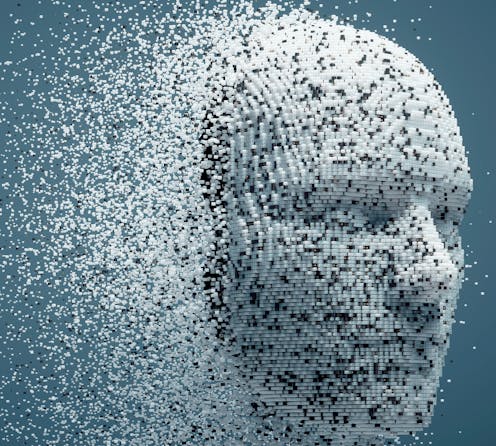
Justice systems around the world are using artificial intelligence (AI) to assess people with criminal convictions. These AI technologies rely on machine learning algorithms and their key purpose is to predict the risk of reoffending. They influence decisions made by the courts and prisons and by parole and probation officers.
This kind of tech has been an intrinsic part of the UK justice system since 2001. That was the year a risk assessment tool, known as Oasys (Offender Assessment System), was introduced and began taking over certain tasks from probation officers.
Yet in over two decades, scientists outside the government have not been permitted access to the data behind Oasys to independently analyse its workings and assess its accuracy – for example, whether the decisions it influences lead to fewer offences or reconvictions.
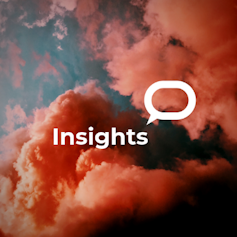
This article is part of Conversation Insights
The Insights team generates long-form journalism derived from interdisciplinary research. The team is working with academics from different backgrounds who have been engaged in projects aimed at tackling societal and scientific challenges.
Lack of transparency affects AI systems generally. Their complex decision-making processes can evolve into a black box – too obscure to unravel without advanced technical knowledge.
Proponents believe that AI algorithms are more objective scientific tools because they are standardised and this helps to reduce human bias in assessments and decision making. This, supporters claim, makes them useful for public protection.
But critics say that a lack of access to the data, as well as other crucial information required for independent evaluation, raises serious questions of accountability and transparency.
It also calls into question what kinds of biases exist in a system that uses data from criminal justice institutions, like the police, which research has repeatedly shown is skewed against ethnic minorities.
However, according to the Ministry of Justice, external evaluation poses data protection implications because it would require access to personal data, including protected characteristics such as race, ethnicity and gender (it is against the law to discriminate against someone because of a protected characteristic).
Oasys introduced
When Oasys was introduced in the UK in 2001 it brought with it sweeping changes to how courts and probation services assessed people convicted of crimes.
It meant that algorithms would begin having a huge influence in deciding just how much of a “risk” people involved in the justice system posed to society. These people include those convicted of a crime and awaiting punishment, prisoners and parole applicants.
Before Oasys, a probation officer would interview a defendant to try to get to the bottom of their offending and assess whether they were sorry, regretful or potentially dangerous. But post 2001 this traditional client-based casework approach was cut back and the onus was increasingly put on algorithmic predictions.
These machine learning predictions inform a host of decisions, such as: granting bail, outcomes of immigration cases, the kinds of sentences people face (community-based, custodial or suspended), prison security classifications and assignments to rehabilitation programmes. They also help decide the conditions on how people convicted of crimes are supervised in the community and whether or not they can be released early from prison.
