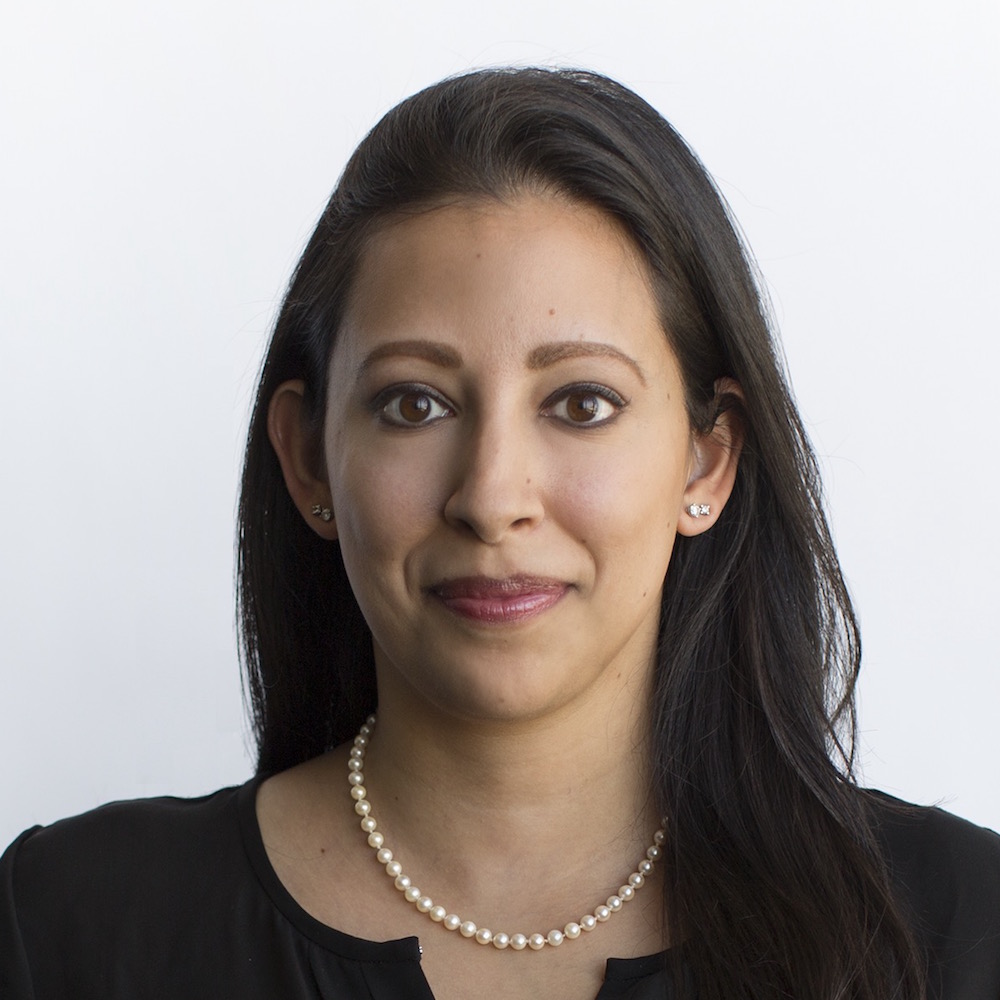
Generative AI has captured the imagination of the general public and broadcasters alike.
While an English lit high school student might look at ChatGPT as a shortcut to an essay comparing Beowulf and Grendel’s mom, broadcasters are eyeing generative AI to improve relevancy, workflows and ultimately their bottom lines.
Cambridge, Mass., -based Diesel Labs is helping them and other M&E enterprises leverage generative AI along with their vast stores of data to improve a wide variety of decisions about content—from marketing to which show or story concept stands the best chance of attracting an audience.
In this Q&A, company founder and CEO Anjali Midha discusses how movie and television studios are using generative AI, the role it might soon play in local television newsrooms, what generative AI brings to the process of data-driven media decisions and whether it might one-day take the human role out of making editorial decisions.
(An edited transcript.)
TVTech: What is the focus of Diesel Labs and how does generative AI in media plays into delivering on it?
Anjali Midha: At the core of it, what we're sitting on here is an understanding of how and when content becomes a part of our cultural zeitgeist.
For example, the “Barbie” movie, which we all know has done exceptionally well—beaten all the records—but that's actually a very interesting case study.
We saw “Barbie” go off the charts last year. You might recall that they dropped some stills from the movie. This was part of their social media campaign. I think it was of Margot Robbie and Ryan Gosling, in their beach gear while they were rollerblading down the boardwalk.
Right from that moment, we could see that that initial foray into starting to market the movie was already several times higher than anything we'd seen for any other film in the past couple of years. These signals are incredibly valuable. And they do tell you something even at that early stage—a year before the film was due to come out—that we were going to be looking at something that perhaps was going to perform fairly unusually.
