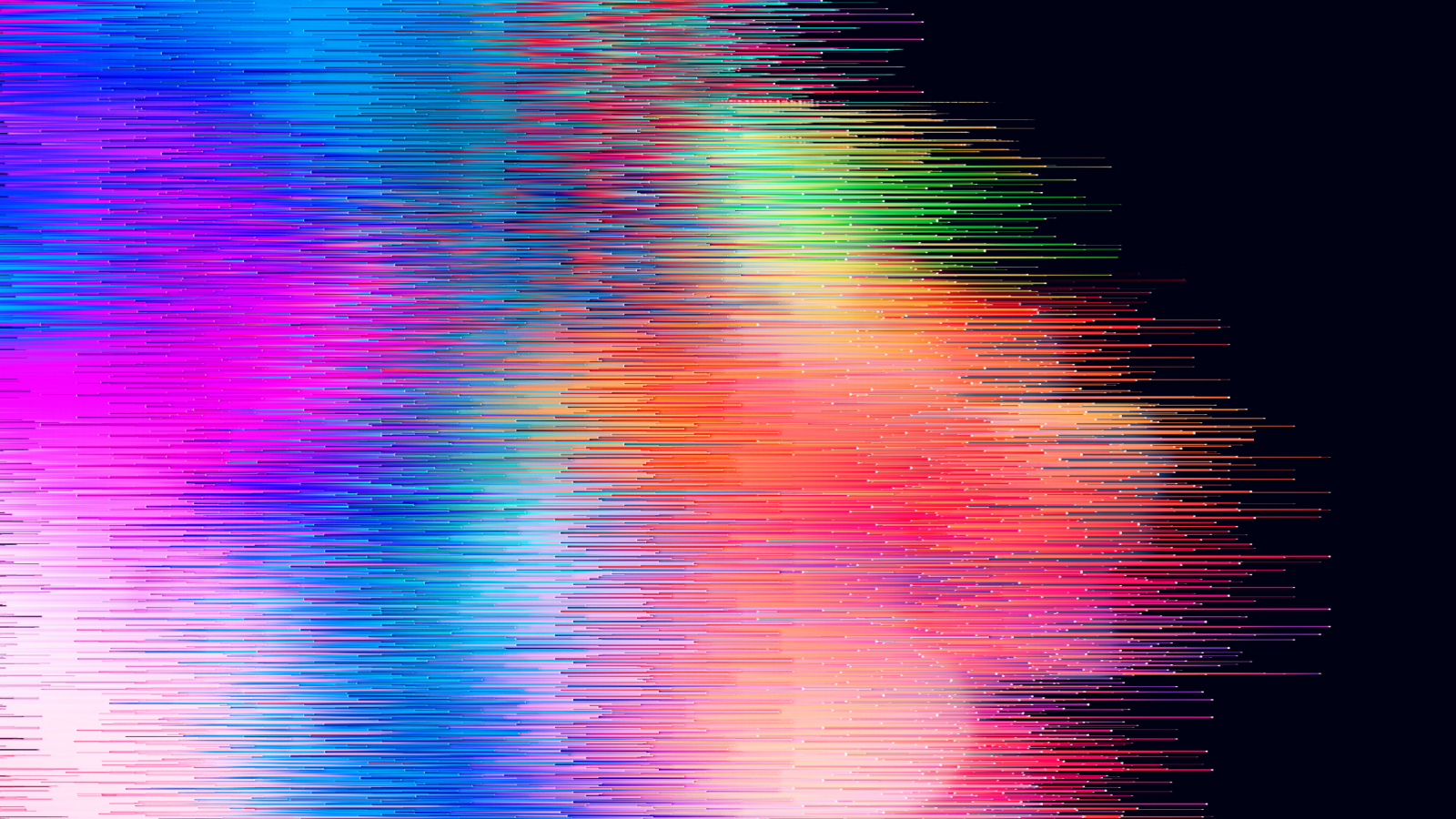
Popular artificial intelligence (AI) powered image generators can run up to 30 times faster thanks to a technique that condenses an entire 100-stage process into one step, new research shows.
Scientists have devised a technique called "distribution matching distillation" (DMD) that teaches new AI models to mimic established image generators, known as diffusion models, such as DALL·E 3, Midjourney and Stable Diffusion.
This framework results in smaller and leaner AI models that can generate images much more quickly while retaining the same quality of the final image. The scientists detailed their findings in a study uploaded Dec. 5, 2023, to the preprint server arXiv.
"Our work is a novel method that accelerates current diffusion models such as Stable Diffusion and DALLE-3 by 30 times," study co-lead author Tianwei Yin, a doctoral student in electrical engineering and computer science at MIT, said in a statement. "This advancement not only significantly reduces computational time but also retains, if not surpasses, the quality of the generated visual content.
Diffusion models generate images via a multi-stage process. Using images with descriptive text captions and other metadata as the training data, the AI is trained to better understand the context and meaning behind the images — so it can respond to text prompts accurately.
Related: New AI image generator is 8 times faster than OpenAI's best tool — and can run on cheap computers
In practice, these models work by taking a random image and encoding it with a field of random noise so it is destroyed, explained AI scientist Jay Alammar in a blog post.This is called "forward diffusion," and is a key step in the training process. Next, the image undergoes up to 100 steps to clear up the noise, known as "reverse diffusion" to produce a clear image based on the text prompt.
By applying their new framework to a new model — and cutting these "reverse diffusion" steps down to one — the scientists cut the average time it took to generate an image. In one test, their model slashed the image-generation time from approximately 2,590 milliseconds (or 2.59 seconds) using Stable Diffusion v1.5 to 90 ms — 28.8 times faster.
DMD has two components that work together to reduce the number of iterations required of the model before it spits out a usable image. The first, called "regression loss," organizes images based on similarity during training, which makes the AI learn faster. The second is called "distribution matching loss," which means the odds of depicting, say, an apple with a bite taken out of it corresponds with how often you're likely to encounter one in the real world. Together these techniques minimize how outlandish the images generated by the new AI model will look.
"Decreasing the number of iterations has been the Holy Grail in diffusion models since their inception," co-lead author Fredo Durand, professor of electrical engineering and computer science at MIT, said in the statement. "We are very excited to finally enable single-step image generation, which will dramatically reduce compute costs and accelerate the process."
The new approach dramatically reduces the computational power required to generate images because only one step is required as opposed to "the hundred steps of iterative refinement" in original diffusion models, Yin said. The model can also offer advantages in industries where lightning-fast and efficient generation is crucial, the scientists said, leading to much quicker content creation.