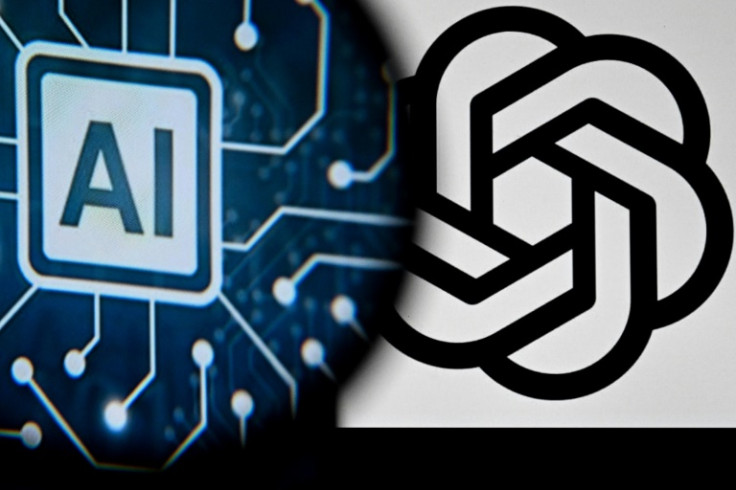
Artificial Intelligence (AI) systems, such as ChatGPT, have made remarkable progress, yet they still need human assistance for enhancement and accuracy. According to a new study published by Nature, AI systems remain heavily reliant on large datasets curated and labelled by humans to learn and generate their responses.
Despite significant advancements in large language models (LLMs), one major limitation is their inability to train on their content to improve intelligence. Training an AI involves feeding it vast amounts of data to help it understand context, language patterns, and various nuances before fine-tuning based on performance.
In Nature's groundbreaking study, researchers experimented by providing LLMs with AI-generated text. This approach led to models needing to remember the less frequently mentioned information in their datasets, causing their outputs to become more homogeneous and eventually nonsensical. "The message is, we have to be very careful about what ends up in our training data," says co-author Zakhar Shumaylov, an AI researcher at the University of Cambridge, UK. Otherwise, "things will always, provably, go wrong," he added.
Limitations of AI
The team used mathematical analysis to show that the problem of model collapse is likely to be universal, affecting all sizes of language models that use uncurated data, simple image generators, and other types of AI. "This is a concern when trying to make AI models that represent all groups fairly because low-probability events often relate to marginalised groups," says study co-author Ilia Shumailov, who worked on the project at the University of Oxford, UK.
Language models build associations between tokens—words or word parts—in large volumes of text, often scraped from the Internet. They generate text by predicting the statistically most probable next word based on these learned patterns. In the study, the researchers started by using an LLM to create Wikipedia-like entries, then trained new iterations of the model on text produced by its predecessor.
As AI-generated information—synthetic data—polluted the training set, the model's outputs became gibberish. For instance, the ninth iteration of the model completed a Wikipedia-style article about English church towers with a treatise on the many colours of jackrabbit tails. The team expected to see errors but were surprised by how quickly "things went wrong."
"Collapse happens because each model is necessarily sampled only from the data on which it is trained. This means that infrequent words in the original data are less likely to be reproduced, and the probability of common ones being regurgitated is boosted," explains Shumaylov. "Complete collapse eventually occurs because each model learns not from reality but from the previous model's prediction of reality, with errors amplified in each iteration."
Future Collapse?
The study also highlights a more significant issue. As synthetic data builds up on the web, the scaling laws suggesting that models should improve with more data may break down because training data will lose the richness and variety of human-generated content. Hany Farid, a computer scientist at the University of California, Berkeley, compares this problem to "inbreeding within a species." Farid states, "If a species inbreeds with its own offspring and doesn't diversify its gene pool, it can lead to a collapse of the species."
Farid's work has demonstrated similar effects in image models, producing eerie distortions of reality. The solution? Developers might need to find ways, such as watermarking, to keep AI-generated data separate from accurate data, which would require unprecedented coordination by big-tech firms, suggests Shumailov.
Even when Shumailov and his team fine-tuned each model on 10 per cent real data alongside synthetic data, the collapse occurred more slowly. Society might need to find incentives for human creators to continue producing content, and filtering may become essential. For example, humans could curate AI-generated text before it goes back into the data pool.