It can go in either direction. We can also see things underperform in ways that are highly unusual. That gives us a different kind of insight of like, “OK, how do we want to actually spread these marketing dollars out as we approach the launch of a film?”
That's one way to think about the data we're sitting on. You can think of it as these are early attention signals that help us understand the appetite or the anticipation for content, whether it be a theatrical release, a streaming TV show or a streaming movie.
I think that idea can hold true whether you're operating at a national level, pushing out a global theatrical release or you're operating at a more local level.
TVT: How does this apply to local TV?
AM: We can see in our data what types of news stories—what articles—people are reading and circulating within their social networks. Are people right now talking about the tragic wildfires in Maui? Are they talking about the fact that the next “Game of Thrones” season is coming out in the next year and are getting excited for that.
When you think about it from the perspective of how you address what the audience is inclined to want to hear about, those are some of the techniques that we can reveal not only using the data we have, but also these new generative AI models that make it so incredibly quick.
Whereas before you needed to have an analyst go through the data with a fine-toothed comb to understand what was happening, this new technology honestly helps us to summarize incredibly vast amounts of information in a very short amount of time. It democratizes access to that information.
It is still expensive, but I imagine that the costs for those kinds of things will be coming down in the near term. We in the industry will appreciate the efficiency with which we can analyze this data, rather than needing five hours to study it as an analyst simply asks generative AI a question and get the answer back within seconds.
TVT: It would seem the garbage-in-garbage-out computing cliché must be factored into this process. In other words, the data has to be trustworthy, right?
AM: The underlying data that we're using for these models must be very, very good. You cannot use bad data, messy data or data that's otherwise not easily interpretable. You can appreciate how, if I'm giving you misinformation, you might come to the wrong conclusions.
So, one of the things that –at least here at Diesel Labs—we pay a lot of attention to and that we probably spend the vast majority of our day thinking about is how do we make sure this data is as clean, curated and perfected as possible so that we can make sure we're facilitating very, very good decisions, not only internally here, but for all of our partners that we work with as well.
TVT: That makes sense. How does that happen?
AM: Well, you know, that is a bit of our secret sauce. But that said, we do work with our partners very often to reveal what is the underlying data that we're using, and we can do this in an anonymized and aggregated fashion so that we're protecting both our clients and also the folks who are sharing their information in social and video spaces.
There are ways we can make sure that we are literally gut checking down to a very small specific metric on a specific platform. We do have mechanisms to do that, and it's an incredibly important part of our overall process.
TVT: How does generative AI play into this? Is it mostly a matter of enabling a regular person to ask a normally worded question to interrogate this data rather than having to be an expert using sophisticated programming?
AM: With generative AI tools or advanced modeling in general what you're doing is delivering to it information what we sometimes call training data, and you're teaching it. What did people say about “Barbie?” What did people say about “Game of Thrones?” What did people say about “Suits,” which right now is very popular on Netflix, even though it's a syndicated or licensed show?
Basically, what you're doing is combining everything everyone is saying about this content with all the amazing and rich data that these streaming platforms, movie studios or distributors have on their own content.
How many people are watching it? When did they stop watching it? What did they watch before? What did they watch after, when you combine all those things together, it is like a 1 + 1 = 3, because the more information you give it, the smarter the results will be when you start to interrogate the data using those advanced models.
TVT: TV broadcasters are deploying the ATSC 3.0 standard, which has over-the-top support and two-way connectivity. That can capture vastly more data about their audience’s viewing habits—what they are watching, when they are watching, how long they are watching, all data that on a granular scale wasn’t really available with the over-the-air-only broadcast model. Shouldn’t that level of data help drive better decisions whether those decisions are about which news story to produce or what show to make or air.
AM: Yes. For example, before the writers’ strike, we were getting questions like, “Hey, we have two options in front of us. We can have a sitcom featuring this person that has this sort of narrative arc, or we can do a game show like this with this host and this narrative arc.”
We're already able to put together those kinds of elements to create a view of what we think the audience might look like for the different options on the table. So, when you think about what we're doing now without that information you just described—essentially what I might call sort of more true measurement in terms of viewership—what more will we be able to do? The more information you have, the better your actual decision-making becomes.
TVT: Isn’t there a worry that generative AI used in this way might lock people into a box from an editorial perspective?
AM: At the end of the day, art is art. I want to make sure that everyone knows that none of this is meant to stifle creativity. I'm an avid viewer of content. I'm sure you are as well. Sometimes the most interesting, eye-catching and disruptive stories are those that come out of left field—those that are not easy to predict.
So, it's important to remember that's not what this is all about. It's not about stifling or replacing the creative process. It's about becoming better at making good decisions around how to best set up your content for success. Who to market it to if your initial attempts haven't been working very well. How to put a better wrapper around these things and make sure that audiences become aware of them?
This is about creating more tools for those of us who are in the media industry to get better around.
More information is available on the Diesel Labs website.