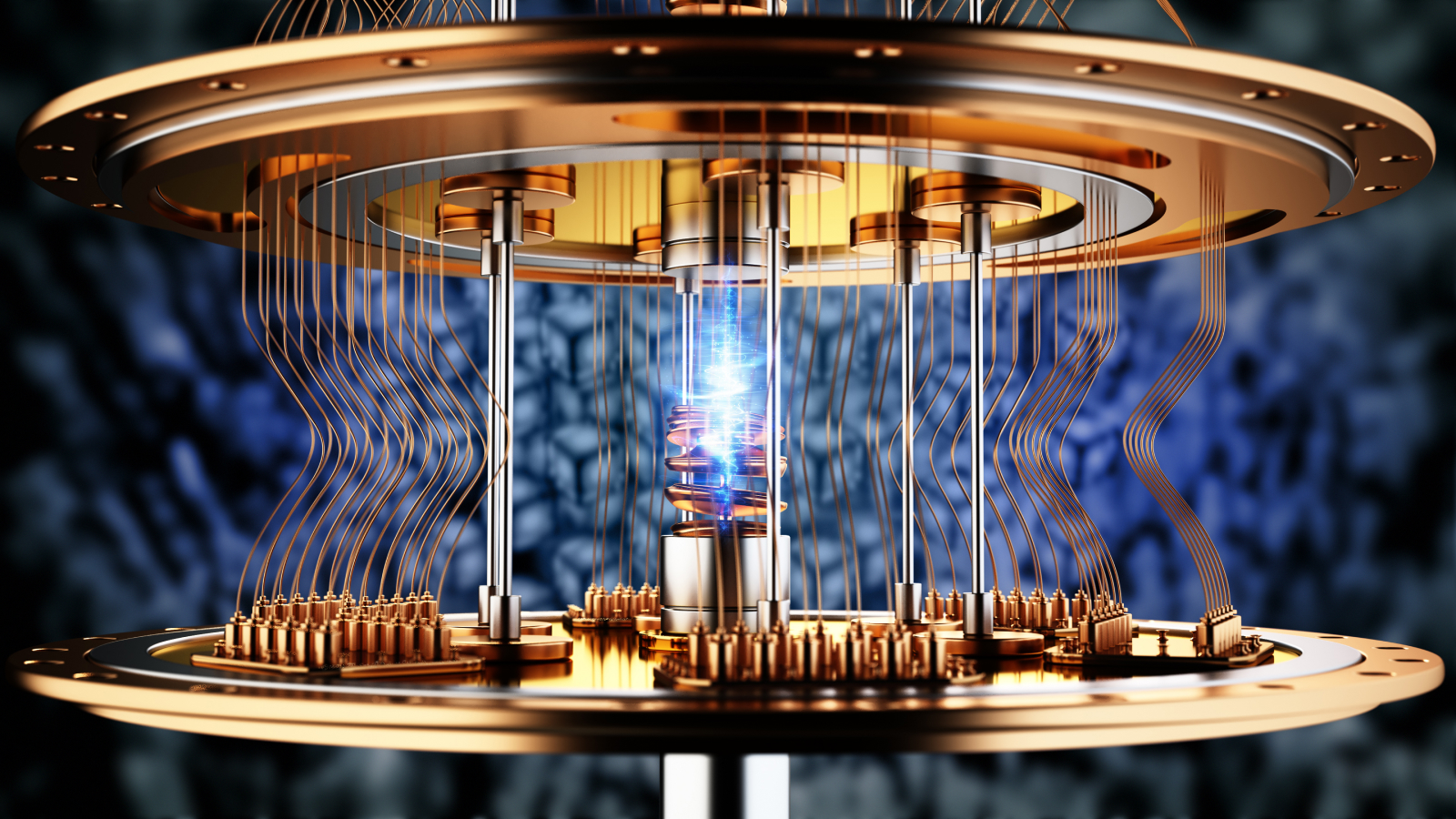
Technology companies are pouring billions of dollars into quantum computing, despite the technology still being years away from practical applications. So what will future quantum computers be used for — and why are so many experts convinced they will be game-changing?
Building a computer that harnesses the unusual properties of quantum mechanics is an idea that has been in contention since the 1980s. But in the last couple of decades, scientists have made significant strides in building large-scale devices. Now, a host of tech giants from Google to IBM as well as several well-funded startups have invested significant sums into the technology — and they have created several individual machines and quantum processing units (QPUs).
In theory, quantum computers could solve problems that are beyond even the most powerful classical computer. However, there’s broad consensus that such devices will need to become much larger and more reliable before that can happen. Once they do, however, there is hope that the technology will crack a host of currently unsolvable challenges in chemistry, physics, materials science and even machine learning.
"It's not just like a fast classical computer, this is a completely different paradigm,” Norbert Lütkenhaus, executive director of the Institute for Quantum Computing at the University of Waterloo in Canada told Live Science. "Quantum computers can solve some tasks efficiently that classical computers simply cannot do."
