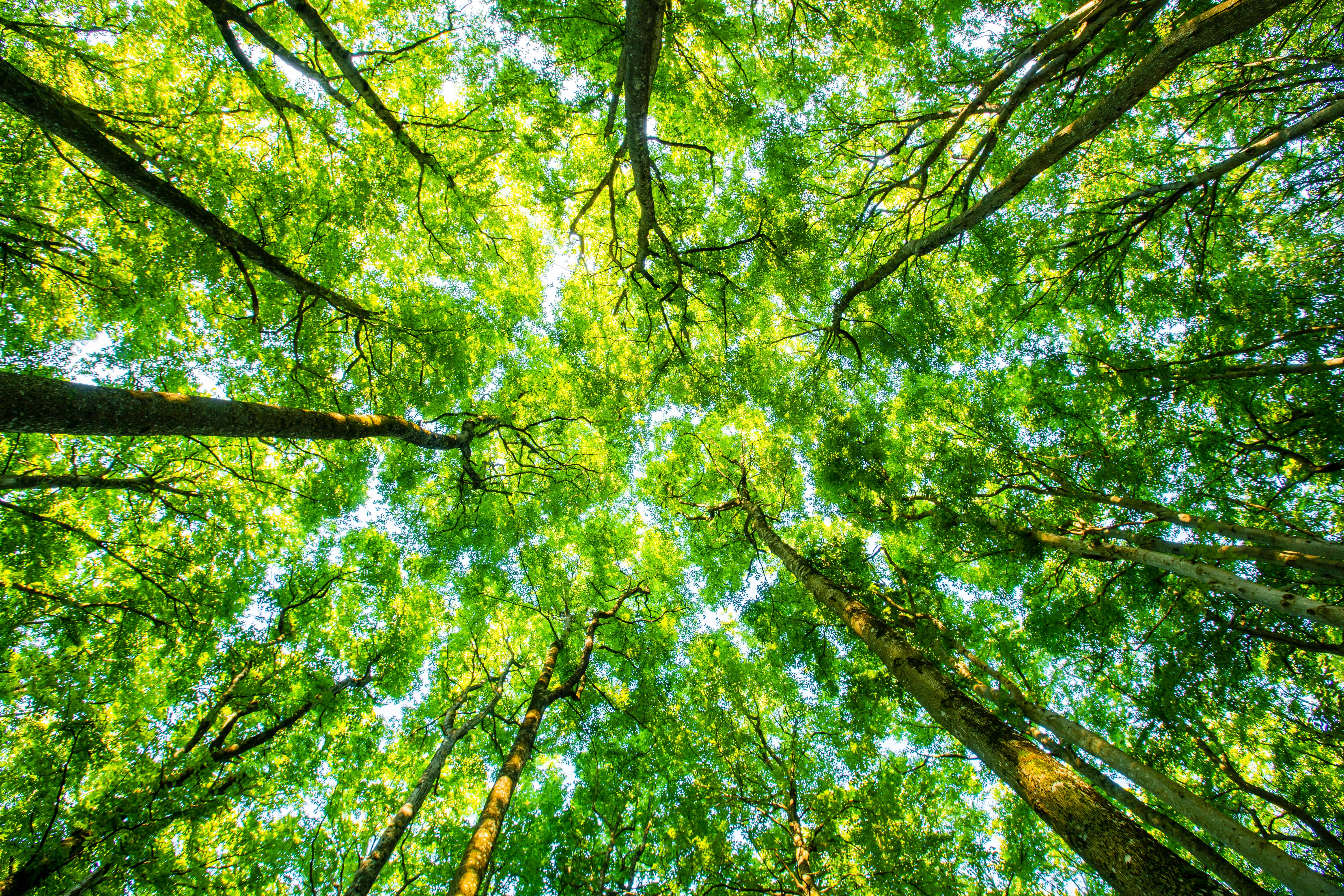
AI has dominated the headlines lately — and not necessarily in a good way.
From AI bot ChatGPT helping college students plagiarize essays to tools like Dall-E potentially spreading misinformation, conversation about the future of AI seems increasingly pessimistic.
