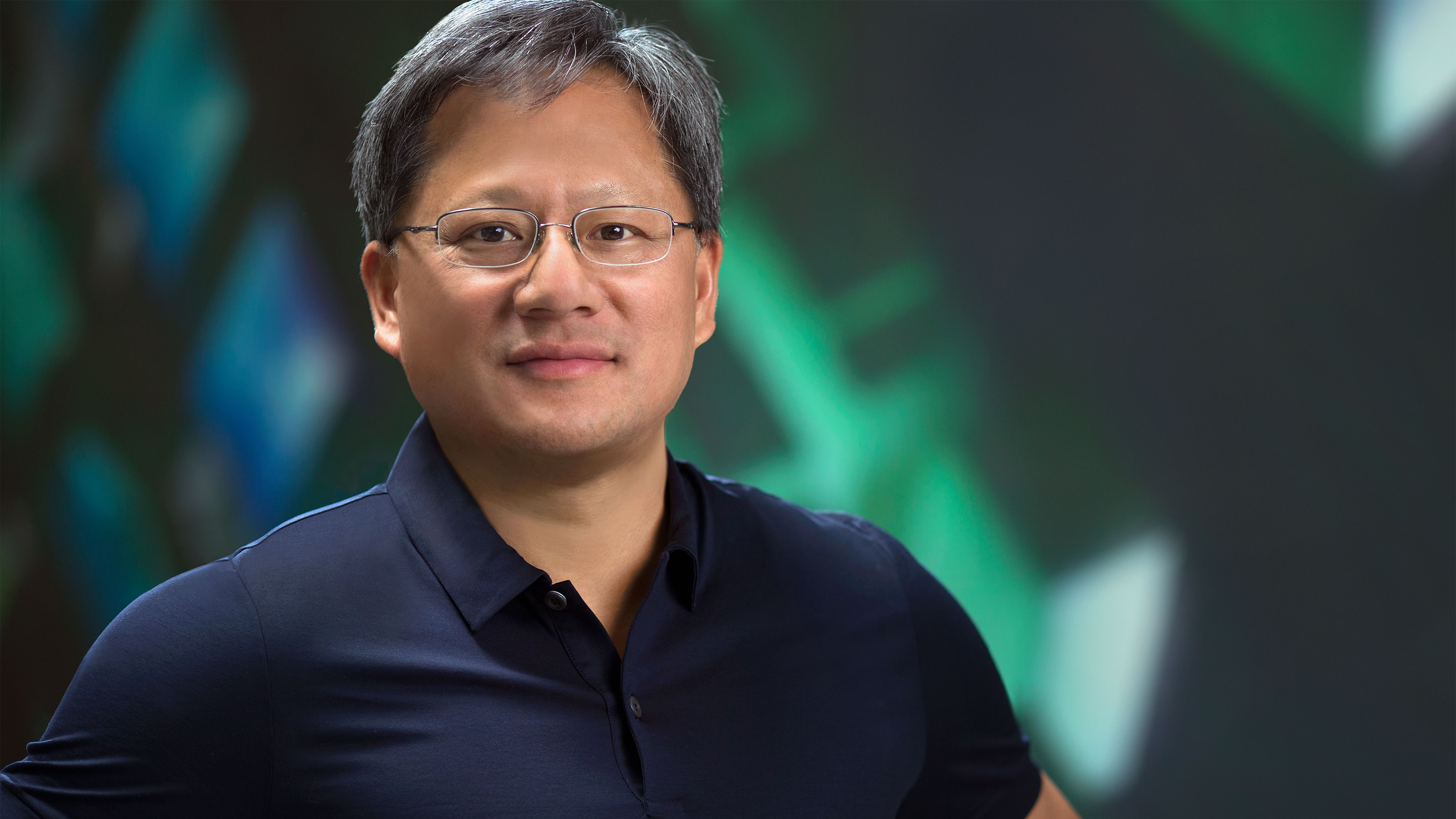
When we first heard that OpenAI's head, Sam Altman, was looking to build a chip venture, we were impressed but considered it as another standard case of a company adopting custom silicon instead of using off-the-shelf processors. However, his reported meetings with potential investors to reportedly raise $5 to $7 trillion to build a network of fabs for AI chips is extreme given that the entire world's semiconductor industry is estimated to be around $1 trillion per year. Nvidia's Jensen Huang doesn't believe that much investment is needed to build an alternative semiconductor supply chain just for AI. Instead, the industry needs to continue its GPU architecture innovations to continue improving performance — in fact, Huang claims that Nvidia has already increased AI performance 1 million fold over the last ten years.
"If you just assume that that computers never get any faster you might come to the conclusion [that] we need 14 different planets and three different galaxies and four more Suns to fuel all this," said Jensen Huang at the World Government Summit.
