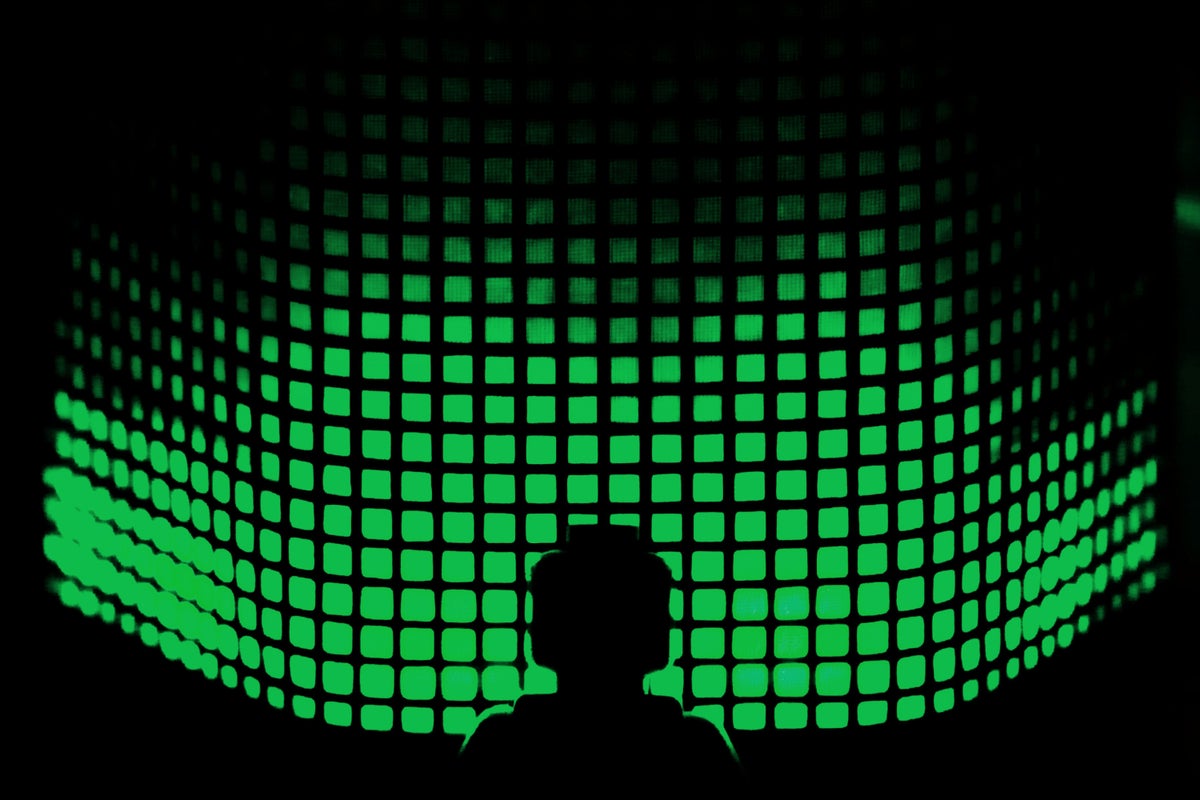
Soda is a Business Reporter client
Today’s data teams need an integrated platform that creates end-to-end observability to ensure data quality remains trustworthy
The shift to digital automation is accelerating how businesses use data products to automate processes, create innovative products and services and deliver insights and efficiencies.
Built using ever-increasing volumes of data from different sources, data products use data to drive outcomes and transform the way a business operates. The challenge for any organisation is to be able to continuously monitor and manage these data products once they are in production. As these products are fed from an increasing number of data sources, inevitable changes, broken transformation logic and concept drift will all impact the quality, reliability and, inevitably, trust in the data.
As such, today’s data teams need clear, comprehensive workflows to find, analyse and resolve data quality and reliability issues from ingestion through to consumption. They need to be able to automatically alert the right people at the right time to get ahead of data issues before there is a downstream impact on the business.
Soda is data reliability and quality company that provides open-source tools and a cloud platform that together enable data teams to create the observability they need across the entire data-product lifecycle. We believe in bringing everyone in the digital world closer to their data so they can confidently make data-informed decisions.