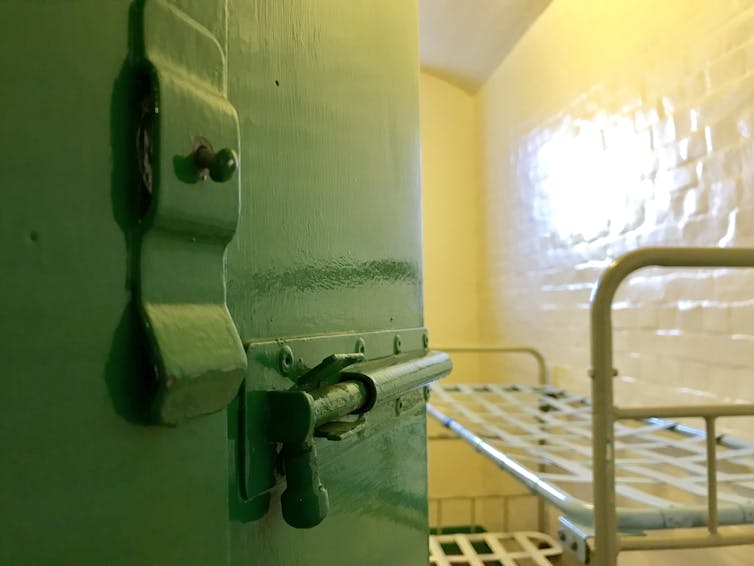
Some attempts at more rigorous risk assessments predate Oasys. The Parole Board in England and Wales deployed a re-conviction prediction score in 1976 which estimated the probability of a reconviction within a fixed period of two years on release from prison. Then, in the mid-1980s, a staff member with the Cambridgeshire Probation Service developed a rather simple risk prediction scale to provide more objectivity and consistency about predicting whether probation was an appropriate alternative to a custodial sentence. Both these methods were rather crude in terms of using only a handful of predictors and deploying rather informal statistical methods.
Harnessing computer power
Around this time, Home Office officials noticed the increased interest in the UK and the US authorities for developing predictive algorithms that could harness the efficiencies computers offered. These algorithms would support human opinions with scientific evidence about what factors were predictive of reoffending. The idea was to use scarce resources more effectively while protecting the public from people categorised as being at high risk of reoffending and causing serious harm.
The Home Office commissioned its first statistical predictive tool, which was deployed in 1996 across probation offices in England and Wales. This initial risk tool was called the Offender Group Reconviction Scale (OGRS). The OGRS is an actuarial tool in that it uses statistical methods to assess information about a person’s past (such as criminal history) to predict the risk of any type of reoffending.
The OGRS is still in use today after several revisions. And this simple algorithm has become incorporated into Oasys which has grown to incorporate additional machine learning algorithms. These have developed over time, predicting different types of reoffending. Reoffending is measured as reconviction within two years of release.
Oasys itself is based on the “what works” approach to risk assessment. Supporters of this method say it relies upon “objective evidence” of what is effective in reducing reoffending. “What works” introduced some basic principles of risk assessment and rehabilitation and it gained currency with governments around the world in the 1990s.
Risk factors can include “criminogenic needs” – these are factors in an offender’s life that are directly related to recidivism. Examples include, safe housing, job skills and mental health. The “what works” approach is based on several principles, one of which involves matching appropriate rehabilitation programmes to a person’s criminogenic needs.
So, a person convicted of a sex crime, with a history of alcohol abuse, might be given a sentence plan that includes a sex offender treatment programme and drug treatment. This is meant to reduce their likelihood of reoffending.
Following Home Office pilot studies between 1999 and 2001, Oasys was rolled out nationally and His Majesty’s Prison and Probation Service (HMPPS) have used the technology widely ever since.
What the algos do – scoring ‘risk’
The Offender Group Reconviction Scale and variations of Oasys are frequently modified and some information about how they work is publicly available.
The available information suggests that Oasys is calibrated to predict risk. The algorithms consume the data probation officers obtain during interviews and information in self-assessment questionnaires completed by the person in question. That data is then used to score a set of risk factors (criminogenic needs). According to the designers, scientific studies indicate that these needs are linked to risks of reoffending.