The current state-of-the-art
The most fundamental building block of a quantum computer is the qubit — a unit of quantum information that is comparable to a bit in a classical computer, but with the uncanny ability to represent a complex combination of both 0 and 1 simultaneously. Qubits can be implemented on a wide range of different hardware, including superconducting circuits, trapped ions or even photons (light particles).
Today’s largest quantum computers have just crossed the 1,000 qubit mark, but most feature just a few tens or hundreds of qubits. They are far more error-prone than classical computing components due to the extreme sensitivity of quantum states to external noise, which includes temperature changes or stray electromagnetic fields. That means that it's currently difficult to run large quantum programs for long enough to solve practical problems.
Related: Radical quantum computing theory could lead to more powerful machines than previously imagined
That doesn’t mean today’s quantum computers are useless, though, said William Oliver, director of the Center for Quantum Engineering at the Massachusetts Institute of Technology (MIT) in the USA. "What quantum computers are used for today is basically to learn how to make quantum computers bigger, and also to learn how to use quantum computers," he said in an interview with Live Science.
Building ever larger processors provides crucial insight into how to engineer larger, more reliable quantum machines and provides a platform to develop and test novel quantum algorithms. They also let researchers test quantum error-correction schemes, which will be crucial for achieving the technology’s full promise. These typically involve spreading quantum information over multiple physical qubits to create a single "logical qubit," which is far more resilient.
Lütkenhaus said that recent breakthroughs in this area suggest fault-tolerant quantum computing might not be so far off. Several companies including QuEra, Quantinuum and Google have recently demonstrated the ability to generate logical qubits reliably. Scaling up to the thousands, if not millions, of qubits that we need to solve practical problems will take time and a lot of engineering effort, says Lütkenhaus. But once that’s been achieved, a host of exciting applications will come into view.
Where quantum could be a game changer
The secret to quantum computing’s power lies in a quantum phenomenon known as superposition, said Oliver. This allows a quantum system to occupy multiple states simultaneously until it is measured. In a quantum computer, this makes it possible to place the underlying qubits into a superposition representing all potential solutions to a problem.
"As we run the algorithm, the answers that are incorrect are suppressed and the answers that are correct are enhanced," said Oliver. "And so by the end of the calculation, the only surviving answer is the one that we're looking for."
This makes it possible to tackle problems too vast to work through sequentially, as a classical computer would have to, Oliver added. And in certain domains, quantum computers could carry out calculations exponentially faster than their classical cousins as the size of the problem grows.
One of the most obvious applications lies in simulating physical systems, said Oliver, because the world itself is governed by the principles of quantum mechanics. The same strange phenomena that make quantum computers so powerful also make simulating many quantum systems on a classical computer intractable at useful scales. But because they operate on the same principles, quantum computers should be able to model the behavior of a wide range of quantum systems efficiently.
This could have a profound impact on areas like chemistry and materials science where quantum effects play a major role, and could lead to breakthroughs in everything from battery technology to superconductors, catalysts and even pharmaceuticals.
Quantum computers also have some less savory uses. Given enough qubits, an algorithm invented by mathematician Peter Shor in 1994 could crack the encryption that underpins much of today’s internet. Fortunately, researchers have devised new encryption schemes that sidestep this risk, and earlier this year the US National Institute of Standards and Technology (NIST) released new "post-quantum" encryption standards that are already being implemented.
Emerging possibilities of quantum computing
Other applications for quantum computers are, at present, somewhat speculative, said Oliver.
There are hopes the technology could prove useful for optimization, which involves searching for the best solution to a problem with many possible solutions. Lots of practical challenges can be boiled down to optimization processes, from easing traffic flows through a city to finding the best delivery routes for a logistics company. Building the best portfolio of stocks for a specific financial goal could also be a possible application.
So far, though, most quantum optimization algorithms offer less than exponential speed-ups. Because quantum hardware operates much slower than current transistor-based electronics, these modest algorithmic speed advantages can quickly disappear when implemented on a real-world device.
At the same time, progress in quantum algorithms has spurred innovations in classical computing. "As quantum algorithm designers come up with different optimization schemes, our colleagues in computer science advance their algorithms and this advantage that we seem to have ends up evaporating," added Oliver.
Other areas of active research with less clear long-term potential include using quantum computers to search large databases or conduct machine learning, which involves analyzing large amounts of data to discover useful patterns. Speed-ups here are also less than exponential and there is the added problem of translating large amounts of classical data into quantum states that the algorithm can operate on — a slow process that can quickly eat into any computational advantage.
But it is still early days, and there is plenty of scope for algorithmic breakthroughs, said Oliver. The field is still in the process of discovering and developing the building blocks of quantum algorithms — smaller mathematical procedures known as "primitives" that can be combined to solve more complex problems.
"We need to understand how to build quantum algorithms, identify and leverage these program elements, find new ones if they exist, and understand how to put them together to make new algorithms," says Oliver.
This should guide the future development of the field, added Lütkenhaus, and is something companies should bear in mind when making investment decisions. "As we push the field forward, don't focus too early on very specific problems," he said. "We still need to solve many more generic problems and then this can branch off into many applications."