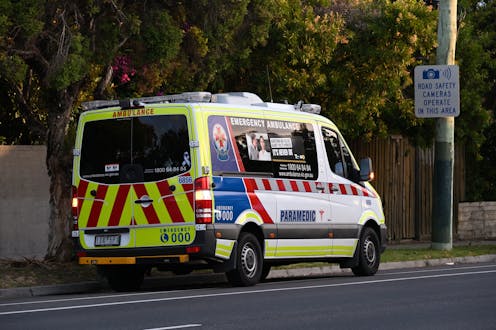
In Australia, stroke is among the leading causes of death and permanent disability. Some 5% of deaths are due to stroke, while strokes cost the Australian health-care system A$6.2 billion annually.
Strokes occur when there’s a sudden loss of blood flow in the brain. This prevents the brain tissue from getting the oxygen and nutrients it needs, which can lead to damage to sections of the brain.
Timely stroke treatment can limit brain damage and improve outcomes for patients. But this depends on early recognition of the symptoms, which is not always easy.
Our team has developed a new smartphone app to screen a person’s facial expressions and detect whether they’ve had a stroke. We’ve recently published the results of a pilot study of this tool, and found it could identify if someone has had a stroke quickly and relatively accurately.
Scanning facial expressions
One of the earliest external symptoms of stroke can be found in facial expressions such as droop, where one side of the mouth is not activated when a person tries to smile.
However, paramedics responding to emergencies and hospital emergency department staff often miss stroke cases. Facial expressions are naturally different between people, and identifying subtle changes in a high-stress environment is challenging. This can become even more difficult if the patient is from a different ethnicity or cultural background.
With our smartphone app, a paramedic or other first responder asks the patient to try to smile, and “films” the patient’s face while they do so. An AI-based model then analyses the video recording, looking for similar signs as used by clinicians to identify stroke, namely the asymmetrical drooping of the mouth.
The app is designed for simplicity – the user just has to point the camera to the patient and press a button. To ensure the patient’s privacy, the video is analysed in real time and does not have to be stored. This device would only need a smartphone, so would be easy to deploy, and would be a cost-effective solution.
The idea is that first responders such as paramedics or nurses in the emergency department would have this app on their smartphones. When they first see a patient who has experienced a medical emergency, they can use the app to detect if the patient may have suffered a stroke in seconds. That way, treatment can be fast-tracked accordingly.
Our pilot study
We tested the tool on a small dataset, using video recordings of 14 people who had experienced a stroke, and 11 healthy controls.
We found it was 82% accurate, meaning it correctly identified a stroke 82% of the time. Our tool is not designed to replace comprehensive clinical diagnostic tests for stroke, but it could help identify people needing treatment much sooner and assist clinicians.
While these results are promising, we’re planning to continue to optimise the model. Our hope is the accuracy will improve as we build a bigger dataset, with recordings of more patients.
At this stage, the AI model has only been trained and developed on a small dataset, and the data lacks diversity in ethnicity and demographics. It will be essential to refine and test the app for people of different cultural and ethnic backgrounds.
Down the track, we plan to partner with clinicians, emergency departments and ambulance services to conduct clinical trials. We’ll need to test the effectiveness of this tool in the hands of the actual users, such as paramedics, to confirm it helps them look after their patients.

The authors do not work for, consult, own shares in or receive funding from any company or organisation that would benefit from this article, and have disclosed no relevant affiliations beyond their academic appointment.
This article was originally published on The Conversation. Read the original article.