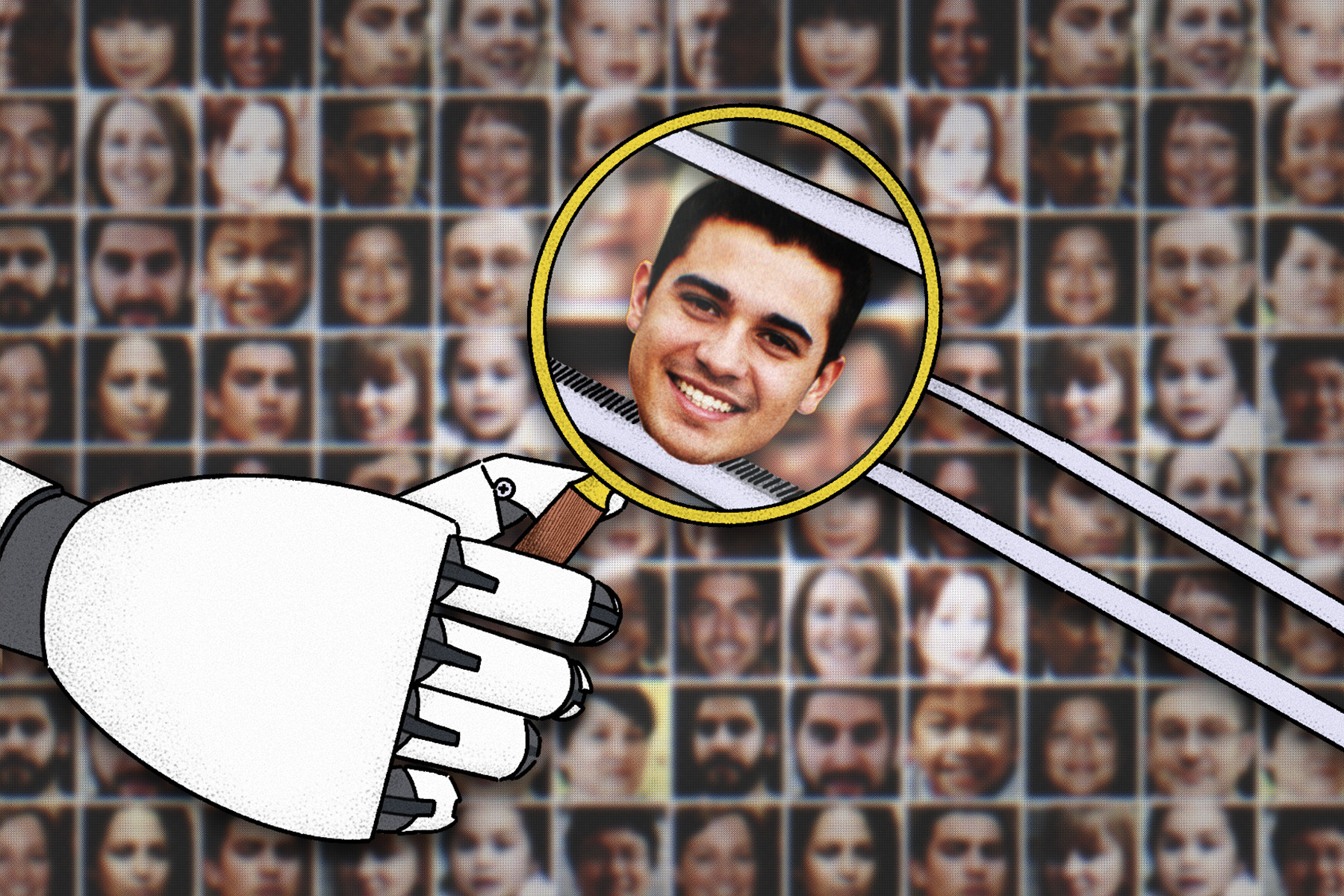
Nearly a decade ago, Ian Goodfellow, then a PhD candidate at Université de Montréal, was drinking with friends at the 3 Brasseurs in Montreal’s downtown when he conceived an idea that would change machine learning—and the world of disinformation—forever.