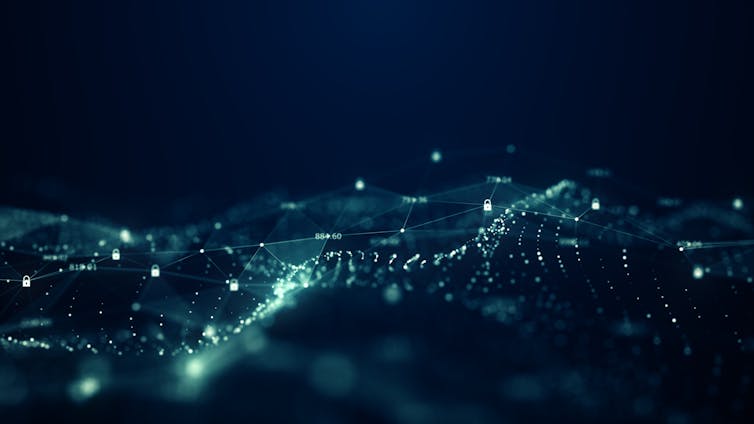
The risk factors include static (unchangeable) things such as criminal history and age. But they also comprise dynamic (changeable) factors. In Oasys, dynamic factors include: accommodation, employability, relationships, lifestyle, drugs misuse, alcohol misuse, thinking and behaviour, and attitudes. Different weights are assigned to different risk factors as some factors are said to have greater or lesser predictive ability.
So what type of data is obtained from the person being risk assessed? Oasys has 12 sections. Two sections concern criminal history and the current offence. The other ten address areas related to needs and risk. Probation officers use discretion in scoring many of the dynamic risk factors.
The person becomes a set of numbers
The probation officer may, for example, judge whether the person has “suitable accommodation”, which could require considering such things as safety, difficulties with neighbours, available amenities and whether the space is overcrowded. The officer will determine whether the person has a drinking problem or if impulsivity is an issue. These judgments can increase the person’s “risk profile”. In other words, a probation officer may consider dynamic risk factors like having no fixed address and having a history of drug abuse, and say that the person poses a higher risk of reoffending.
The algorithms assess the probation officers’ entries and produce numeric risk scores: the person becomes a set of numbers.
These numbers are then recombined and placed into low-, medium-, high-, and very high-risk categories. The system may also associate the category with a percentage indicating the proportion of people who reoffended in the past.
However, there is simply no specific guidance on how to translate any of the risk of reoffending scores into actual sentencing decisions. Probation officers conduct the assessments and they form part of the pre-sentence report (PSR) they present to the court along with a recommended intervention. But it is left to the court to determine a sentence, in line with the provisions of the Sentencing Council.
There is no dataset available to us that directly links Oasys predictions to the decisions they are meant to inform. Hence, we cannot know what decision-makers are doing with these scores in practice.
The situation is muddier considering that multiple risk tools put out results in different ratings (as in high, medium, or low) for the same individual. That’s because the algorithms are predicting different offence types (general, violent, contact sexual and indecent images).
So a person can collect several different ratings. It could be the person is labelled high risk of any reoffending, medium risk of violent offending, and low risk of both sexual offending types. What is a judge to do with these seemingly disparate pieces of data? Probation officers provide some recommendations but the decision is ultimately left to the judge.
Impact on workloads and risk aversion
Another issue is that probation officers have been known to struggle with completing Oasys assessments considering the significant amount of time it takes for each person. In 2006, researchers spoke to 180 probation officers and asked them about their views on Oasys. One probation officer called it “the worst tax form you’ve ever seen”.
In a different study, another probation officer said Oasys was an arduous and time-intensive “box-ticking exercise”.
What can also happen is that risk-aversion becomes entrenched in the system due to the fear of getting it wrong. The backlash can be swift and severe if a person assessed as low risk commits a serious offence - there have been many high-profile media scandals that prove this. In a report for the Prison Reform Trust, one long-term prisoner commented:
They repeatedly go on about ‘risk’ but I realised many years ago that this has nothing to do with risk … it’s all about accountability, they want someone to blame should it all go wrong.
The fear of being blamed is not an idle one. A probation officer was reportedly sacked in 2022 for gross misconduct for rating Damien Bendall as medium risk rather than high risk after a conviction for arson. Bendall was released with a suspended sentence. Within three months, he murdered his pregnant partner and three children.
Jordan McSweeney, another convicted murderer, was released from prison in 2022 with an assessment of medium risk. Three days later, he raped and brutally killed a young woman walking home alone. A review of the case determined that he had been incorrectly assessed and should instead have been labelled high risk.
