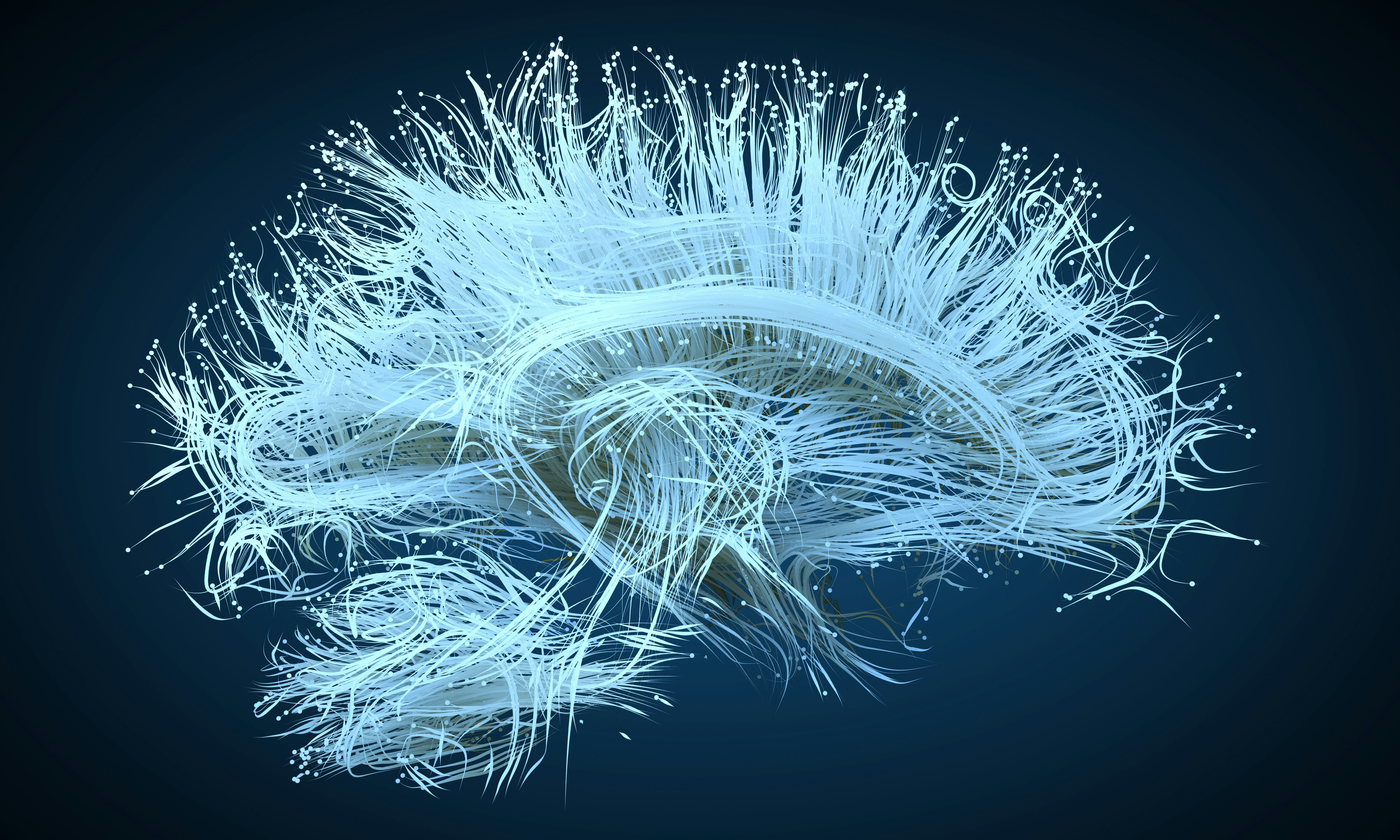
In an episode of Netflix’s Black Mirror, an insurance agent uses a machine — outfitted with a visual monitor and brain sensors — to read people’s memories to help her investigate an accidental death.
