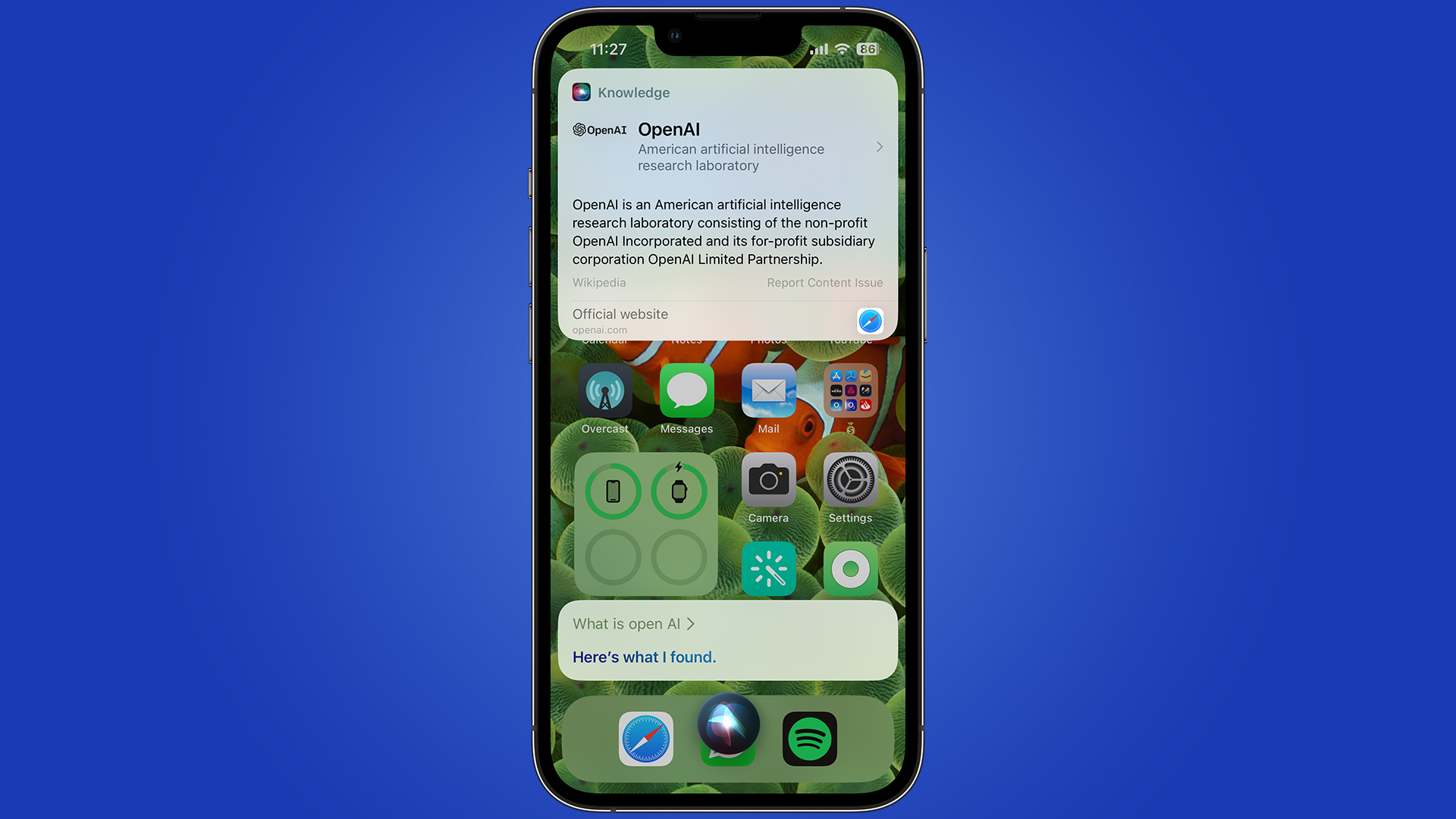
Apple announced iOS 18 at its WWDC developers conference on June 10. One of the biggest software updates we've ever seen, iOS 18 brings some incredible new features and more customization options to the iPhone than ever before. But the biggest addition is Apple Intelligence – Apple's set of AI features. All these features are powered by Apple's own AI models.
What makes these models so special is that they work entirely on your device, rather than sending things to servers. Now, we know a little bit more about how Apple trained these AI models. Per an official Apple research paper, the tech giant trained its models using Google's custom chips rather than hardware from Nvidia.
It turns out, Apple ditched Nvidia and instead opted for Google’s TPUv4 and TPUv5 chips to churn through the mountains of data needed for their Apple Intelligence Foundation Language Models (AFMs). These AFMs are the brains behind the flashy Apple Intelligence features that are starting to roll out to developers.
How Apple trained its AI models on Google chips
Apple’s main LLM (large language model), had the muscle of 8,192 TPUv4 chips working in unison. Picture it as eight slices of 1,024 chips each. The training was very intense, involving a triple-stage process with trillions of tokens – 6.3 trillion to start, followed by a mere 1 trillion, and a final stretch with 100 billion tokens for context-lengthening.
The data buffet for these AFMs was pretty lavish too, with contributions from the Applebot web crawler (following robots.txt, mind you), various licensed datasets, and a sprinkle of public code, math, and other datasets for good measure.
The AFM-on-device model, the slimmer sibling designed for offline features, underwent some serious knowledge distillation. This model, a tidy 3 billion parameters, was distilled from the 6.4 billion parameter server model and trained using a single slice of 2,048 TPUv5p chips.
In terms of performance, Apple claims its AFM-server and AFM-on-device models are top-notch, excelling in benchmarks like Instruction Following, Tool Use, Writing, and more.