But unlike in the Bendall case where an individual probation officer was apparantly blamed, the chief inspector of probation, Justin Russell, explained:
Probation staff involved were … experiencing unmanageable workloads made worse by high staff vacancy rates – something we have increasingly seen in our local inspections of services. Prison and probation services didn’t communicate effectively about McSweeney’s risks, leaving the Probation Service with an incomplete picture of someone who was likely to reoffend.
‘Bias in, bias out’
Despite its widespread use there has been no independent audit examining the kind of data Oasys relies on to come to its decisions. And that could be a problem - particularly for people from minority ethnic backgrounds.
That’s because Oasys, directly and indirectly, incorporates socio-demographic data into its tools.
AI systems, like Oasys, rely on arrest data as proxies for crime when they could in some cases be proxies for racially biased law enforcement (and there are plenty of examples in the UK and around the world of that). Predicting risks of reoffending on the basis of such data raises serious ethical questions. This is because racially biased policing can permeate the data, ultimately biasing predictions and creating the proverbial “bias in, bias out” problem.
In this way, criminal history records open up avenues for labelling and punishing people according to protected characteristics, like race, giving rise to racially biased outcomes. This could mean, for example, a higher percentage of minorities rated in the higher risk groups than non-minorities.
Another source of bias could stem from the way officers “rate” ethnic minorities when answering Oasys-led questions. Probation officers may assess minority ethnic people differently on questions such as, whether they have a temper control problem, are impulsive, hold pro-criminal attitudes, or recognise the impact of their offending on others. Unconscious biases could be at play here resulting from cultural differences in how various ethnic groups perceive these issues. For instance, people from one cultural background may “see” another person with a bad temper whereas that would be seen as acceptable emotional behaviour in another cultural background.
Read more: How can black people feel safe and have confidence in policing?
In its review of AI in the justice system in 2022, the justice and home affairs committee of the House of Lords noted that there are “concerns about the dangers of human bias contained in the original data being reflected, and further embedded, in decisions made by algorithms”.
And it’s not just the UK where such issues have arisen. The problem of racial bias in justice systems has been noted in various countries where risk assessment algorithms similar to Oasys are deployed.
In the US, the Compas and Pattern algorithms are used widely, and the Level of Service family of tools have been taken up in Australia and Canada.
The Compas system, for instance, is an AI algorithm used by US judges to make decisions on granting bail and sentencing. An investigation claimed that the system generated “false positives” for black people and “false negatives” for white people. In other words, it suggested that black people would reoffend when, in reality, they did not and suggested that white people would not reoffend when they actually did. But the developer of the system has challenged these claims.
Read more: AI: why installing 'robot judges' in courtrooms is a really bad idea
Studies suggest that such outcomes stem from racially biased decision making embedded in the data which the developers select to represent the risk factors that will determine the algorithm’s predictions. Criminal history data, such as police arrest records, is one example.
Other socio-economic data that developers select to represent risk factors may also be problematic. People will score as being higher risk if they do not have suitable accommodation or are unemployed. In other words, if you are poor or disadvantaged the system is stacked against you.
People are also classed as “high risk” for personal circumstances which are sometimes beyond their control. Risk factors include “not having a good relationship with a partner” and “undergoing psychiatric treatment”.
Meanwhile, a report issued by Her Majesty’s Inspectorate of Probation in 2021 alludes to the problem of conscious and unconscious biases which can enter the process via probation officers’ assessments, thereby infecting the outcomes.
More transparency could be useful for tracking when and how probation officer discretion has potentially tainted the final assessment, which could have resulted in people being incarcerated unnecessarily or being allocated inappropriate treatment programmes. This could result in flawed risk predictions.
For example, the report states:
It is impossible to be free from bias. How we think about the world and consider risk is intrinsically tied up with our emotions, values and tolerance (or otherwise) of risk challenges.
Social engineering?