“I don’t want to be someone who goes around promoting alcohol for the purposes of science, but in this case, I do actually think that drinking helped a little bit,” said Goodfellow in his appearance on the Lex Fridman Podcast. If the idea came to him at lunchtime rather than over a beer in the evening, he added, he might have been able to talk himself out of it. Instead, he went home and started working on the project.
Goodfellow suspected that pitting two computer systems against each other—called generative adversarial networks, or GANs—would yield more realistic outputs than the deep-learning machines that existed at the time, which would often generate blurry images of people, usually with missing facial features, did. His early model was able to create numbers that looked hand drawn, human-like faces, and photos of animals that resembled something out of a pixelated Monet painting, but as the technology evolved, it became possible to create strikingly realistic forgeries using a much less involved process.
GANs use two competing algorithms that train themselves on a data set—for example, photos of faces. There’s a generator, which creates images based on the original data set, and a discriminator, which tries to identify the images that are fake. At first, both programs are weak, and the images they create aren’t quite right. But, as the algorithms duel with each other over time—engaging in a zero-sum game where the generator wins if it can dupe the discriminator and the discriminator wins if it can detect what the generator produced—the images become more and more convincing. This-person-does-not-exist.com is an example of how GANs can quickly produce an army of headshots of people who aren’t real. If machine learning’s goal is to give computers the ability to imitate intelligent human behaviour, deep-learning commentators have said Goodfellow gifted computers with an imagination.
While GANs ushered in a new era of machine learning with productive applications in medical imaging, predictive face aging, and creating visual art, they have also become a weapon in the arsenal of trolls and others looking to sew mistruths into the fabric of online discourse. For anyone accused of wrongdoing, manipulation tools can be what experts call a liar’s dividend. In the same way politicians have called unfavourable articles written about them “fake news,” being able to refute photos and videos by saying they are deepfakes gives people a get-out-of-jail-free card. In extreme instances, manipulated media can have life-and-death implications, like when a deepfake of Ukraine’s president, Volodymyr Zelenskyy, fictitiously asking his troops to lay down their arms and surrender emerged this year.
Other applications have been more isolated. In 2021, Jonas Bendiksen, a Norwegian photographer, entered his Book of Veles in France’s prestigious Visa pour l’image photojournalism festival. His photographs, depicting life in the North Macedonian town that served as a production site for misinformation and disinformation during the 2016 American presidential election, contained computer-generated people and animals, and no one noticed. If Bendiksen’s images were able to trick experts who have dedicated most of their lives to photography, what chance do the rest of us have?
As artificial intelligence tools become more sophisticated, manipulated media, including deepfakes, have become more challenging to detect—especially amid the sheer volume of them surfacing online. According to a 2021 report by the World Economic Forum, the number of deepfake videos has increased by an estimated 900 percent annually, and they’ve reached a point where people like Bendiksen are able to teach themselves how to make them just by watching YouTube videos.
But now, in an attempt to ease the disinformation crisis, researchers are finding new ways to help audiences distinguish the real from the fake.
For three months between 2019 and 2020, Facebook (now Meta) cohosted the Deepfake Detection Challenge, asking participants to automate the process of determining if a photo has been manipulated with artificial intelligence. The competition drew 2,114 participants and awarded $1 million (US) in prizes to the entries with the most-successful algorithms. But, even with some of the sharpest minds in artificial intelligence working with ample motivation, the best program was able to detect deepfakes only 65 percent of the time.
Currently, most artificial intelligence–based detection programs search for “visual artifacts”—imperfections like lighting inconsistencies, odd shadow placement, and geometric disagreements—to identify where an image could be manipulated. But, due to the evolving nature of artificial intelligence, these programs can quickly learn how to cover their own tracks—like when a 2018 University at Albany study found that fake people tend to blink either more or less than real people in videos and, one year later, researchers in South Korea noted that deepfakes were developing more realistic blinking patterns. Similar fixes have been made with glasses and teeth, both of which never used to look quite right in AI-generated photos. As experts highlight these errors, they are also inadvertently providing deepfake creators a step-by-step guide to creating more deceptive images.
