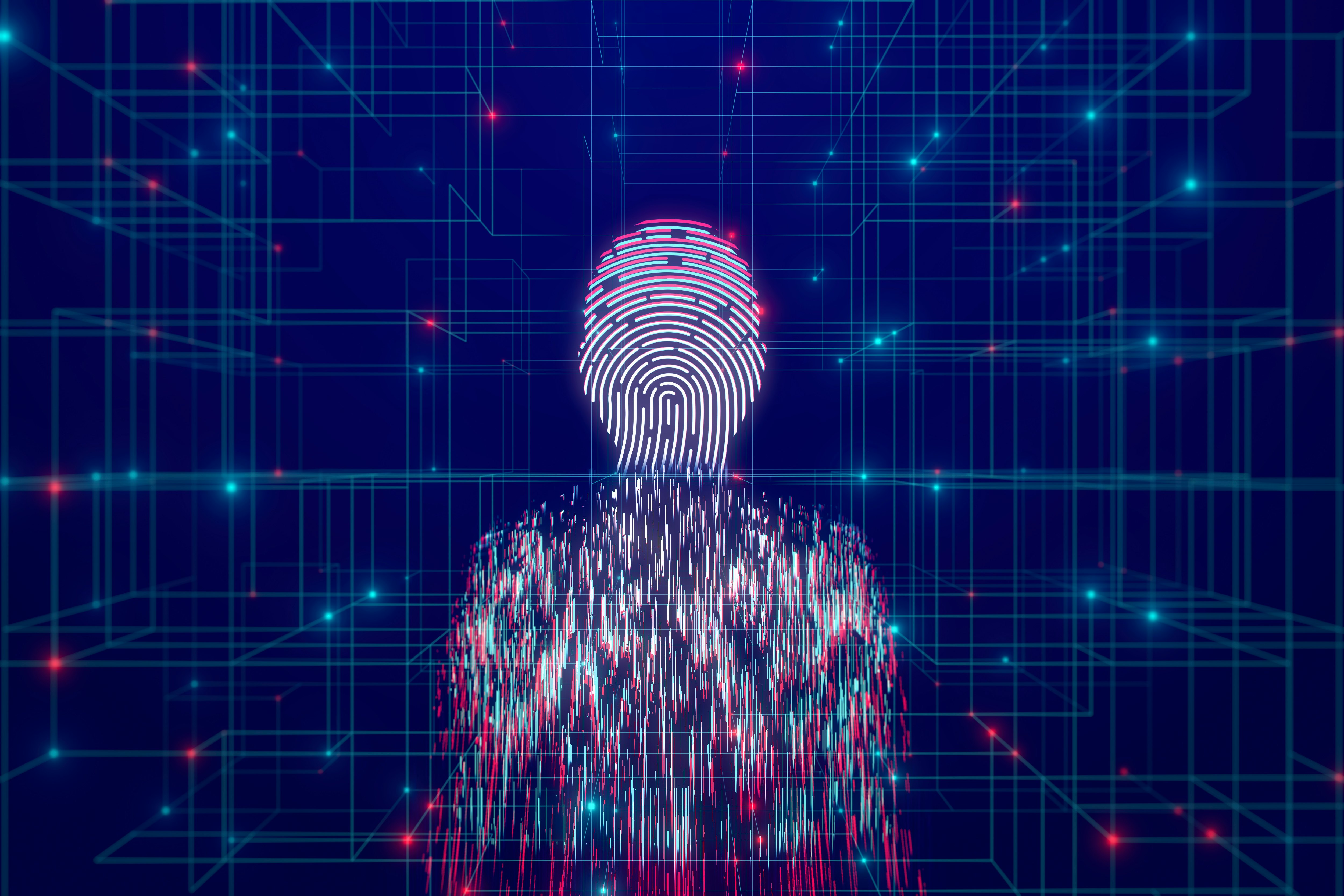
Type “teddy bears working on new AI research on the moon in the 1980s” into any of the recently released text-to-image artificial intelligence image generators, and after just a few seconds, the sophisticated software will produce an eerily pertinent image.
