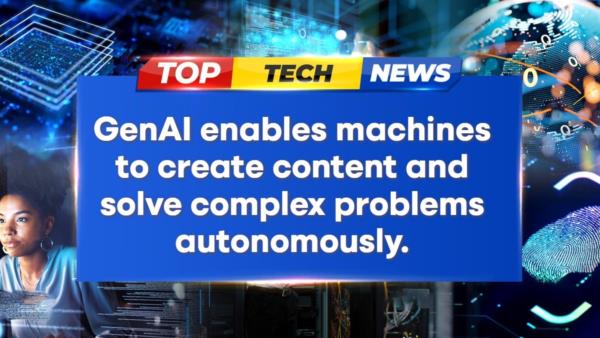
In today's rapidly evolving digital landscape, the emergence of generative artificial intelligence (GenAI) has revolutionized various industries. With its ability to create content, mimic human intelligence, and solve complex problems autonomously, GenAI has emerged as a game-changer. To fully harness the potential of GenAI, organizations must embark on a journey of data preparation and automation, ensuring that their data is governed, labeled, and compliant with ethical and regulatory standards.
As the adoption of AI continues to grow, there has been a shift from traditional analytics to AI-based solutions. This shift is impacting traditional approaches to data cataloging, governance, privacy, security, quality, bias, and compliance. Organizations now prioritize unstructured data over structured data since it forms the foundation of AI. Understanding and managing unstructured data have become more important than ever, but it presents its own set of challenges due to its volume, velocity, and variety across an organization's environment.
Managing today's data landscape requires a shift towards automation and AI. The growing data volume and velocity of change outpace traditional manual processes, making it necessary to leverage automation to keep up. Many organizations are beginning to adapt and innovate at the speed of AI by controlling data sharing, auditing data usage, building policies, and enforcing them to ensure compliance.
When it comes to AI, training data plays a pivotal role. The effectiveness and reliability of GenAI depend on the accuracy, relevance, and safety of its training data. Data used for AI training must be up to date, non-redundant, safe to use for its intended purpose, and validated to avoid confidential or sensitive information leakage. Given the different forms data can take, from structured databases to unstructured content like files, chats, emails, and images, cataloging and managing this diverse data landscape is critical. Additionally, organizations must handle sensitive and dark data in compliance with privacy regulations, security frameworks, and ethical considerations.
To stay ahead in the AI era, organizations must adopt an inventory-driven approach that accurately identifies structured, semi-structured, and unstructured data across both cloud and on-premise environments. This real-time understanding of the data landscape is crucial for the success of GenAI initiatives. By scanning, tagging, and classifying data based on context and type, organizations can create policies that automatically trigger alerts if data is moved, deleted, or in violation of established business policies.
Identifying and mitigating risk in unstructured data is paramount in the age of data breaches and cyber threats. Toxic combinations of sensitive information within unstructured data can have severe consequences if incorporated into GenAI models. Organizations need to actively detect and surface these toxic combinations, preventing them from contaminating AI training data. Regular audits and reports help communicate risk and prompt actionable recommendations to reduce potential risks.
Data privacy regulations, security frameworks, and AI ethics guidelines are constantly evolving, requiring organizations to stay updated and compliant. Implementing policies based on data type and regulation, assessing data against the latest standards, and mitigating compliance risks are crucial steps in maintaining ethical and responsible AI practices. By doing so, organizations can detect compliance violations promptly and align data practices with ever-changing ethical and regulatory requirements.
Embarking on the GenAI journey requires organizations to put controls in place based on data type and manage data specifically for AI purposes. Ensuring data is prepared to be AI-safe helps reduce the risk of leaks and breaches. Automating access governance and control mechanisms allows for effective management of insider risks, going beyond user identification to automatically identify data accessed by different models. It is also essential to understand the data consumed by various AI models for auditing purposes and to effectively manage data privacy, compliance, and security across the entire data landscape.
By following these steps, organizations can unlock the full potential of AI innovation while minimizing risk, meeting ethical and regulatory standards, and driving more value. Although the journey may not be easy, confidently embarking on it and tailoring the approach to your organization's needs can lead to great success.
Forbes Technology Council is an invitation-only community for world-class CIOs, CTOs, and technology executives.