Deepfakes aren’t able to produce images of perfect, fully synthetic humans just yet, so detection tools remain effective. But Andy Parsons, the senior director of the Content Authenticity Initiative at Adobe, who works to develop tools that help combat the rise of disinformation and misinformation, says it won’t be a viable solution forever. “If we zoom out, what does the five- or ten-year horizon look like for detection? I think it’s a losing battle,” he says. “For lack of a better term, the bad guys are probably going to win that one.”
While deepfakes are a rising threat, Jane Lytvynenko, who works on the Media Manipulation Casebook, a resource aimed at journalists and researchers that documents misinformation and disinformation, says the bigger concern is “cheap fakes,” which are photos and video edited without artificial intelligence.
Before joining the Technology and Social Change Project at the Harvard Kennedy School’s Shorenstein Center, Lytvynenko made a name for herself by covering misinformation and disinformation at BuzzFeed News. According to Lytvynenko, aptly named cheap fakes—which use cut and paste, slowed-down audio, and spliced video—provide deceivers an affordable and effective way to create manipulated media. In a video titled “IS SHE DRUNK?!?! Nancy Pelosi Fumbles Words, Struggles Through Press Conference,” posted by a YouTube channel known for touting right-wing conspiracies, Pelosi is shown seemingly slurring her words. The video deploys old-school methods of trickery by slowing down the speed to give the impression her speech was impaired. Despite the fact that the video was later deemed fake, it was still shared widely and remains up on the platform. “People get misinformed by simpler tactics than deepfakes, so there is not much of an incentive right now to deploy complex approaches,” said Lytvynenko.
But a new solution, called content provenance, could offer a better way to adapt to the evolving world of misinformation. With a name borrowed from the art world, this initiative seeks to establish a chain of provenance that documents what has happened to an image throughout the entirety of its digital life, including who shot it, when it was taken, and what edits have been made to it. Rather than work backwards to see if an image has been tampered with, software tries to guarantee an image’s authenticity from its creation. This data is then packaged and, when the image is published online, shared in an info box alongside the photo.
Adobe has begun a push for this type of verification through its Content Authenticity Initiative, first announced in 2019. The program, which has already been rolled out in Adobe Photoshop, offers creators a way to track changes made to a photo and organizations—like Twitter and the New York Times—a way to be more transparent with their audiences. As an opt-in tool, content authenticity won’t pull back the curtain on deepfakes but instead gives credibility to nonmanipulated media in the same way that users can be verified on social media. Since Adobe’s announcement, developing partnerships with digital platforms and media organizations and implementing content authenticity on their own stock images have been the first steps to having the program gain traction.
According to Parsons, deepfake detection and content provenance are complementary authenticators—the former being reactive and the latter a proactive measure. The goal is to not only offer more transparency online but also to encourage audiences to think more critically about the media they’re consuming.
“At the end of the day, you can trust the photography and the math, but in order to imbue media with the trust that they’re looking for, you have to trust a person or an organization,” said Parsons. “Now, I think there’s a greater need than ever to continue to trust those organizations, but also, as a consumer and a fact checker, look at the provenance and understand where it came from and how it might have been manipulated or processed along the way.”
While the onus may be shared by consumers and creators for the time being, it might not be that way forever. In only eight years, a conversation at a Montreal bar transformed the disinformation sphere at breakneck speed. It’s entirely possible that a technology with the ability to curb media manipulation could disrupt the detection scene with the same vigour.
Correction, August 16, 2022: An earlier version of this article stated that Adobe’s Content Authenticity Initiative was rolled out across Creative Cloud applications. In fact, it has only been rolled out in Adobe Photoshop. The Walrus regrets the error.