Miklos Orban, visiting professor at the University of Surrey School of Law, recently engaged with the Ministry of Justice seeking information on Oasys. One of us (Melissa) spoke with Orban about this and he expressed concerns that the system might be a form of social engineering.
He said that governmental officials were eliciting personal and sensitive information from defendants who may think they are making these disclosures to get help or sympathy. But the officers may instead use them for another purpose, such as labelling them with a drinking or drugs problem and then requiring them to go on a suitable treatment programme. He said:
As a convict, you know very little of how risk assessment tools work, and I have my doubts as to how well judges and parole officers understand statistical models like Oasys. And that’s my number one concern.
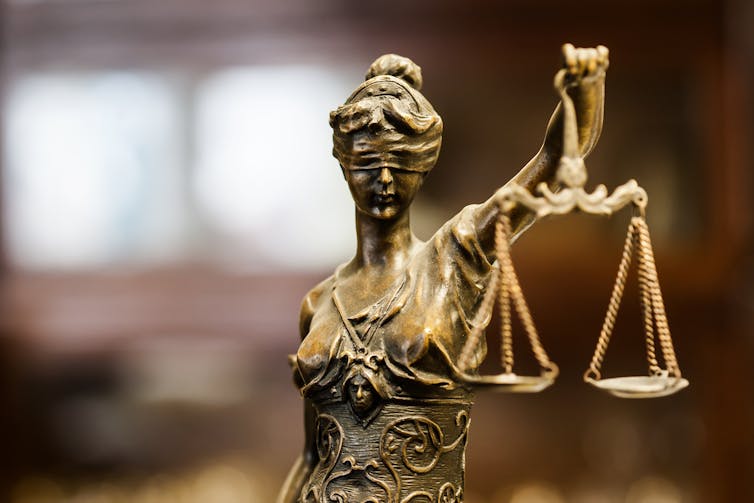
Not much is known about the accuracy of Oasys in relation to gender and ethnicity either. One available study (though a bit dated as it looked at a sample from 2007) shows the non-violent and violent predictive tools are less accurate with women and minority ethnic people.
Meanwhile, Justice, a legal reform organisation, recently cited a lack of research on the accuracy of these tools for women and trans prisoners.
In terms of racial bias, an HM Inspectorate of Prisons’ audit found that an Oasys assessment had not been completed or reviewed in the prior year for almost 20% of black and minority ethnic prisoners.
This is a serious issue because further evaluation can help ensure that minority ethnic people are receiving similar treatment or being assigned to helpful programming. It can avoid probation officers simply assuming the risk status of minority ethnic people is unchangeable and thus reduce their chances of early release since Oasys assessments are required to ascertain whether interventions have reduced risks of reoffending.
Researchers with the Inspectorate of Probation encouraged designers of Oasys to expand the ways it can incorporate a person’s personal experiences with discrimination and how it may impact their relationship with the criminal justice system. But, so far, and to the best of our knowledge, this has not been done.
Algorithms affect real people
Oasys results follow a person’s path through the criminal justice system and could influence key decisions from sentencing to parole eligibility.
Such serious decisions have huge consequences on peoples’ lives. Yet officials can decline to disclose Oasys results to the defendant in question if they are thought to contain “sensitive information”. They can ask and be shown their completed assessment, but they are not guaranteed to see it.
Even if they are given their scores, defendants and their lawyers face significant hurdles in understanding and challenging their assessments. There is no legal obligation to publish information about the system, although the Ministry of Justice has commendably made certain information public.
Still, even if more data were released, defence lawyers may not have the scientific skills to examine the assessments with a sufficiently critical eye.
Some prisoners describe additional challenges. They complain that their risk scores do not reflect how they see themselves. Others believe that their scores contain errors. While some also feel that Oasys mislabels them. In another report compiled by the PRT, one prisoner stated: “Oasys is who I was, not who I am now.”
And a man serving a life sentence described the repeated risk assessment when he spoke to a researcher at the University of Birmingham:
I have likened it to a small snowball running downhill. Each turn it picks up more and more snow (inaccurate entries) until eventually you are left with this massive snowball which bears no semblance to the original small ball of snow. In other words, I no longer exist. I have become a construct of their imagination. It is the ultimate act of dehumanisation.