Seemingly bound by only your imagination, this latest trend in synthetic media has delighted many, inspired others, and struck fear in some.
Google, research firm OpenAI, and AI vendor Stability AI have each developed a text-to-image image generator powerful enough that some observers are questioning whether in the future people will be able to trust the photographic record.
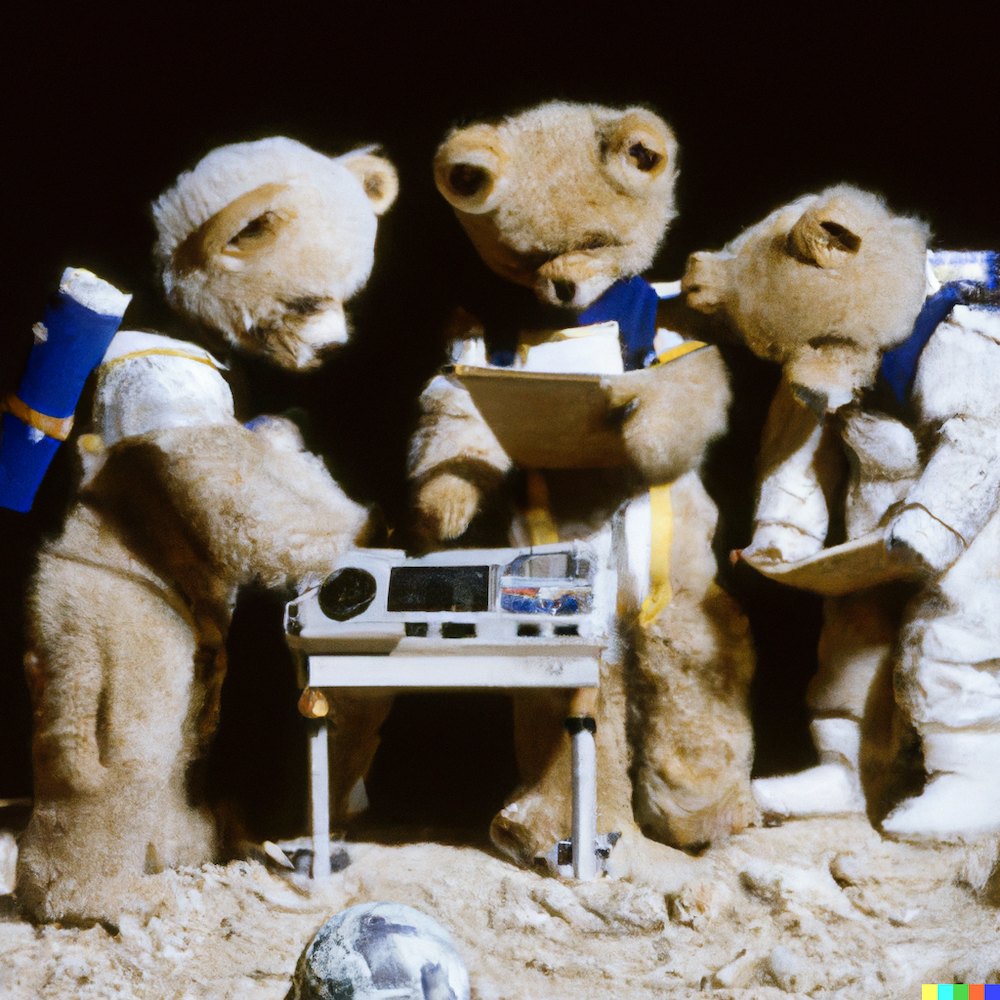
As a computer scientist who specializes in image forensics, I have been thinking a lot about this technology: what it is capable of, how each of the tools has been rolled out to the public, and what lessons can be learned as this technology continues its ballistic trajectory.
