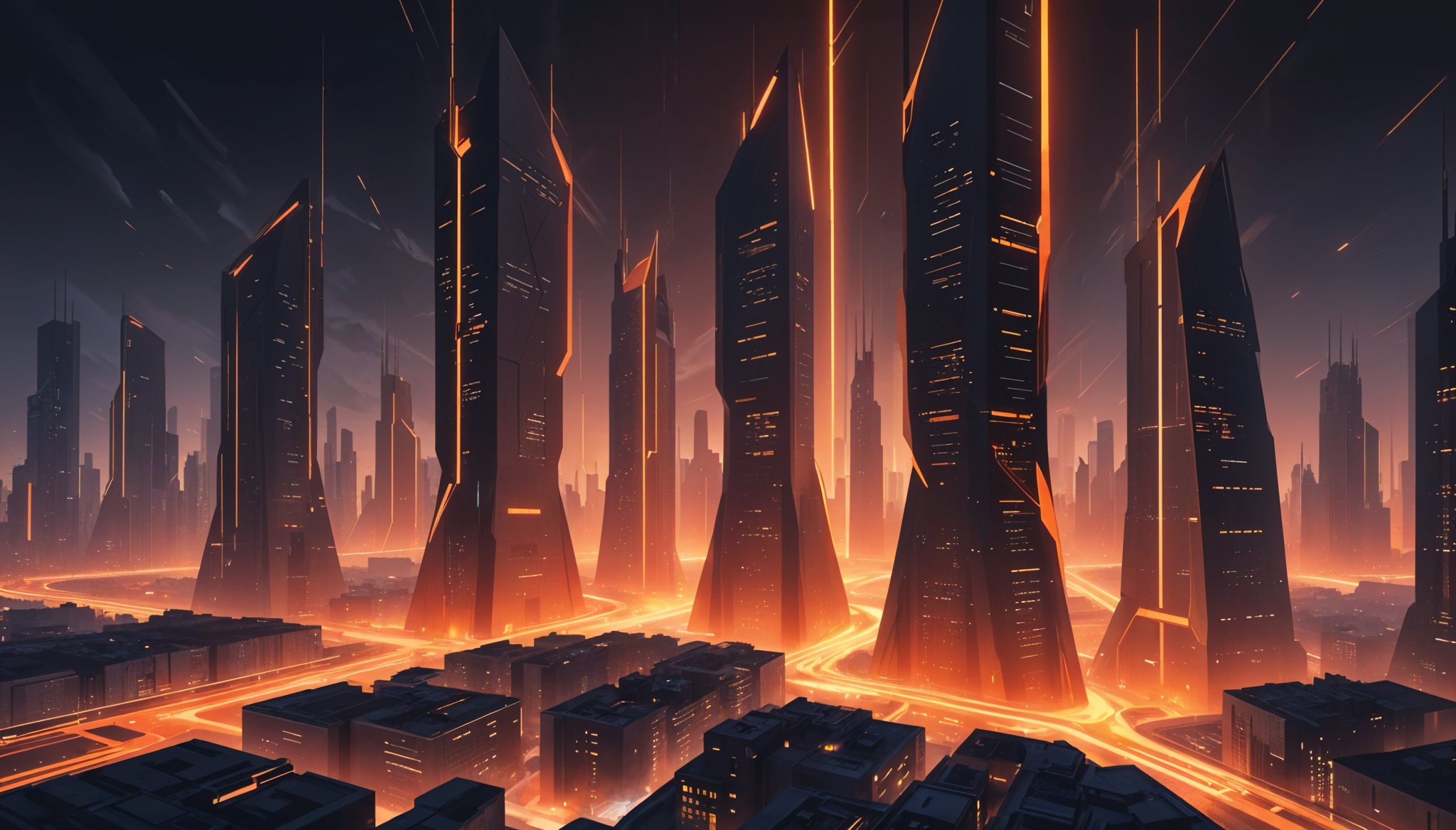
The growth of data center infrastructure over the past decade has shown an increasing demand for distributed services and coordinated multi-site deployments designed to eliminate downtime and provide failover contingency.
With the AI revolution now in full swing, both the technology now deployed into data centers and the types of installations are undergoing a vast change. Structural changes in the way data centers are designed and constructed will be significant, and where these sites are located will be driven by environmental factors.
What will the data centers of the future look like, and why will it not be anything seen from the hyper-scale era?
1. Massive growth is coming
Even without AI, data center numbers and power consumption were predicted to grow significantly in 2024. Based on widely distributed figures, data centers consumed 460 terawatt-hours (TWh) of electricity in 2022, or 2% of global electricity usage. In 2023, that grew by 55% to 7.4 GW (gigawatt = a billion watts).
It's predicted that hyperscale, AI and crypto data centers will all continue to grow almost exponentially, with Goldman Sachs Research publishing estimates that power demand will grow 160% by 2030. That would put data centers close to 4% of the entire global power use.
Even before AI, these numbers were on an upward trend, but AI is set to increase the number and scale of those deployments in a dramatic fashion.
What differentiates AI data centers from those in conventional hyper-scale environments is the demands on power. It's best to consider that most AI deployments have similar storage demands, but the compute power demands are substantially greater.
Therefore, an AI data center will need to be designed with access to power resources, the management of the heat generated from operations and the impact of that on the local environment.