Not all judicial officers are impressed either. When asked about using a risk assessment tool that the state required, a judge in the US said: “Frankly, I pay very little attention to the worksheets. Attorneys argue about them, but I really just look at the guidelines. I also don’t go to psychics.”
There have been relatively few legal challenges to any of the risk assessment algorithms in use across the world.
But one case stands as an outlier. In 2018, the Supreme Court of Canada ruled in the case of Ewert v Canada that it was unlawful for the prison system to use a predictive algorithm (not Oasys) on Indigenous inmates.
Ewert was an Indigenous Canadian serving time in prison for murder and attempted murder. He challenged the prison system’s use of an AI tool to assess his risk of recidivism.
The problem was the lack of evidence that the particular tool was sufficiently accurate when applied to the Indigenous population in Canada. In other words, the tool had never been tested on Indigenous Canadians.
The court understood that there might be risk-relevant differences between Indigenous and non-Indigenous peoples as to why they commit crimes. But since the algorithms had not been tested on Indigenous people, its accuracy for that population was not known. Therefore, using the tools to assess their risks violated the legal requirement that information about an offender must be accurate before it can be used for decision making.
The court also noted that the over-representation of Indigenous people in the Canadian justice system was in part attributable to discriminatory policies.
Individual vs group risk
The feeling that the scores produced by risk assessment algorithms such as Oasys may not be properly personalised or contextualised finds merit when considering how predictive algorithms in general work.
They assess people and produce risk scores and this has a longer history in business. The lending industry uses algorithms to assess the creditworthiness of customers. Insurance companies deploy algorithms to generate quotes for car insurance. The insurance algorithms often use driving records, age and gender to determine the likelihood of claiming against the policy.
But an all too common and mistaken assumption is that algorithms can provide a prediction about the specific person. On the contrary, publicly available information shows that the algorithms rely upon statistical groups.
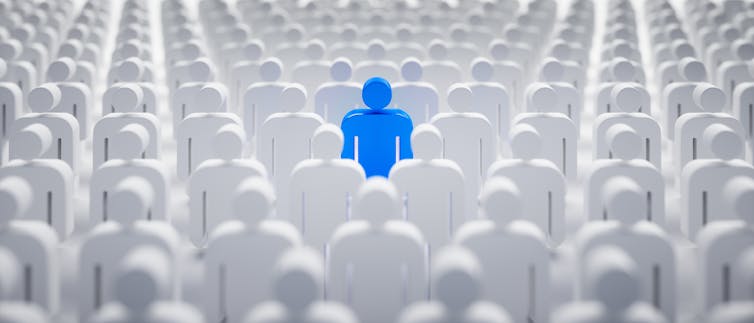
What does this mean? As we said earlier, they compare the circumstances and attributes of the person being risk assessed with risk factors and scores associated with criminal justice populations – or groups.
For example, what if “John” is placed in the medium-risk category, which is associated with a reoffending likelihood of 30%? This does not mean there is a 30% chance that John will reoffend. Instead, it means that about 30% of those assigned medium risk are forecasted to reoffend based on the observation that 30% of the medium risk had in the past been reconvicted.
This number cannot be directly assigned to any individual within that medium-risk group. John may, individually, have a 1% chance of reoffending. The scales are not individualised in this way and so John, himself, cannot be assigned specifically with a number.
The reason for this is that the predictive factors are not causal in nature. They are correlated, meaning there may be some relationship between the factors and reoffending. Oasys uses male gender as one of the predictive factors of reoffending. But being male does not cause reoffending. The relationship as perceived by Oasys merely suggests that males are more likely to commit crimes than females.
There are important consequences to this. The individual can thereby be seen as being punished, not for what he or she is personally predicted to do. They face imprisonment because of what others – who share a similar risk score – have done.
This is why more transparency of predictive algorithms is needed.