While mind-reading machines aren’t real, brain-computer interfaces, which are in various stages of development, establish a communication pathway between the brain and a computer, enabling individuals with neurological or other disorders to regain motor function, talk to their loved ones, or potentially reverse blindness, though they often involve some sort of implantable device.
Now, researchers are one step closer to enabling a computer to read our minds sans surgery.
In a paper published Monday in the journal Nature Neuroscience, researchers at the University of Texas at Austin created a speech decoder capable of converting thoughts into words using brain activity collected from functional MRI (or fMRI), a type of MRI that measures changes in the brain’s blood oxygen levels. The speech decoder, powered by the same sort of AI behind ChatGPT, only gets a general sense of what someone is thinking, not the exact words. However, it is among the first brain decoders not to rely on an implantable device. With more research, it may enable communication that brain-computer interfaces aren’t capable of. However, this type of technology comes with an abundance of ethical and privacy issues.
Reading thoughts with neural networks
Functional MRIs detect changes in your brain’s blood oxygen levels, specifically the ratio of oxygenated to deoxygenated blood which occurs as a result of neural activity. (The more active your neurons are, the more oxygen and glucose they consume.)
To get their speech decoder to recognize an individual’s thoughts using this brain activity proxy, the researchers, led by Alexander Huth, an assistant professor of neuroscience and computer science at the University of Texas at Austin, first had to train the AI with fMRI data. This involved having three volunteers lying inside a bulky MRI machine for about 16 hours each listening to audio stories, a bulk of which came from The Moth Radio Hour and podcasts like The New York Times’ Modern Love and the TED Radio Hour.
“What we were going for was mostly what is interesting and fun for the subjects to listen to because I think that’s really critical in actually getting good [functional] MRI data, doing something that won’t bore your subjects out of their skulls,” said Huth in a press briefing.
The participants also watched silent videos still rich in story, such as Pixar shorts and other animated videos.
While the subjects were chilling with their podcasts, the AI examined the wealth of data — which the researcher said was over five times larger than any existing language set — using an encoding model, which takes the words a subject is hearing and predicts how their brain will respond. (This part relies on GPT, the granddaddy of all AI chatbots.)
The AI’s function is a bit different than your run-of-the-mill chabot since the researchers made it capable of decoding as well. This involves the AI guessing sequences of words a subject might have heard and checking how closely its guess resembled the actual words. It would then predict how a human brain would respond to the guess word and compare its educated guess against actual brain responses.
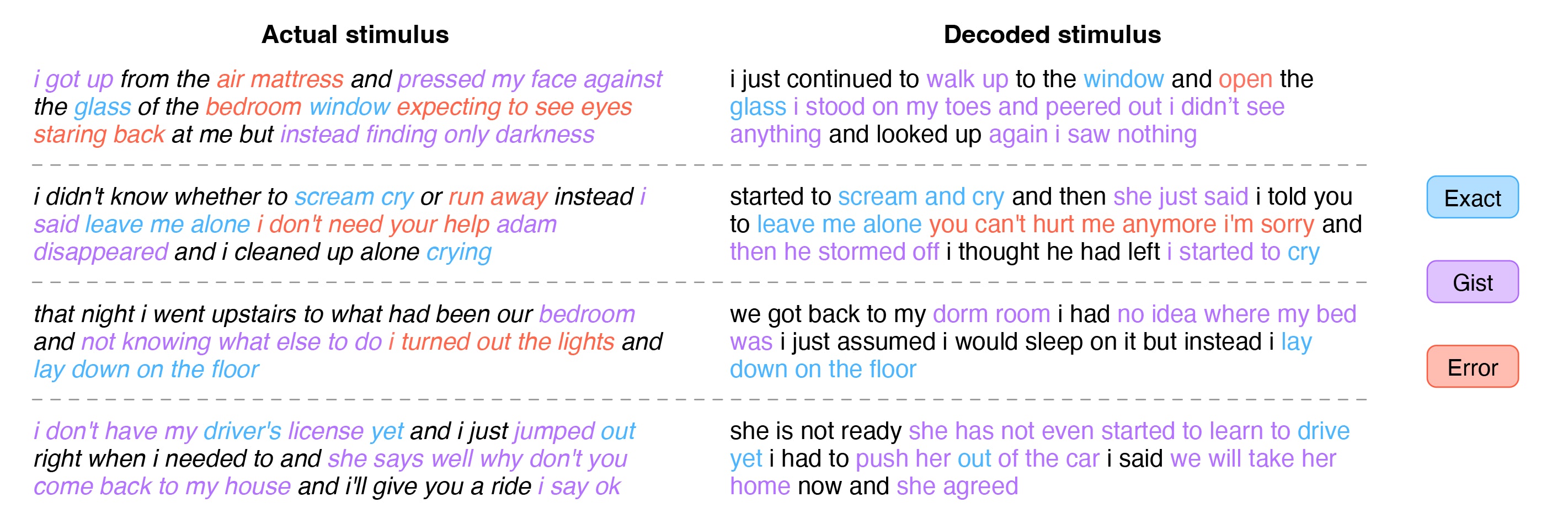
Its word-for-word error rate was pretty high, between 92 and 94 percent. The AI isn’t able to convert thoughts into someone’s exact words or sentences, rather it paraphrases, Jerry Tang, the paper’s first author and doctoral student at the University of Texas at Austin, said in a press briefing.