Adversarial approach
Although their digital precursor dates back to 1997, the first synthetic images splashed onto the scene just five years ago. In their original incarnation, so-called generative adversarial networks (GANs) were the most common technique for synthesizing images of people, cats, landscapes, and anything else.
A GAN consists of two main parts: a generator and a discriminator. Each is a type of large neural network, which is a set of interconnected processors roughly analogous to neurons.
Tasked with synthesizing an image of a person, the generator starts with a random assortment of pixels and passes this image to the discriminator, which determines if it can distinguish the generated image from real faces. If it can, the discriminator provides feedback to the generator, which modifies some pixels and tries again. These two systems are pitted against each other in an adversarial loop. Eventually, the discriminator is incapable of distinguishing the generated image from real images.
Text-to-image
Just as people were starting to grapple with the consequences of GAN-generated deepfakes — including videos that show someone doing or saying something they didn’t — a new player emerged on the scene: text-to-image deepfakes.
In this latest incarnation, a model is trained on a massive set of images, each captioned with a short text description. The model progressively corrupts each image until only visual noise remains and then trains a neural network to reverse this corruption. Repeating this process hundreds of millions of times, the model learns how to convert pure noise into a coherent image from any caption.
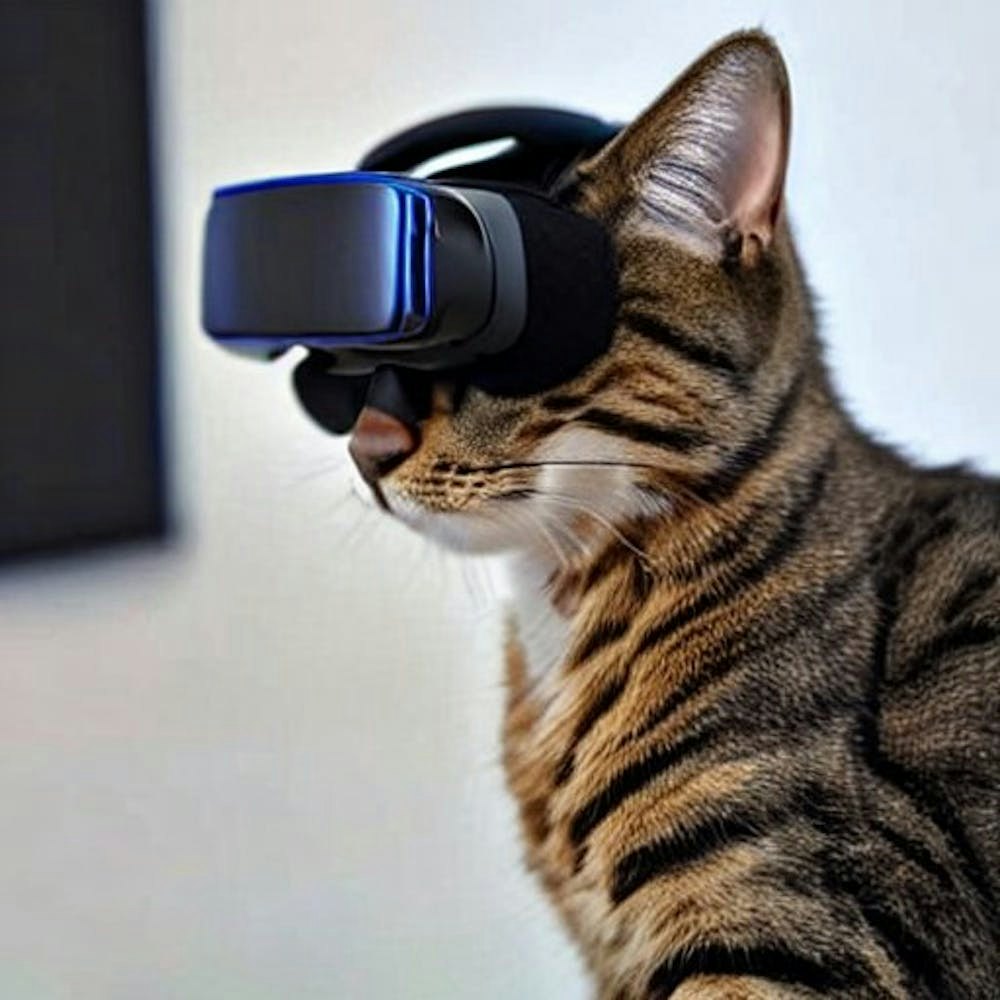
While GANs are only capable of creating an image of a general category, text-to-image synthesis engines are more powerful. They are capable of creating nearly any image, including images that include an interplay between people and objects with specific and complex interactions, for instance, “The president of the United States burning classified documents while sitting around a bonfire on the beach during sunset.”
