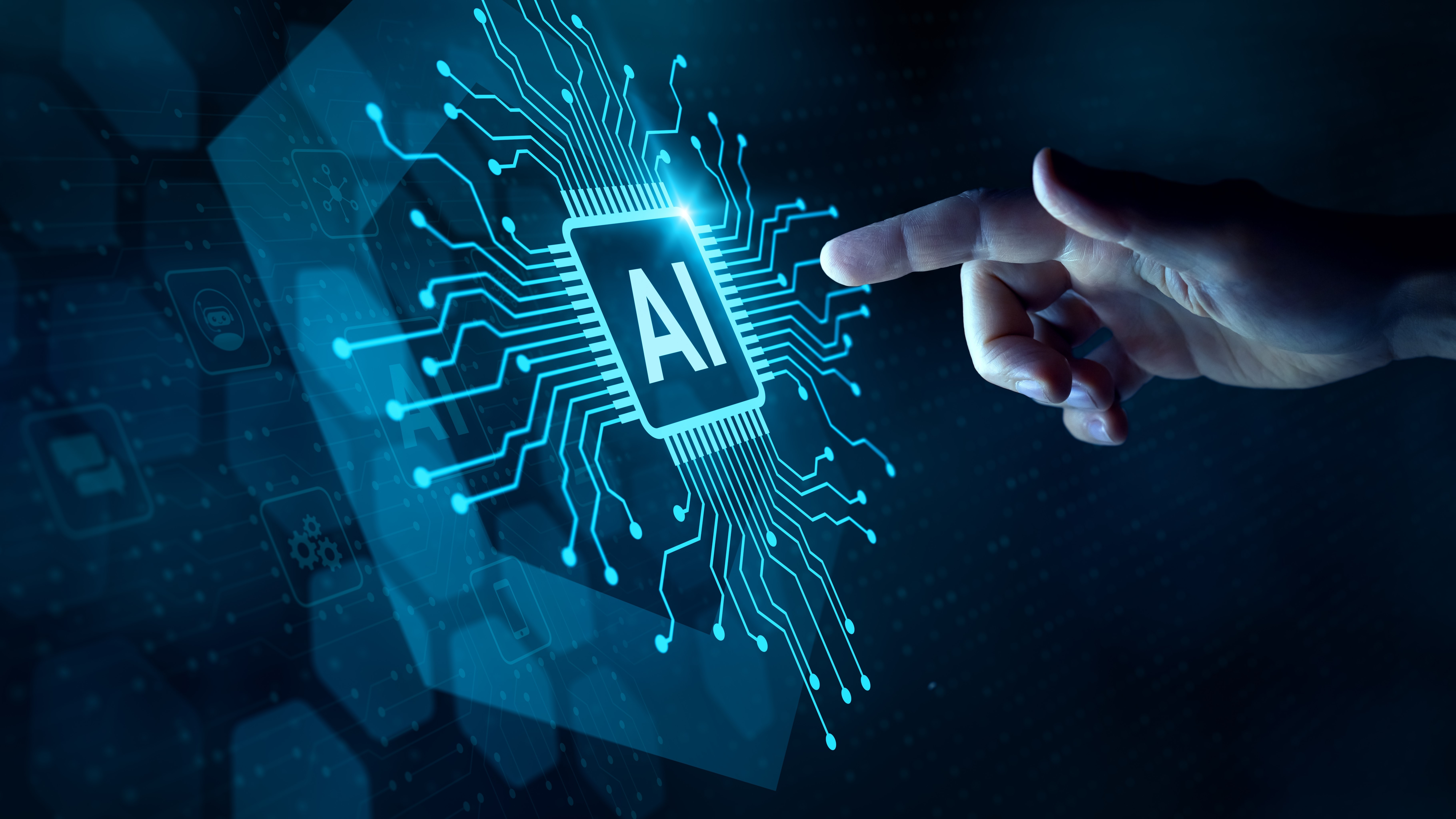
We’re almost at the point where AI can no longer be simply defined as an emerging technology. It’s here, and it’s booming. According to McKinsey, more than 70% of companies worldwide have either already deployed AI-based technology, or are seriously exploring its capabilities. That’s up from just 20% in 2017. In the past year alone we’ve seen incredible advancements in the AI space, particularly when it comes to generative AI and the use of Large Language Models (LLMs) to compute, make predictions, generate content, and analyze large sets of data in real-time for a plethora of applications.
Studies by the likes of HubSpot and IBM reveal that AI can save employees roughly 2.5 hours per day, and businesses can cut their overall costs by almost a third. An entire ecosystem of partners and complementary services is now being formed around AI, and its reach isn’t just confined to business. ChatGPT users can now have live conversations with AI; Meta’s Llama 3.2 update allows its AI assistant to read and comment on images, and Apple Intelligence is about to leave beta and allow users to do everything from generate code to create new graphics, all with technology they can carry in their pockets.