But some scientists are still banking on AI technology to help save our planet. Researchers deployed an AI technique known as deep learning to map all the individual trees in Rwanda — and their methods could potentially help us conserve trees all around the world.
“Our approach provides a possibility to map and monitor the impact of landscape restoration at the level of individual trees,” Maurice Mugabowindekwe and Ankit Kariryaa tell Inverse. The two researchers from the University of Copenhagen are co-authors of the new study.
Their findings were published earlier this month in the journal Nature Climate Change.
How they did it — Using aerial satellite images of Rwanda that were taken in 2008, the scientists successfully mapped 355 million overstory trees, whose treetops are visible in the images. These trees spanned different landscapes, from savannahs to natural forests to urban tree ecosystems.
The scientists were able to document each individual tree using an AI machine learning technique called deep learning.
“Deep learning is a subset of machine learning methods for training artificial neural networks,” the study authors write.
Machine learning essentially trains computers on large datasets, helping them learn through a series of interconnected nodes in neural networks, which mimic how neurons in the human brain work.
“The trained deep learning model is then used to map all individual trees in the country,” says Mugabowindekwe and Kariryaa.
Finally, the scientists also used field data and information from the Rwanda National Forest Inventory to estimate the total amount of carbon stored in the different tree types, known as carbon stock.
What they found — Their country-wide deep learning experiment yielded some surprising results.
They found that the majority of trees mapped weren’t in natural forests but in other ecosystems. Approximately 72 percent of trees mapped were found in farmlands and savannas, while 17 percent were located in plantations — tree farms containing only one or a few tree species.
They also found that although natural forests only made up 11 percent of the total mapped trees, they accounted for a majority of the carbon stored in trees in Rwanda. About 51 percent of the nation’s carbon stock came from these natural forests.
The researchers say that based on this evidence focusing on conserving and restoring natural forests is still vital to tackle climate change, but we shouldn’t forget about trees outside of forests either. The researchers stress the “relevance of all trees for conservation and protection efforts.”
Why it matters — Trees store or “sequester” large amounts of carbon, preventing their release into the atmosphere. Therefore, trees are essential for combating global warming, which is caused primarily by the release of greenhouse gases like carbon dioxide.
“In order to mitigate climate change and fight the ongoing biodiversity loss, countries and jurisdictions across the world are pledging to restore landscapes by planting a certain amount of trees,” the study authors explain.
But previous tree mapping methods relied upon expensive data collection, which makes tree monitoring difficult for lower-income countries with fewer resources. Without reliable monitoring tools, researchers say there is a “high risk” that countries will be unable to accurately track their restoration efforts.
“This could make the conservation and sustainable management of both forests and other tree-dominated landscapes very challenging,” Mugabowindekwe and Kariryaa say.
Their deep learning, which researchers say maps individual trees with “unprecedented accuracy,” could be a game-changer for tree monitoring and conservation on a wide scale at a lower cost. According to their data, the deep learning method had a 96.2 percent “per pixel” accuracy in mapping the trees.