OpenAI’s text-to-image image generator, DALL-E, took the internet by storm when it was unveiled on January 5, 2021. A beta version of the tool was made available to 1 million users on July 20, 2022. Users around the world have found seemingly endless ways to prompt DALL-E, yielding delightful, bizarre, and fantastical imagery.
A wide range of people, from computer scientists to legal scholars and regulators, however, have pondered the potential misuse of the technology. Deepfakes have already been used to create nonconsensual pornography, commit small- and large-scale fraud, and fuel disinformation campaigns. These even more powerful image generators could add jet fuel to these misuses.
Three image generators, three different approaches
Aware of the potential abuses, Google declined to release its text-to-image technology. OpenAI took a more open, and yet still cautious, approach when it initially released its technology to only a few thousand users (myself included). They also placed guardrails on allowable text prompts, including no nudity, hate, violence, or identifiable persons. Over time, OpenAI has expanded access, lowered some guardrails, and added more features, including the ability to semantically modify and edit real photographs.
Stability AI took yet a different approach, opting for a full release of their Stable Diffusion with no guardrails on what can be synthesized. In response to concerns of potential abuse, the company’s founder, Emad Mostaque, said, “Ultimately, it’s people’s responsibility as to whether they are ethical, moral, and legal in how they operate this technology.”
Nevertheless, the second version of Stable Diffusion removed the ability to render images of NSFW content and children because some users had created child abuse images. In responding to calls of censorship, Mostaque pointed out that because Stable Diffusion is open source, users are free to add these features back at their discretion.
