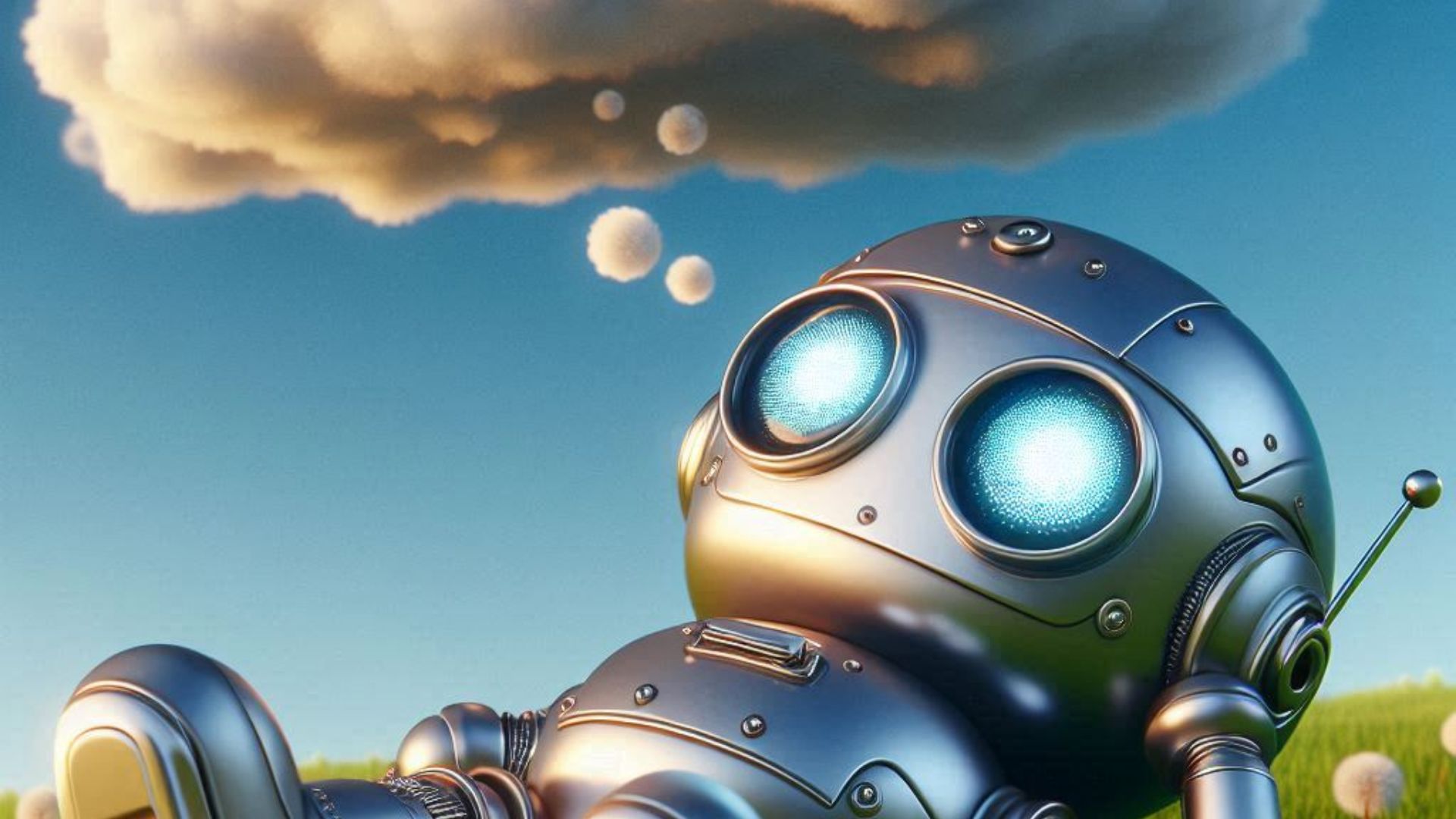
What you need to know
- Aside from privacy and security, hallucination and the spread of misinformation are among the biggest deterrents preventing AI from advancing.
- A new study leverages semantic entropy to assess the quality and different meanings of generated outputs to determine the quality of responses and spot traces of hallucination.
- However, semantic entropy demands more computing power and resources, including time.
AI is revolutionizing how people interact with the internet, which doesn't sit well with publishers, websites, and writers. This is because AI chatbots steal lift information from thoroughly researched articles and generate curated and precise responses to queries. The issue has landed top players in the AI landscape, including OpenAI and Microsoft, in the corridors of justice over copyright infringement issues.
As you may know, AI chatbots like ChatGPT and Microsoft Copilot heavily rely on copyrighted content for their responses. Interestingly, OpenAI CEO Sam Altman admitted it's impossible to develop ChatGPT-like tools without copyrighted content. The ChatGPT maker argued that copyright law doesn't forbid training AI models using copyrighted material.
