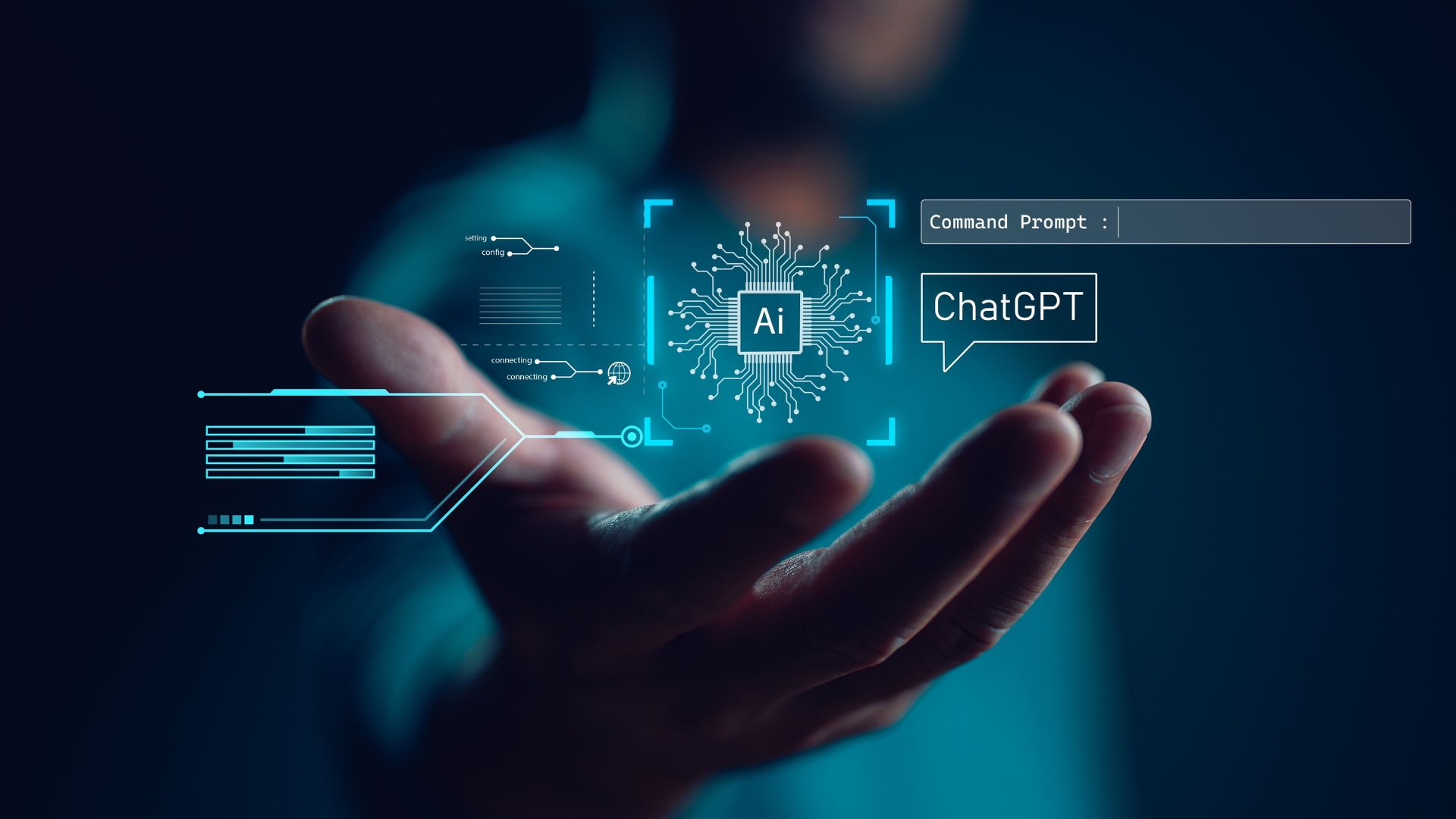
Even in this new era of AI, the old computer science adage of “Garbage in, garbage out” remains as relevant today, if not more relevant, than ever before. Using data that is “ML model ready” is the difference between effective and ineffective AI implementation.
When it comes to training effective Machine Learning (ML) models, engineers are increasingly battling against messy data. This creates a challenge for those who are expected to make sense of and order these data sets for AI tools.
So, how can the data scientists and data engineers of the world ensure that all data is truly “ML model ready”?
Unstructured and heterogeneous data: the enemy of AI projects
The main challenge when dealing with unstructured and heterogeneous data sources comes back to the fact that ML models rely heavily on the data that they are trained on, and if this data were to change unexpectedly, it would have a significant impact on the model’s overall performance. With this in mind, it is crucial to understand where your data comes from to prevent exposing your ML model to unsourced information, which may cause it to make incorrect predictions or decisions.
To help combat this issue, engineers should enforce a dedicated data lineage and data change function to help mitigate against “bad data”. A data lineage process involves tracking data through its entire lifecycle. By creating a clear audit trail of this information, businesses can monitor any changes and understand the data source to ensure that ML models run as efficiently as possible.
Alongside data lineage, another data processing technique that should be leveraged is semantic modelling. Semantic modelling allows organizations to improve the quality of their data by representing all data in a way that accurately captures its source, allowing you to understand the significance of the data, along with its intended use. This process allows organizations to make more accurate interpretations of all data and ensure it is processed in the most efficient way possible - leading to enhanced ML model performance.
By taking advantage of data lineage and data change functions, ML models will be built on a more reliable foundation, improving the trustworthiness of its decision making capabilities and overall performance.
How well an ML model performs is directly dependent on the accuracy of the data that it is trained on, so leveraging these techniques will ensure that ML models are effective down to its foundations.
The importance of considering ethics at every turn
Ethics is a critically important, but often overlooked part of the AI implementation process. Building and deploying AI safely and responsibly is a challenge faced by all businesses - but there are a couple of key ways companies can address these challenges. Firstly, organizations should make certain that there is always a human in the loop during the implementation process. This acts as an extra layer of security and allows businesses to identify and address any biases in the training data while also bringing ethical judgement capabilities to the training process - which are both extremely important steps.
Finally, by leveraging data lineage and semantic descriptions, businesses will be able to fully understand the lifecycle of all data and have the additional context behind it, including its structure and relationships with other data sets, thanks to semantic descriptions. Therefore, monitoring data lineage and leveraging semantic descriptions can support compliance with data protection and management policies from the offset by assigning permissions for data usage - further helping to mitigate ethical issues.
With AI implementation becoming a key priority for businesses as they look to streamline processes and enhance overall products and services, it is vital that their ML models are being trained effectively and that ethics are considered at every turn. Without ethical consideration and thoughtful data processing practices, businesses risk creating ineffective and unethical ML models that lead to inadequate AI implementation.
We list the best data visualization tools.
This article was produced as part of TechRadarPro's Expert Insights channel where we feature the best and brightest minds in the technology industry today. The views expressed here are those of the author and are not necessarily those of TechRadarPro or Future plc. If you are interested in contributing find out more here: https://www.techradar.com/news/submit-your-story-to-techradar-pro