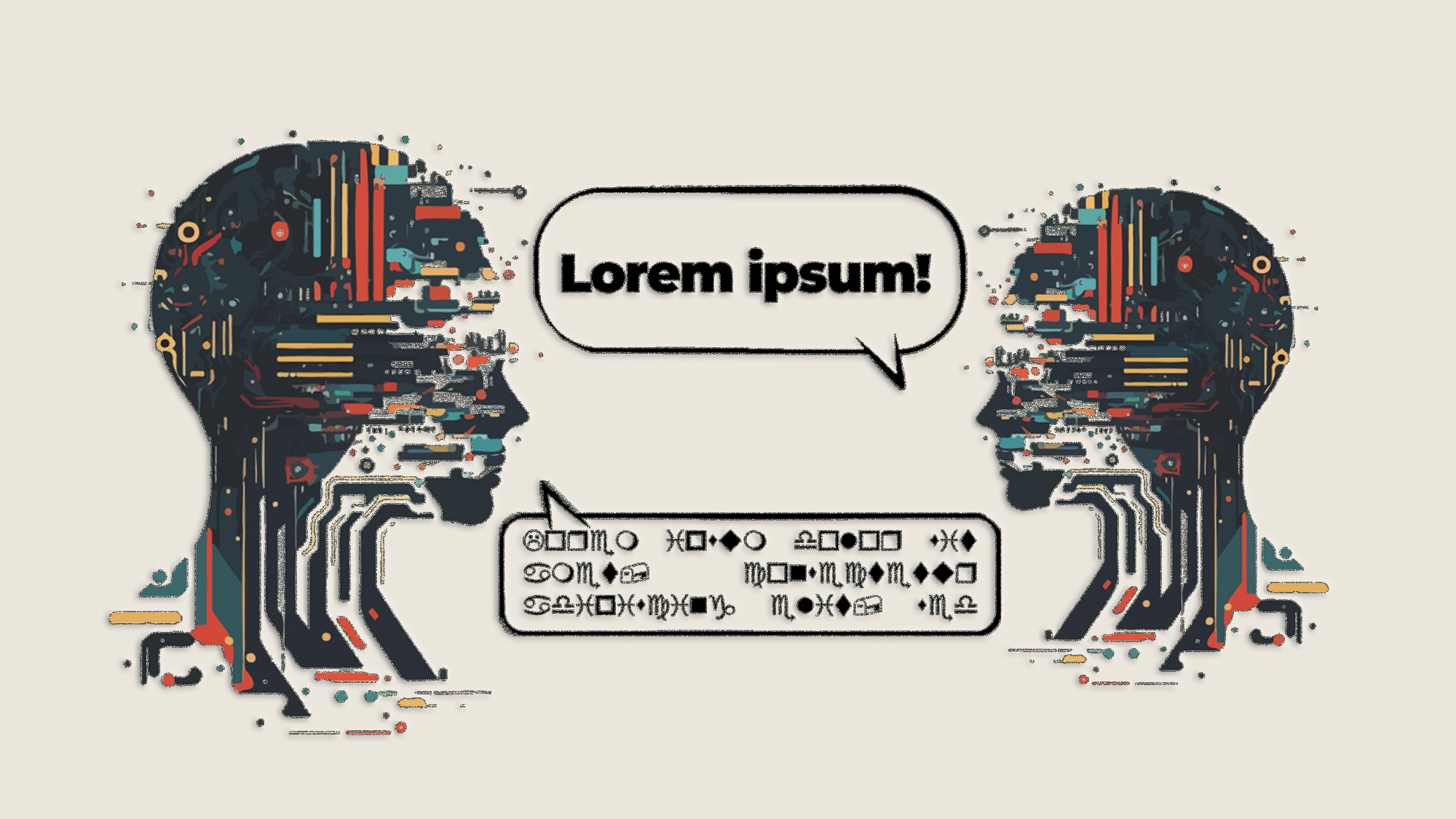
OpenAI ChatGPT, Google Bard, and Microsoft Bing AI are incredibly popular for their ability to generate a large volume of text quickly and can be convincingly human, but AI "hallucination", also known as making stuff up, is a major problem with these chatbots. Unfortunately, experts warn, this will probably always be the case.
A new report from the Associated Press highlights that the problem with Large Language Model (LLM) confabulation might not be as easily fixed as many tech founders and AI proponents claim, at least according to University of Washington (UW) professor Emily Bender, a linguistics professor at UW's Computational Linguistics Laboratory.
“This isn’t fixable,” Bender said. “It’s inherent in the mismatch between the technology and the proposed use cases.”
In some instances, the making-stuff-up problem is actually a benefit, according to Jasper AI president, Shane Orlick.
"Hallucinations are actually an added bonus,” Orlick said. “We have customers all the time that tell us how it came up with ideas—how Jasper created takes on stories or angles that they would have never thought of themselves.”
Similarly, AI hallucinations are a huge draw for AI image generation, where models like Dall-E and Midjourney can produce striking images as a result.
For text generation though, the problem of hallucinations remains a real issue, especially when it comes to news reporting where accuracy is vital.
"[LLMs] are designed to make things up. That’s all they do,” Bender said. “But since they only ever make things up, when the text they have extruded happens to be interpretable as something we deem correct, that is by chance,” Bender said. “Even if they can be tuned to be right more of the time, they will still have failure modes—and likely the failures will be in the cases where it’s harder for a person reading the text to notice, because they are more obscure.”
Unfortunately, when all you have is a hammer, the whole world can look like a nail
LLMs are powerful tools that can do remarkable things, but companies and the tech industry must understand that just because something is powerful doesn't mean it's a good tool to use.
A jackhammer is the right tool for the job of breaking up a sidewalk and asphalt, but you wouldn't bring one onto an archaeological dig site. Similarly, bringing an AI chatbot into reputable news organizations and pitching these tools as a time-saving innovation for journalists is a fundamental misunderstanding of how we use language to communicate important information. Just ask the recently sanctioned lawyers who got caught out using fabricated case law produced by an AI chatbot.
As Bender noted, a LLM is built from the ground up to predict the next word in a sequence based on the prompt you give it. Every word in its training data has been given a weight or a percentage that it will follow any given word in a given context. What those words don't have associated with them is actual meaning or important context to go with them to ensure that the output is accurate. These large language models are magnificent mimics that have no idea what they are actually saying, and treating them as anything else is bound to get you into trouble.
This weakness is baked into the LLM itself, and while "hallucinations" (clever technobabble designed to cover for the fact that these AI models simply produce false information purported to be factual) might be diminished in future iterations, they can't be permanently fixed, so there is always the risk of failure.