Investing trillions of dollars to build enough chips for AI data centers can certainly solve the problem of shortages in the course of the next three to five years. However, the head of Nvidia believes that creating an alternative semiconductor supply industry just for AI may not be exactly the best idea since, at some point, it may lead to an oversupply of chips and a major economic crisis. A shortage of AI processors will eventually be solved, partly by architectural innovations, and companies who want to use AI on their premises will not need to build a billion-dollar data center.
"Remember that the performance of the architecture is going to be improving at the same time so you cannot assume just that you will buy more computers," said Huang. "You have to also assume that the computers are going to become faster, and therefore, the total amount that you need is not going to be as much."
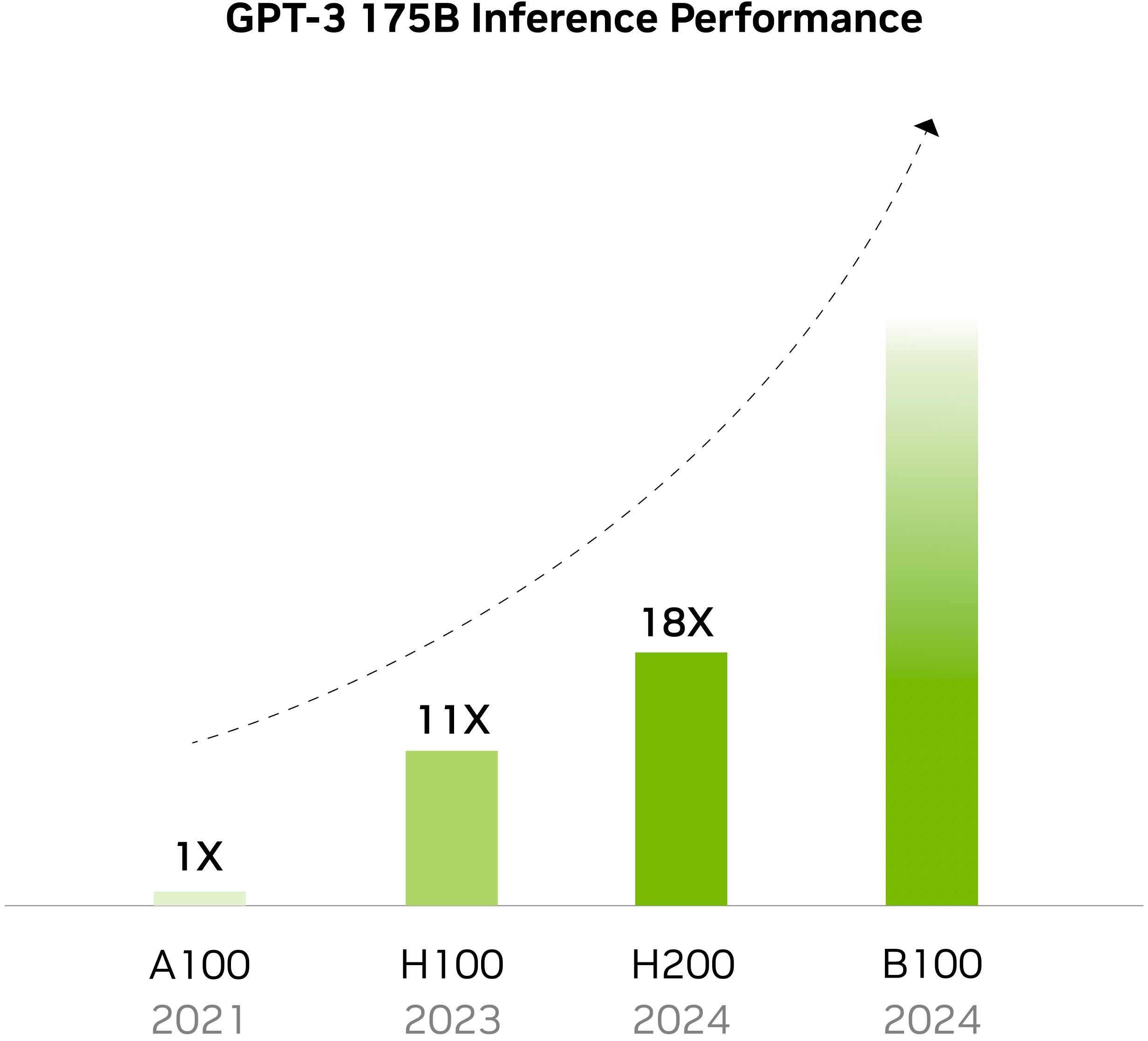
Indeed, Nvidia's GPUs evolve very fast when it comes to AI and high-performance computing (HPC) performance. The half-precision compute performance of Nvidia's V100 datacenter GPU was a mere 125 TFLOPS in 2018, but Nvidia's modern H200 provides 1,979 FP16 TFLOPS.
"One of the greatest contributions, and I really appreciate you mentioning that, is the rate of innovation," Huang said. "One of the greatest contributions we made was advancing computing and advancing AI by one million times in the last ten years, and so whatever demand that you think is going to power the world, you have to consider the fact that [computers] are also going to do it one million times faster [in the next ten years]."