To empower and include everyone in the mission to establish and maintain reliable data, we provide:
- Automated data monitoring
- Data health dashboards
- Data reliability checks as-code
- Data sharing agreements
- Incident resolution
It is estimated that by the end of 2022, organisations will rigorously track data quality levels via metrics, increasing data quality by 50 per cent to significantly reduce operational risks and costs. Anomaly detection is playing a huge part in this shift. To detect outlier or abnormal data points across large volumes of data, teams can use anomaly detection to apply machine learning algorithms that learn normal patterns over time. Where the behaviour of a dataset is known and predictable, users can (and should!) manually configure threshold-based monitors to define anomalous behaviour. It is important that users – the subject matter experts – can provide feedback to train the algorithm so that it can continue to learn and adapt to seasonality and acceptable variations in data quality.
Operational analytics dashboards provide a standard way of reporting on data quality efforts. With at-a-glance visibility, data teams can answer critical questions about the health and quality of their data, such as: How broad is our test coverage? Are users adopting the data quality solution in their daily work? Are data standards improving, with issues being discovered before there is a downstream impact? Is the data meeting the quality agreements? A dashboard is a compelling way to communicate the business value and impact of data quality, making it clear where data is moving the needle and where it is falling short.
Data engineers and analysts need the tools and means to deliver high-quality, reliable data products that meet the complex and evolving needs of their organisations. Engineers and analysts are often firefighting data issues when reports, dashboards, or machine learning models break, and downstream data consumers are left desperate for a solution. If engineers can test and monitor data as-code, they can efficiently set up and scale intelligent testing-as-code to check data quality across every data workload, from ingestion to transformation to production. Data analysts no longer need to rely on data engineers to implement data checks; they can do it themselves with a readable language to author checks, analyse incidents, and fix issues so that data quality remains trustworthy.
The rise of the data-domain-team approach is evolving toward decentralised data management, where data ownership is shared within a team of data engineers, product owners, scientists and analysts. In this environment of shared data ownership, teams can establish expectations for data quality with their data consumers. They can set up agreements that define what valid, timely, accurate and complete data looks like. And because everyone uses the same data reliability language, an agreements workflow easily aligns data producer and consumer expectations.
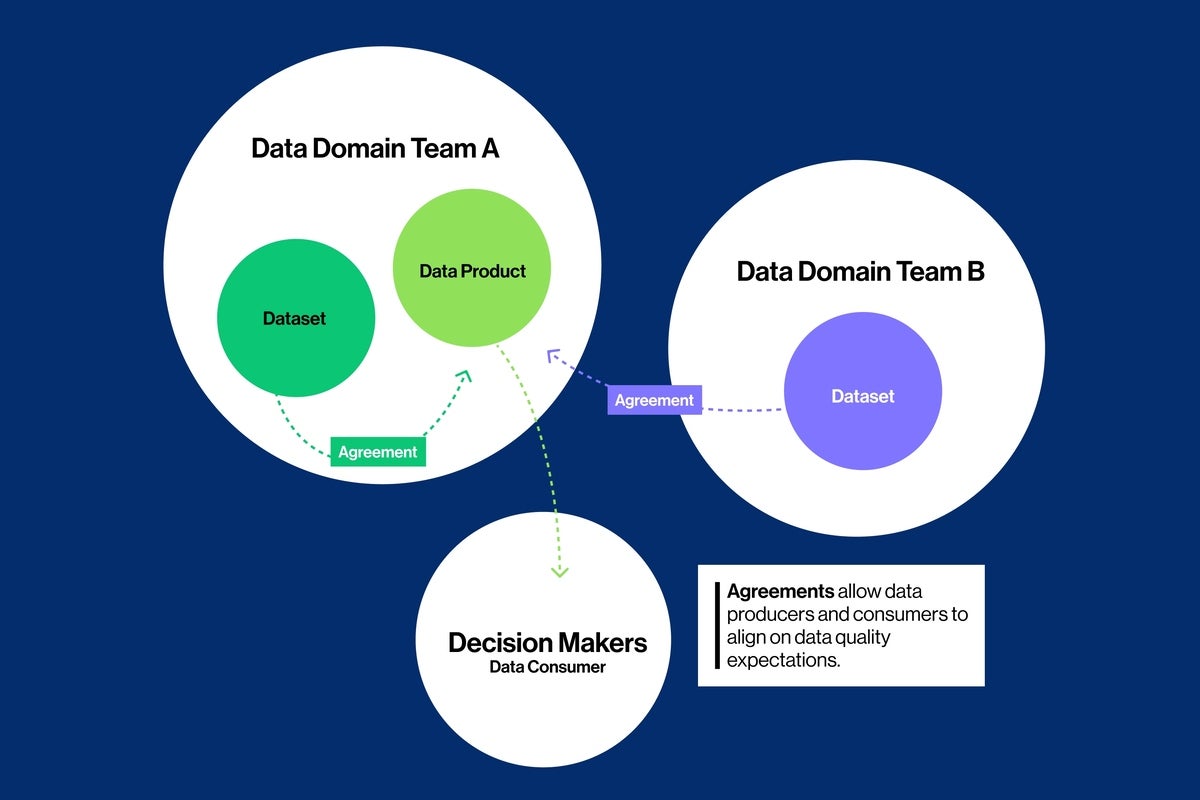
The full cost and impact of data downtime can disrupt a business and impact not just revenue, but productivity, regulatory or compliance mandates, customer retention, and employee satisfaction. Teams need a simplified process to detect, triage, diagnose and resolve data quality issues, using solid incident management best practices that include defined roles and responsibilities. Soda’s built-in incident management alleviates the pain of finding out too late that a data quality issue has had a downstream impact.
