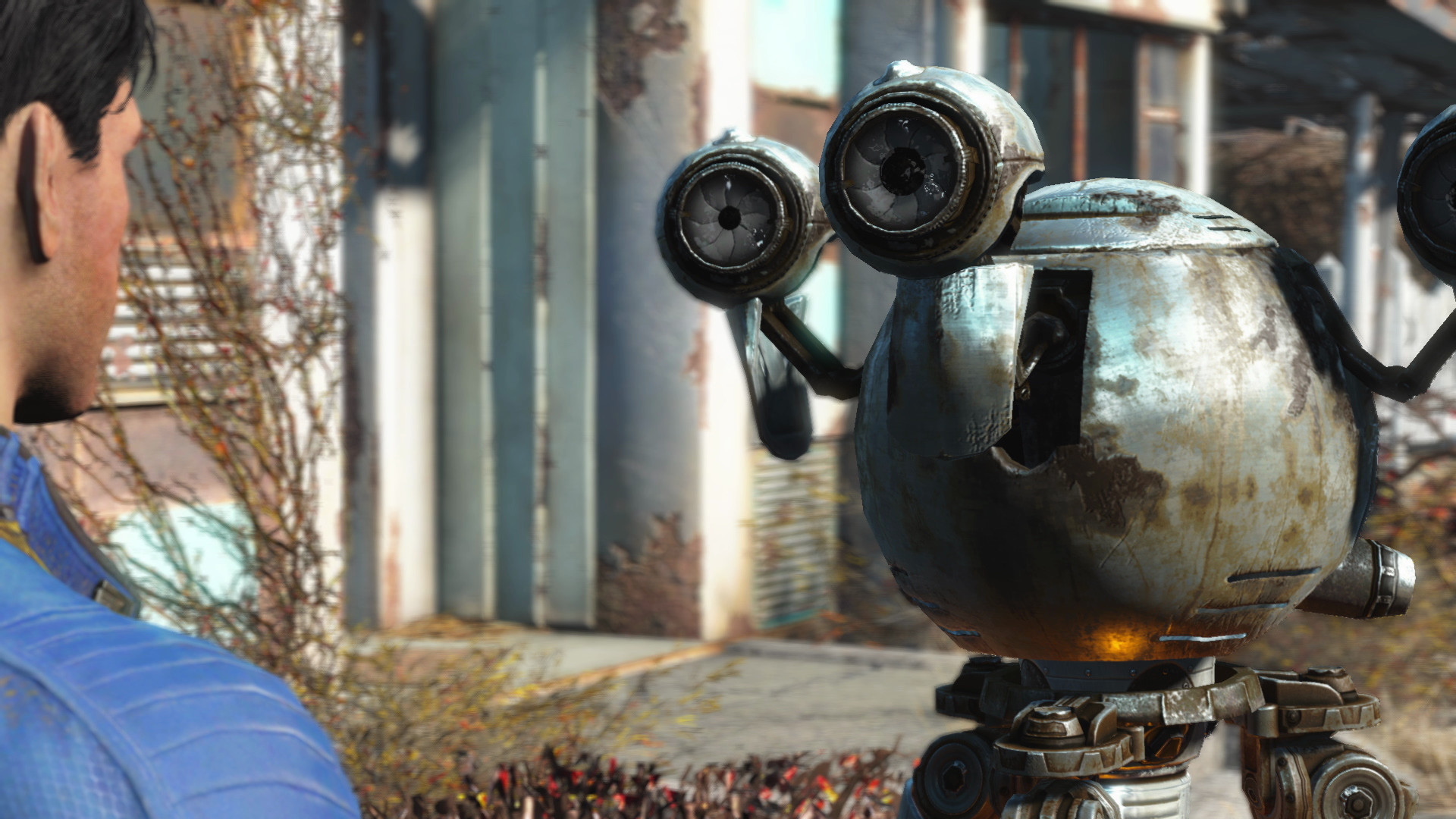
Like it or not, we're very much in the world of generative AI now. Massively complex neural networks trained on vast quantities of data, all so we can use it to make pictures of donkeys riding space rockets or tell us which churro coating is the best. I jest, of course, because large language models (LLMs) can be very useful but there's one area they've yet to be used in and that's robotics. Not anymore, as Google, the University of California, and a host of other labs around the world have started the RT-X project, with the aim of using AI to make an all-purpose 'brain' for robots.
Up to now, nobody seems to have really attempted this but it's only because the data used to train neural networks is based almost entirely on human endeavours, such as art, music, writing, and so on. As shocking as this may seem, the internet isn't full of data about robots and how well they carry out specific tasks.
Hence why Google and the University of California decided to set up the RT-X project (via Fudzilla), roping in 32 other robotics laboratories from around the world, to help them generate the kind of data required to train a neural network. That means collating data from millions and millions of robot interactions, doing such things as pick-and-place or welding in manufacturing lines.
The goal is to have a big enough dataset to create an LLM that can be used to produce the code required to program a robot to do any task. In essence, it's a general-purpose robot brain.
My own experiences of programming robot arms, from the days when I taught engineering, were primitive affairs, but I can easily see the appeal and potential of this work. Rather than manually coding everything yourself, the idea is that you'd type into the interface something along the lines of 'Put oranges in the grey box and leave apples alone.' The LLM would then handle the production of code required to do this.
