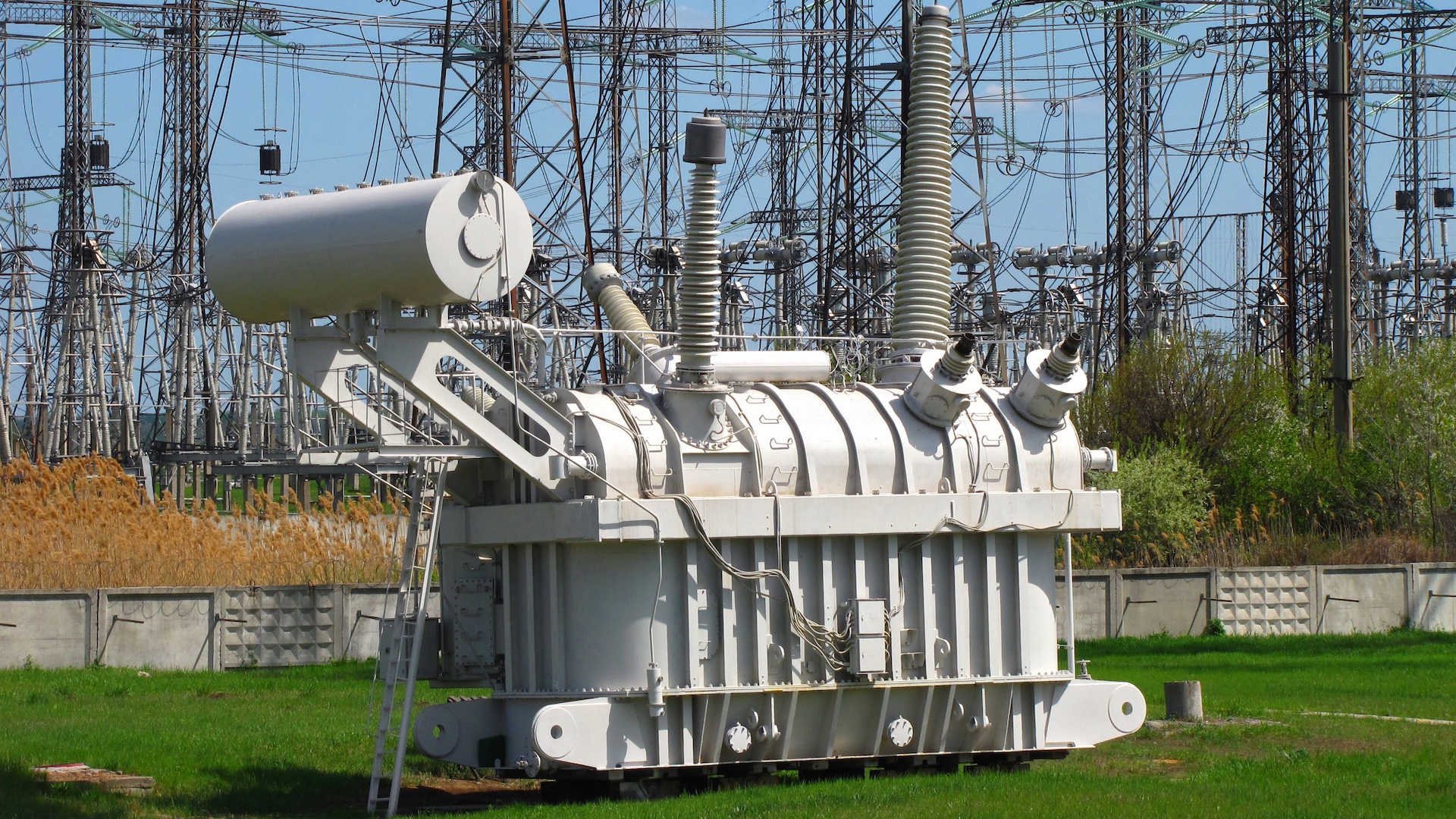
Artificial intelligence (AI) computing requires tremendous amounts of electricity, but targeted research might hold the key to greatly reducing that. However, a team of researchers in the U.S. has developed technology that could reduce the energy consumption required by AI processing by a factor of at least a thousand.
A group of engineering researchers at the University of Minnesota Twin Cities have demonstrated an AI efficiency-boosting technology and published a peer-reviewed paper outlining their work and findings. The paper was published in npj Unconventional Computing, a peer-reviewed journal by Nature. In essence, they’ve created a shortcut in the normal practice of AI computations that greatly reduces the energy requirement for the task.
In current AI computing, data is transferred between the components processing it (logic) and where data is stored (memory/storage). This constant shuttling of information back and forth is responsible for consuming as much as 200 times the energy used in the computation, according to this research.
Thus the researchers have turned to Computational Random-Access Memory (CRAM) to address this. The CRAM the research team has developed places a high-density, reconfigurable spintronic in-memory compute substrate within the memory cells themselves.
This differs from existing processing-in-memory solutions such as Samsung's PIM technology, as Samsung's solution places a processing computing unit (PCU) within the memory core. Data still has to travel from memory cells to the PCU and back, just not nearly as far.
Using CRAM, the data never leaves memory, instead undergoing processing entirely within the computer’s memory array. According to the research team, this allows the system running the AI computing application an energy consumption improvement “on the order of 1,000x over a state-of-the-art solution.”
Other examples suggest the potential for even greater energy savings and faster processing. In one test, performing an MNIST handwritten digit classifier task, the CRAM proved 2,500 times more energy-efficient and 1,700 times as fast as a near-memory processing system using the 16nm technology node. This task is used to train AI systems to recognize handwriting.
The importance of this kind of work cannot be overstated. Recent reports suggest AI workloads already consume almost as much electricity as the entire nation of Cyprus did in 2021. The total energy consumption came in at 4.3 GW in 2023 and is expected to grow at a rate of 26% to 36% in the coming years. Arm’s CEO recently suggested that by 2030, AI may consume a quarter of all energy produced in the U.S.
The first author of the paper Yang Lv, a University of Minnesota Department of Electrical and Computer Engineering postdoctoral researcher, and the rest of the research team have already applied for several patents based on the new technology. They plan to work with leaders in the semiconductor industry, including those in Minnesota, to provide large-scale demonstrations and produce the hardware to help advance AI functionality while also making it more efficient.