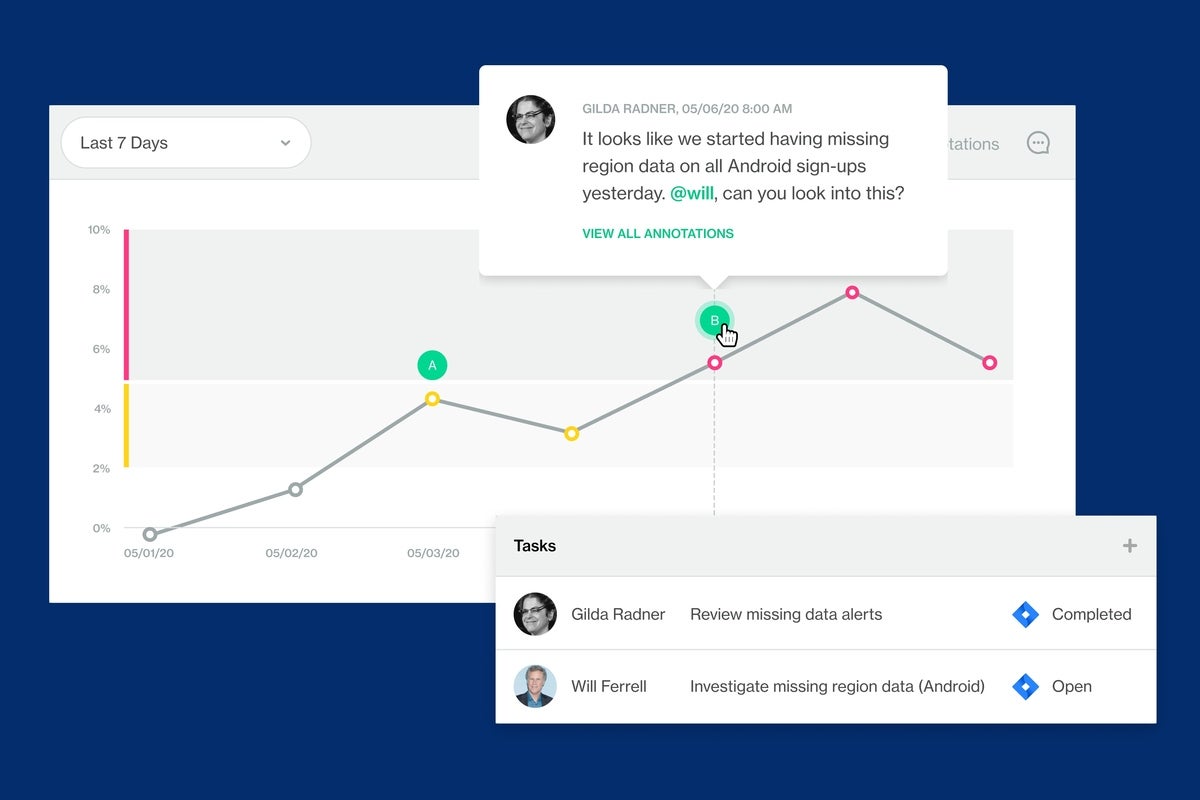
Organisations that rely on data-informed decisions need an integrated platform that creates complete transparency and provides data teams with the end-to-end observability and control in defining what good data looks like.
About Soda
Soda is the data reliability and quality platform that creates the observability data teams need to find, analyse and resolve data issues. Our open-source tools and cloud platform bring everyone closer to the data to confidently make data-informed decisions. Soda is one of the 2021 Gartner® Cool Vendors™ in Data Management, a recognition that our approach to solving the number one data management challenge faced by modern organisations – ensuring reliable, trusted data is available for all – is valid.
For more information, visit soda.io
Originally published on Business Reporter