Perhaps more interestingly, while tools like Copilot and ChatGPT still fend for data from online sources, there have still been reports of hallucinations, the spread of misinformation, or the outright presentation of wrong information. When you launch Copilot in Windows 11, you'll find a disclaimer indicating "Copilot uses AI. Check for Mistakes."
According to a new study, a group of Oxford researchers have seemingly found a way around this critical issue. Copilot has been spotted spreading misinformation about the forthcoming US Presidential elections, with researchers indicating that the problem is systemic after establishing a pattern. With the prevalence of such critical issues and deep fakes, more users are having reservations about the technology and taking everything they see with a pinch of salt.
Prof. Yarin Gal says:
“Getting answers from LLMs is cheap, but reliability is the biggest bottleneck. In situations where reliability matters, computing semantic uncertainty is a small price to pay.”
Misinformation continues to prevail with the rapid adoption of AI
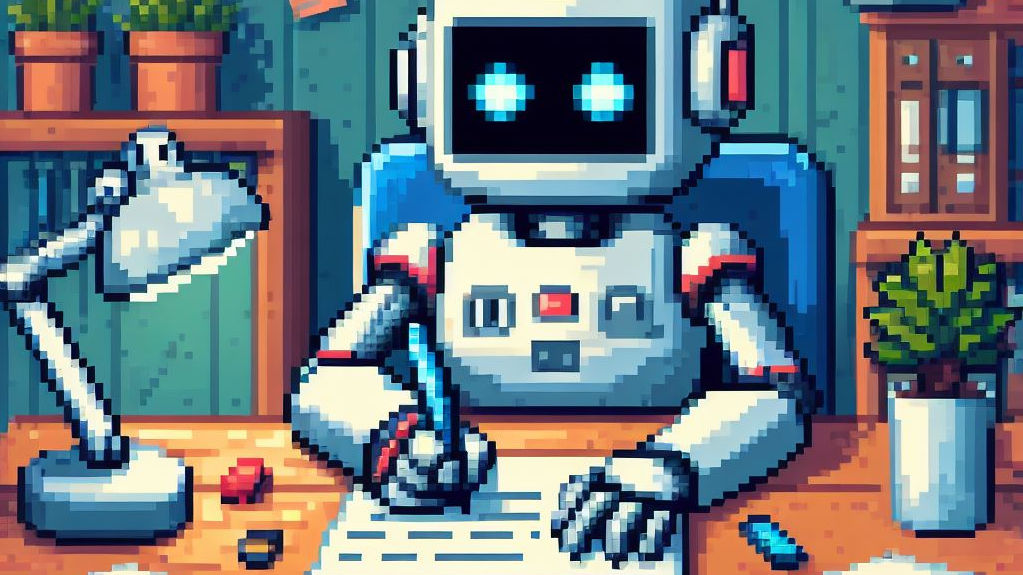
According to Former Twitter CEO Jack Dorsey:
"Don't trust; verify. You have to experience it yourself. And you have to learn yourself. This is going to be so critical as we enter this time in the next five years or 10 years because of the way that images are created, deep fakes, and videos; you will not, you will literally not know what is real and what is fake."
Dorsey adds that everything will soon feel like a simulation as AI models and chatbots become more sophisticated. However, the Oxford researchers at the very least have found a way around the issue, as highlighted in their report:
"With previous approaches, it wasn’t possible to tell the difference between a model being uncertain about what to say versus being uncertain about how to say it. But our new method overcomes this."
AI chatbot hallucination is a broad topic, but the researchers break it down into two parts — "We want to focus on cases where the LLM is wrong for no reason (as opposed to being wrong because, for example, it was trained with bad data),” indicated Dr. Sebastian Farquhar, from the University of Oxford’s Department of Computer Science while speaking to Euronews Next.
The study entailed scrutinizing the varied meanings of the responses generated via semantic entropy, which goes beyond the sequence of the words. Semantic entropy can determine the difference in the meanings of the outputs generated. If the analysis detects a high level of semantic entropy, it essentially means there is a huge difference in the meaning of the generated outputs.
According to Dr. Sebastian Farquhar:
“When an LLM generates an answer to a question you get it to answer several times. Then you compare the different answers with each other. In the past, people had not corrected for the fact that in natural language there are many different ways to say the same thing. This is different from many other machine learning situations where the model outputs are unambiguous."
The research was conducted on six models, including OpenAI's GPT-4. The researchers' findings indicate semantic entropy is more efficient and effective at spotting questions picked from Google searches, technical biomedical questions, and more compared to other methods prone to wrong responses.
The only downside of semantic entropy is that it requires more computing power and resources.