“For instance, in one example, the user heard the words ‘I don’t have my driver’s license yet’, and the decoder predicted the words ‘She has not even started to learn to drive yet,’” said Tang.
The advent of mental privacy
Huth says what makes this brain-computer interface approach unique, aside from being non-invasive, is that it looks at speech at the level of ideas rather than at the tail-end, which most existing speech decoding systems do.
“Most of the existing systems work by looking at the last stage of speech output. They record from motor areas of the brain, these are the areas that control the mouth, larynx, [and] tongue,” said Huth. “What they can decode is how the person’s trying to move their mouth to say something, which can be very effective and seems to work quite well [such as] for locked-in syndrome.”
But it’s this top-down sort of processing that makes this particular speech decoder not a one-size-fits-all. Huth and his colleagues found a decoder trained on one subject’s functional MRI data couldn’t be applied to another. An individual also had to actually be paying attention. Otherwise, the AI decoder couldn’t pick up the story from the brain signals.
“You can’t just run [this AI] on a new person,” said Huth. “Even though, in broad strokes, what our brains are doing are quite similar, the fine details — which is what these models are really getting at in these cubic millimeters of the cortex — that appears to be pretty important here in terms of getting out this information.”
The data could even be sabotaged, in a sense, if a subject was doing anything but paying attention to the story, such as telling another story over the one being listened to or played, counting numbers, or thinking about their favorite fuzzy animal.
The fact that the AI’s mind-reading abilities aren’t perfect is a sigh of relief. But it doesn’t do away with the ethical issues that may invariably present once this technology gains momentum. Huth and Tang acknowledged the potential for mental privacy concerns and the need for policymakers to erect effective guardrails.
“What we’re also concerned [is that] a decoder doesn’t need to be accurate for it to be used in bad ways,” said Tang, citing the polygraph as a cautionary example. “While this technology is in its infancy, it’s very important to regulate what brain data can and cannot be used for. If one day it does become possible to get accurate decoding without a person’s cooperation, we will have a regulatory foundation in place that we can build off of.”
Right now, the AI decoder is a proof-of-concept that isn’t exactly portable since it needs functional MRI data. However, Huth and Tang said their lab is exploring ways to make the technology portable with other non-invasive neuroimaging techniques such as electroencephalogram, magnetoencephalography, and one, perhaps most promising, functional near-infrared spectroscopy (fNIRS).
“Functional near-infrared spectroscopy is the most similar to functional MRI since it’s measuring fundamentally the same signal,” said Huth. “Our techniques should be able to apply pretty much wholesale to fNIRS recordings, and we’re working with some folks to test this out.”
It looks like it will be a long time before we’ll ever see this technology applied Black Mirror-style — and let’s hope that day never comes.