The technology is soaring, but like a busy road with too much traffic, there’s always a bottleneck. AI adoption is off the charts, business ambition is strong, but do we have the connectivity infrastructure to meet the low-latency needs of new AI applications? The traffic is coming; perhaps it’s time to turn our attention to the roads.
The invisible hurdle
Attitudes to AI are largely positive, but part of that is down to novelty. One metric that truly matters, return on investment (ROI), is still proving elusive for most business AI deployments. Three-quarters of enterprises have not moved beyond “baby steps”, that is one or two pilot projects (MIT Technology Review). And, although 50% of those surveyed expect to deploy AI at scale across all business functions within two years, they reported implementation challenges and bottlenecks – not due to capital, culture or lack of expertise, but in the infrastructure carrying their data.
Let’s be clear. These aren’t just teething troubles. Short-term hiccups at the start of the project are to be expected, but the underlying implementation challenges enterprises have highlighted point to a more fundamental, structural issue with the feasibility of AI roll-outs.
Underperforming AI is bad for business, and not just in the sense that it won’t realize its ROI, but because its insights and other outputs are limited. This happens when AI systems struggle to access and interpret data across the organization in real-time. The full potential of AI can only be realized when organizations have the right infrastructure in place to support its implementation. One critical aspect often overlooked is the importance of network interconnection. Here’s how this plays out within a typical enterprise.
Cloud and AI – a winning combination
Cloud computing plays a crucial role in AI implementation. Partly in response to the accelerating pace of data generation meaning that on-premise data storage is becoming unviable and partly because of the many accessibility benefits of storing raw and structured data in the cloud, businesses are increasingly migrating data lakes and warehouses to the cloud, enabling scalability and access to vast computing power. The AI Infrastructure Alliance showed that 38% of organizations had their AI infrastructure fully set up in the cloud, while 29% operate a hybrid environment.
These organizations rated the availability of cost and computing power as the number one challenge when scaling AI and their number one computing concern was latency (28%). A similar picture emerges across different territories, too. For example, a joint survey of European organizations by DE-CIX and IDC highlighted network performance and latency (22%) as the main concern, especially when AI use cases require real-time data.
The connectivity conundrum
Let’s consider how organizations typically use AI. Firstly, they need to train AI models, either first-time development or the periodic retraining that models need from time to time. For this, latency is not such a big issue, but high bandwidth connectivity is critical. In the cloud, it is best to use the cloud provider’s own connectivity solution (such as Microsoft ExpressRoute or AWS Direct Connect), accessible from a range of cloud exchanges, to avoid costly overheads for data egress. Secondly, they need AI to work in real-time, which is where latency does matter. Many use cases fall into this category, from customer services bots to product support where real-time interaction is desirable right through to where it is critical, such as autonomous vehicles, healthcare, and some financial services use cases. Here, the AI models need real-time access to data sources, as well as to the intended users of the insights and AI agents for different services and workloads.
In short, AI needs both high bandwidth and low latency network performance. Oh, and did we mention this all needs to be seamless?
The missing link is interconnection
Here is when the network performance bottleneck becomes apparent. Too many enterprises still rely on public internet or third-party transit for connecting data and AI systems. This creates considerable performance and security issues, with enterprises having little or no control over data pathways, network bandwidth and latency, and the security of critical company data in transit.
To control data flows, enterprises need to control how networks interconnect with each other. That’s why increasingly organizations are choosing network interconnection solutions, which provide secure, dedicated connections between on-premise systems and cloud-based AI services. By establishing direct, high-performance links, businesses ensure control over performance, security, and data routing. In practice, this network interconnection creates responsive, interoperable environments for cloud and multi-cloud scenarios, enabling low-latency access to AI-as-a-service offerings and real-time data analysis. It assures secure data exchange within partner ecosystems and improves the overall resilience of the cloud infrastructure environment, enabling business to roll out AI implementations at scale that deliver their intended ROI.
Enterprises need AI-ready infrastructure
AI offers organizations unprecedented opportunities to transform their operations and revenue generation. The awe-inspiring capabilities of AI models and data analytics tools naturally enough garner much attention, but organizations need to ensure that the underlying infrastructure supporting AI implementations is equally scalable and resilient for AI to live up to its potential. Investing in a robust interconnection strategy alongside cloud migration is critical for businesses to overcome AI connectivity bottlenecks and truly unlock its transformative potential.
Partnering with high-performance interconnection providers can help in the design of a secure, scalable network tailored to specific AI needs. The future of AI has already arrived; we just need to make sure we’re ready for it.
We've featured the best IT infrastructure management service.
This article was produced as part of TechRadarPro's Expert Insights channel where we feature the best and brightest minds in the technology industry today. The views expressed here are those of the author and are not necessarily those of TechRadarPro or Future plc. If you are interested in contributing find out more here: https://www.techradar.com/news/submit-your-story-to-techradar-pro