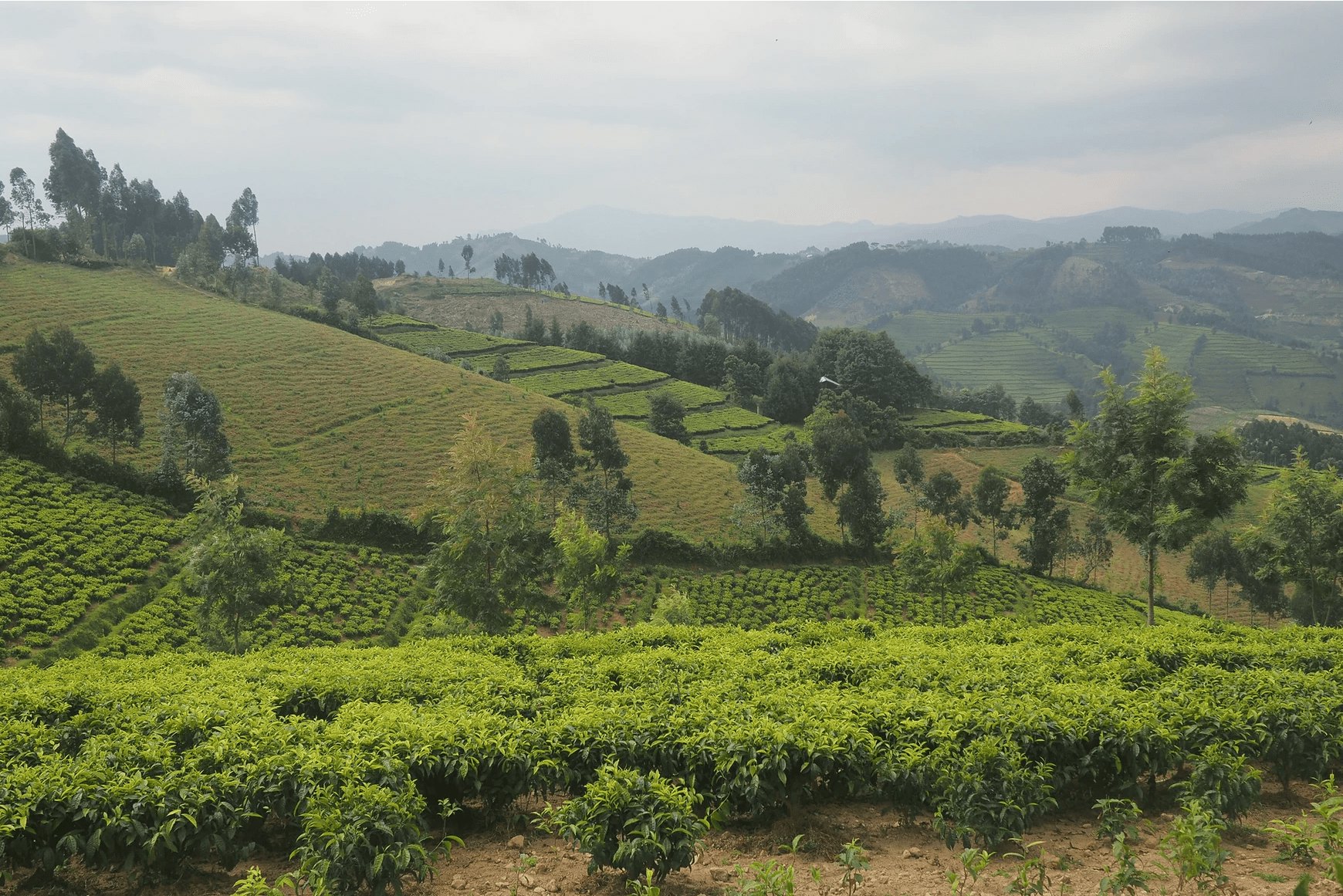
The scientists were also able to take their unique tree-mapping methods beyond Rwanda to countries like Kenya and Burundi and even venturing as far as France and California. With their cutting-edge method, it’s possible we could get close to mapping individual trees all around the world — even in terrains that are difficult to access.
“This demonstrates that our models can be used to accurately map individual trees across challenging ecosystems,” write the researchers.
What’s next — Rwanda has been at the forefront of conservation efforts. In 2019, the country achieved its goal of covering at least 30 percent of the country with forests. Now, it’s committed to restoring 80 percent of degraded land by 2020 under the Bonn Challenge.
It’s also a good place to analyze tree mapping methods, as there’s a varied landscape across the region ranging from rainforests to savannahs.
The researchers at the University of Copenhagen are currently working with the Rwandan government to track changes to the tree landscape between 2008 and 2019 to gain a better picture of the restoration needed.
Mugabowindekwe and Kariryaa’s deep learning tools will undoubtedly help with big ecosystem restoration goals in Rwanda — and possibly in countries all around the world.
“They offer a new possibility to automate the tedious and time-consuming but extremely important task of mapping individual trees at a country, continental, or even global scale,” conclude the scientists.