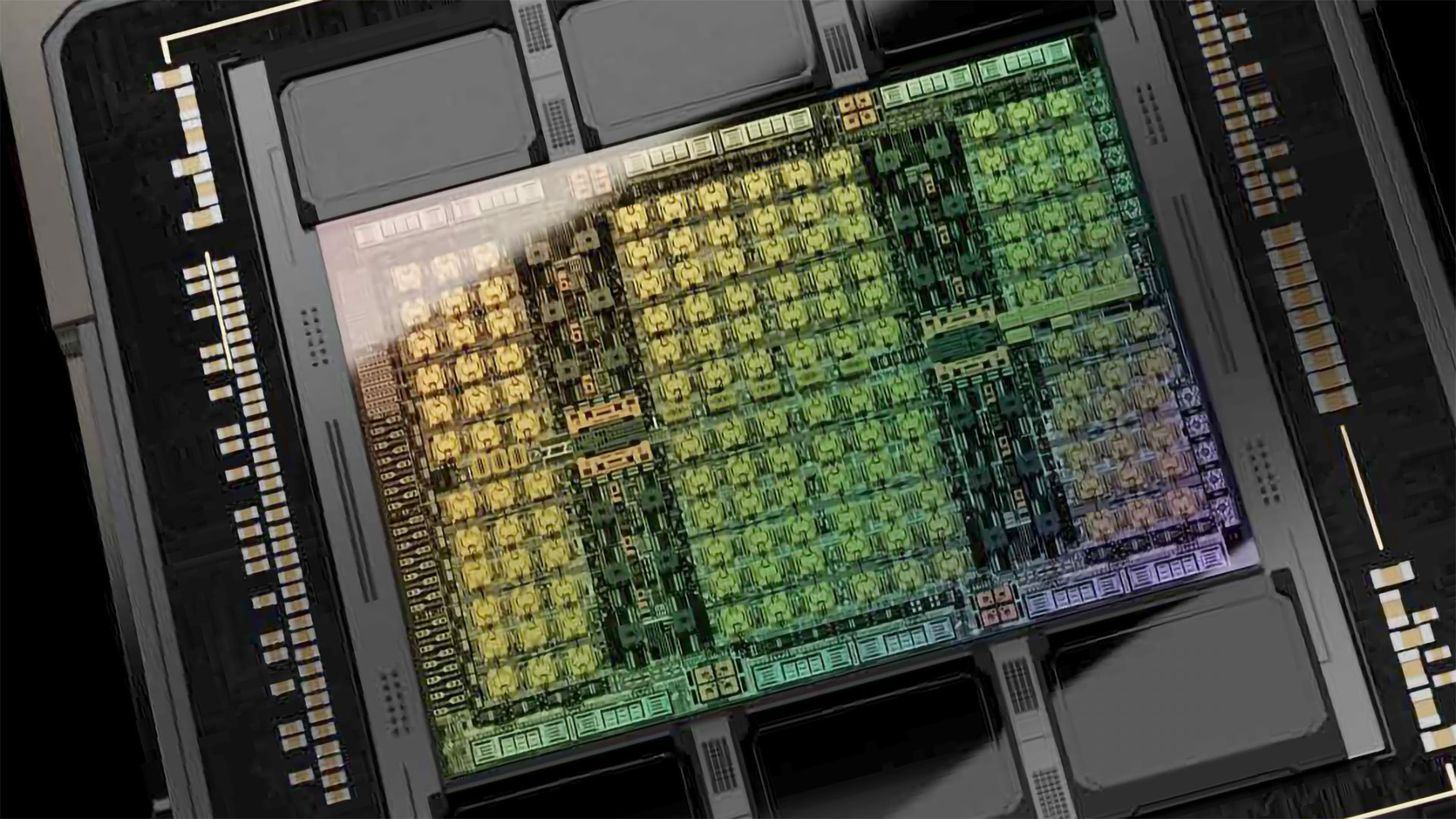
Nvidia's skyrocketing rise in 2023 and 2024 was fueled by the explosive demand for GPUs in the AI sector, mostly in the U.S., Middle-Eastern countries, and China. Since there are U.S. export restrictions and Nvidia cannot sell its highest-end Hopper H100, H200, and H800 processors to China without an export license from the government, it instead sells its cut-down HGX H20 GPUs to entities in China. However, while being cut down, the HGX H20 performs extraordinarily well in terms of sales, according to analyst Claus Aasholm. You can see the product's sales performance in the table embedded in the tweet below.
From @clausaasholm "the downgraded [Nvidia] H20 system that passes the embargo rules for China is doing incredibly well. With 50% growth, quarter over quarter.." pic.twitter.com/YdpvrRrvheDecember 27, 2024
"The downgraded H20 system that passes the embargo rules for China is doing incredibly well," wrote Aasholm. "With 50% growth, quarter over quarter, this is Nvidia's most successful product. The H100 business 'only' grew 25% QoQ."
Based on Claus Aasholm's findings, Nvidia earns tens of billions of dollars selling the HGX H20 GPU despite its seriously reduced performance compared to the fully-fledged H100. Artificial intelligence is indeed a megatrend that drives sales of pretty much all types of data center hardware, including Nvidia's Hopper GPUs, including the HGX H20.
The world's leading economies — the U.S. and China — are racing to gain maximum AI capabilities. For America, the growth is more or less natural: more money and more hardware equal higher capabilities, yet it is not enough. OpenAI alone earns billions, but it needs more to gain more hardware and, therefore, AI training and inference capabilities.
Despite all restrictions, China's AI capabilities — both in hardware and large-model development — are expanding. Just last week, it turned out that Chinese AI company Deepseek revealed in a paper that it had trained its 671-billion-parameter DeepSeek-V3 Mixture-of-Experts (MoE) language model on a cluster of 2,048 Nvidia H800 GPUs and that it took two months, a total of 2.8 million GPU hours. By comparison, Meta invested 11 times the compute resources (30.8 million GPU hours) to train Llama 3, which has 405 billion parameters, using 16,384 H100 GPUs over 54 days.
Over time, China's domestic accelerators from companies like Biren Technologies and Moore Threads might eat into what is now a near-monopoly for Nvidia in Chinese data centers. However, this simply cannot happen overnight.