But even if we know what the inputs are, the weighting system is often obscure as well. And developers are frequently changing the algorithms for a host of reasons. The purposes may be valid. It could be that predictors of reoffending change over time in connection with societal shifts. Or it could be that new scientific knowledge suggests a modification is necessary.
Nevertheless, we have been unable to discover much about how well the Oasys system, or its components, performs. The Ministry of Justice has, to our knowledge, only released retroactive results.
Those statistics cannot inform on the predictive performance of the tool for predictions made today, or for how accurate they are when we relook at the offenders in two years. Frequent retrospective results are needed to provide up to date information on the performance of algorithms.
Independent evaluation
To the best of our knowledge (and to the knowledge of other experts in the field), Oasys has not been independently evaluated. There is a clear need for more information on the effectiveness and accuracy of these tools and their impact on gender, race, disability and other protected characteristics. Without these sources it is not possible to fully understand the prospects and challenges of the system.
We acknowledge that the lack of transparency surrounding Oasys is a common, though not universal, denominator that unites these types of algorithms deployed by justice systems and other sectors across the world. A court case in the state of Wisconsin that challenged the use of a risk assessment tool that the developer claimed was confidential succeeded only to a point.
The defendant, convicted of charges related to a drive-by shooting, claimed that it was unfair to use a tool which used a private algorithm because it prevented him from challenging its scientific credentials. The US court ruled that the government did not have to reveal the underlying algorithm. However, it required authorities to issue warnings when the tool was used.
These warnings included:
the fact that failure to disclose meant it was not possible to tell how scores were determined
the algorithms were group-based assessments incapable of individualised predictions
there could be biases toward minority ethnic people
the tool had not been tested for use in the state of Wisconsin.
Opening up the black box
Problems such as AI bias and lack of transparency are not peculiar to Oasys. They affect many other data-driven technologies deployed by public sector agencies.
In response, UK government agencies, such as the Central Digital and Data Office and the Centre for Data Ethics and Innovation (CDEI) have recognised the need for ethical approaches to algorithm design and implementation and have introduced remedial strategies.
A recent example is the Algorithmic Transparency Recording Standard Hub which offers public sector organisations the opportunity to provide information about their algorithms.
A relatively recent report published by the CDEI also discussed bias-limitation measures, such as reducing the significance of things like arrest history as they have been show to be negative proxies for race.
A post-prediction remedy in the CDEI report requires practitioners to lower the risk classification allocated to people belonging to a group known to be consistently vulnerable to higher risk AI scores than others.
More generally, researchers and civil society organisations have proposed pre and-post implementation audits to test, detect and resolve AI problems of the kind associated with Oasys.
The need for appropriate regulation of AI systems including those deployed for risk assessment has also been recognised by key regulatory bodies in the UK and around the world, such as Ofcom, the Information Commissioner’s Office and the Competition and Markets Authority.
When we put these issues to the MOJ, it said the system had been subject to external review, but it was not specific on the data. It said it has been making data available externally through the Data First programme and that the next dataset to be shared with the programme will be “based on” the Oasys database and released “within 12 months”.
An MOJ spokesperson added: “The Oasys system has been subject to external review and scrutiny by the appropriate bodies. For obvious reasons, granting external access to sensitive offender information is a complex process, which is why we’ve set up Data First which allows accredited researchers to access our information in an ethical and responsible way.”
In the end, we recognise that algorithmic systems are here to stay and we acknowledge the ongoing efforts to reduce problems with accuracy and bias.
Better access to, and input from, external experts to evaluate these systems and put forward solutions would be a useful step towards making them fairer.
The justice system is vast and complex and technology is needed to manage it. But it is important to remember that there are people behind the numbers.

For you: more from our Insights series:
Invisible Windrush: how the stories of Indian indentured labourers from the Caribbean were forgotten
To hear about new Insights articles, join the hundreds of thousands of people who value The Conversation’s evidence-based news. Subscribe to our newsletter.

The authors do not work for, consult, own shares in or receive funding from any company or organization that would benefit from this article, and have disclosed no relevant affiliations beyond their academic appointment.
This article was originally published on The Conversation. Read the original article.