By using specific inputs, such as a video feed from the robot's camera, the code would be automatically adjusted to account for not only the environment that the robot is in, but also what make and model of the robot is actually being used. The first tests of the RT-X model, as reported in IEEE Spectrum, were more successful than the best effort of the laboratory's coding.
The next steps were even more impressive. Human brains are exceptionally good at reasoning: Tell someone to pick up an apple and place it between a soda can and an orange on the table, and you'd expect them to do so without issue. Not so with robots and typically all of this would have to be directly coded into it.
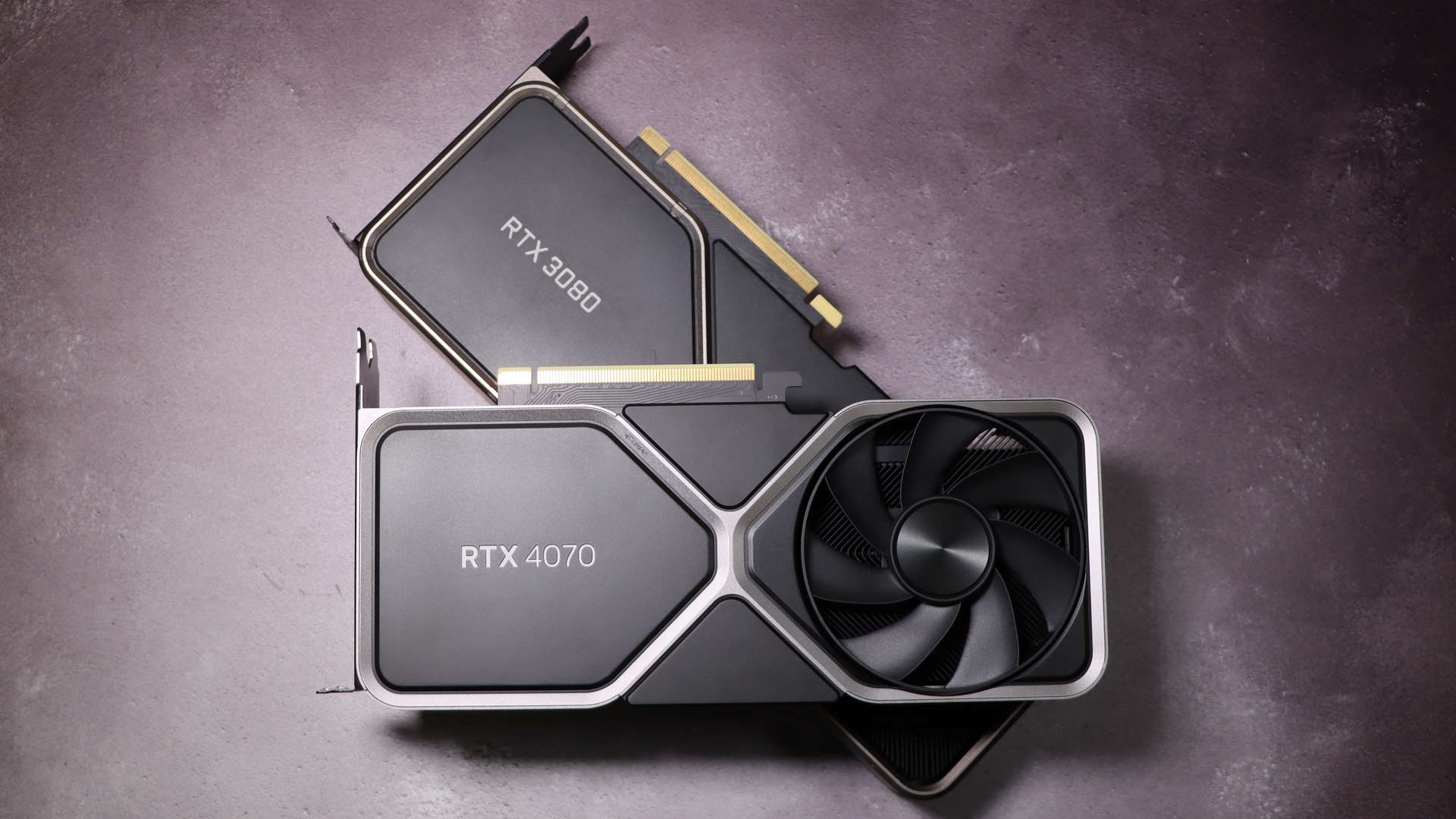
Best CPU for gaming: The top chips from Intel and AMD.
Best gaming motherboard: The right boards.
Best graphics card: Your perfect pixel-pusher awaits.
Best SSD for gaming: Get into the game ahead of the rest.
However, Google found that the LLM could 'figure it out', even though this specific task was never part of the neural network training dataset.
Although it's early days for the RT-X project, the benefits of generative AI are clear to see and the plan now is to expand the amount of training, from as many robotic facilities as possible, to produce a fully cross-embodiment LLM.
We're naturally cross-embodiment (i.e. our brains can be taught to do many complex tasks, such as playing a sport, riding a bike, or driving a car), but at the moment, robots are not even remotely so.
One day, though, we'll be able to go up to a drive-thru, order our food, and get exactly what we ordered and placed correctly into our hands! Now if that's not progress, I don't know what is. I can't wait to hail our AI mega-brained overlords…err…helpful robots.