The genie is out of the bottle
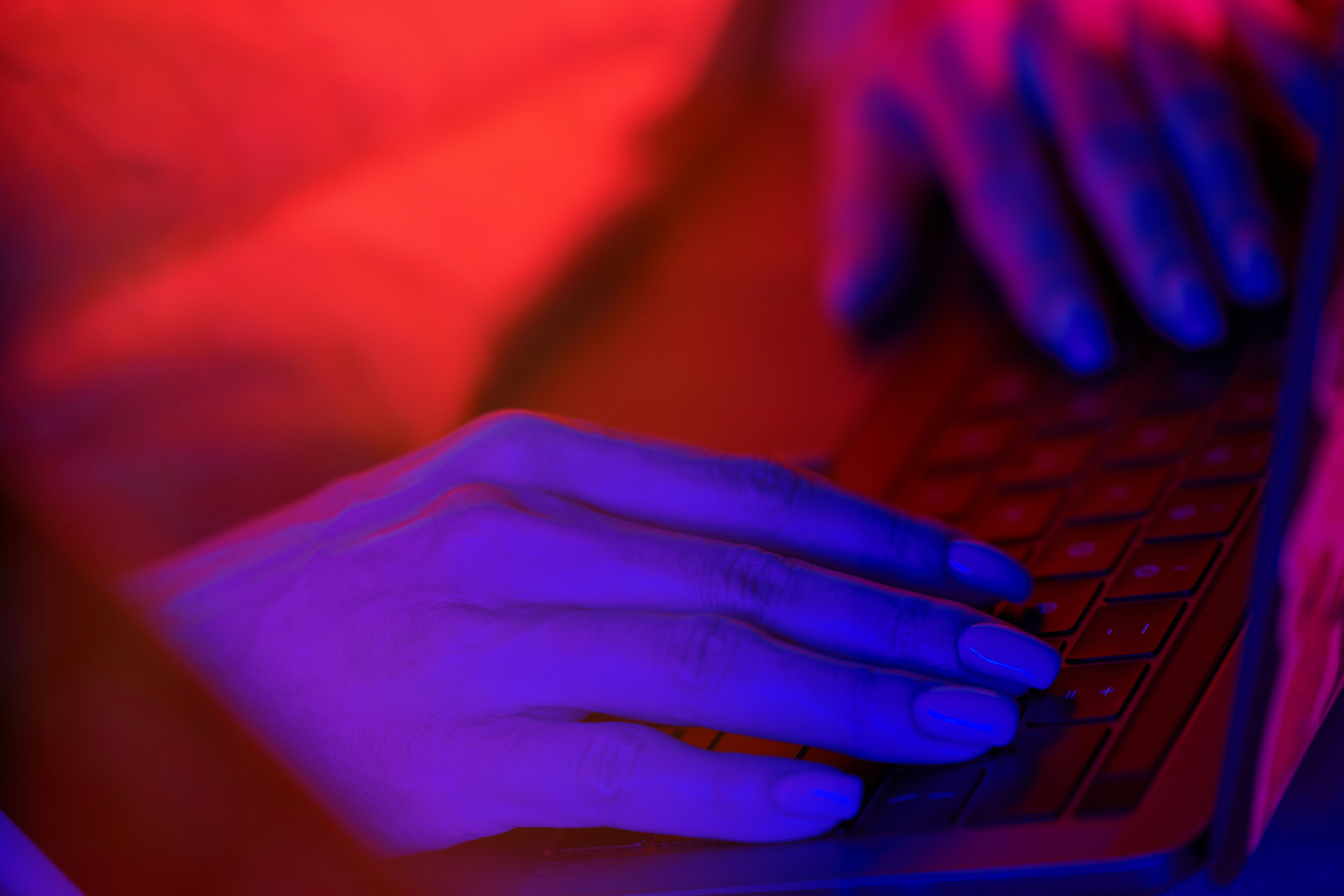
Regardless of what you think of Google’s or OpenAI’s approach, Stability AI made their decisions largely irrelevant. Shortly after Stability AI’s open-source announcement, OpenAI lowered its guardrails on generating images of recognizable people. When it comes to this type of shared technology, society is at the mercy of the lowest common denominator — in this case, Stability AI.
Stability AI boasts that its open approach wrestles powerful AI technology away from the few, placing it in the hands of the many. I suspect that few would be so quick to celebrate an infectious disease researcher publishing the formula for a deadly airborne virus created from kitchen ingredients while arguing that this information should be widely available.
Image synthesis does not, of course, pose the same direct threat, but the continued erosion of trust has serious consequences ranging from people’s confidence in election outcomes to how society responds to a pandemic and climate change.
Moving forward, I believe that technologists will need to consider both the upsides and downsides of their technologies and build mitigation strategies before predictable harms occur. Other researchers and I will have to continue to develop forensic techniques to distinguish real images from fakes. Regulators are going to have to start taking more seriously how these technologies are being weaponized against individuals, societies, and democracies.
And everyone is going to have to learn how to become more discerning and critical about how they consume information online.
The original article from The Conversation was updated to correct the name of the company Stability AI, which was misidentified.
This article was originally published on The Conversation by Hany Farid at the University of California, Berkeley. Read the original article here.