The limiting factors in this are patently the availability of power and the reliability of those power services, since most power networks don't have substantial headroom in their supply profiles with overall demand increasing and most countries trying to end reliance on fossil fuels.
The United States sees itself as well-placed to cope with the AI revolution since it has plenty of space to build data centers away from urban areas, something that Europe will find more challenging.
However, without the unified power strategy or network that most European countries take for granted, the USA isn't the perfect choice for offering reliable power.
Therefore, while we'll see substantial growth in data centers built for hosting AI, issues regarding physical placement, power demands, and thermal management might all provide a retarding force on the speed of these changes.
Locations with access to renewable power from solar, wind, and hydropower will be paramount, along with development land, internet trunking, and the technology to mitigate the heat created, which will be the keys to project viability.
2. New types of facilities
Cloud data centers of the past generally mixed storage capacity with localised processing to provide a means to process data sets and serve them to client front ends, typically web customer-facing environments. These mainly differed in the scale of the services offered and the exact blend of storage and processing power they offer. Scalability was often about increased drive sizes and intentionally unused racks, and the computing platforms weren't replaced regularly.
Many enterprise customers didn't like the lack of control that cloud providers offered them over these general-purpose hyper-scale facilities, and many changed to in-house data centers that could more cost-effectively support the exact needs of their business.
AI data centers are likely to be much more complex, and depending on the hosted models that might be working with generative or predictive networks, optimisation for the specific workload will be critical to them converting power into valuable data.
AI data centers are different in several critical ways, as they can be either a prototyping environment or a deployment hub. A development environment is where an AI dataset is formed and tweaked, but it won't be where it is deployed.
These development AI data centers can be located anywhere in the world, and they will manage AI models from the prototyping stage through to deployment. Considering the security and containment issues associated with AI development, they might even be intentionally disconnected from the Internet.
Deployment AI data centers are where those data sets generated in the development centers will be deployed, and these might need to be placed closer to the point of service if latency is a factor.
To explain why, imagine an AI dataset developed to automate road traffic. Data flowing from the vehicles about where they are and what they see will need to flow into the model, and the corresponding instructions for each need to be sent back, all within a timeframe where that data is still relevant.
Building a data center in California to manage vehicles in New York wouldn't work since the latency would be too significant to cope with the changes that might occur within each time slice. A local AI data center in New York would be needed to reduce the latency sufficiently.
Not all AI deployments are time-critical in this fashion, but some are, and these might spawn smaller localised deployments for specific purposes.
3. Superior thermal management
The watts that existing hyper-scale facilities consume a becoming a major concern for climate scientists today, and AI is projected to increase the amount of electricity converted into heat.
Allowing AI to manage data centers based on local weather could significantly reduce consumption, pushing more tasks onto data centers in cooler locations rather than turning the air conditioning up during a summer heat wave.
New AI data centers also need to be built with heat in mind, using natural heat sinks like solid rock and the thermal advantages of more northerly (or southerly) latitudes.
Another approach might be to place a data center near a small settlement, take the excess heat, and distribute it as hot water or hot air for those living there to use in the winter. This would work similarly to how geothermal energy is used in Iceland, and planning permission for the facility might be tied to an agreement to provide cheap or free heat to residents.
Any data center needs to formulate a detailed thermal emissions plan, and blasting hot air into the Midwest of America in the summer won't make those businesses popular with people or investors.
Whatever approach is taken, the era of air-cooled data centers is coming to a close, and from this point forward, all new facilities are likely to be liquid-cooled, as it allows for the increased rack densities that AI brings.
4. Minimal headroom operations
With the massive outlay that an AI data center represents, getting the most from that facility is critical to achieving the full economic benefit of its construction and running.
As the manufacturing sector discovered with JIT (just-in-time), minimising the amount of waste within any production cycle is critical to the right balance in the profit and loss accounts.
Where in the past, having unused capacity in a facility was something of a selling point to potential customers, the exact opposite will be true with AI infrastructure.
The caveat to operating at maximum capacity for extended periods is increased temperatures and voltages. It will be necessary to regularly assess and potentially enhance the performance and design of cooling and electrical systems. Additionally, high-density racks necessitate integrating additional power and water into the facility to cope, further increasing the investment needed to bring these facilities online.
The increased maintenance and monitoring to keep running at high levels of efficiency will also necessitate a local engineering workforce operating 24/7. The era where a data center can be largely unmanned, with the exception of security personnel, and administered by an engineer half a continent away will be over.
In a scenario familiar with the repair and overhaul plans that airlines execute on their aircraft, AI data centers will need regular and time-critical attention to work optimally for extended periods of time. And, with the regular appearance of new and more efficient AI technology, upgrade cycles will become commonplace.
All the big chip players realise the potential of successful AI chips. AMD, Intel, Nvidia, and Qualcomm have all invested billions of dollars in designing supporting technology, and some of these businesses have effectively staked their futures on it.
5. AI running AI
The final irony of AI data centers is that engineers who are experts in hyper-scale deployments are currently designing them. But in the future, AI will be used to design these facilities and adapt them to the ongoing workload, along with managing the power, heat and delivery of the service they provide.
With AI running an array of processing nodes and conventional hyper-scale data centers, it should be able to identify cost savings and efficiencies, making changes in seconds as to where a dataset should be processed or reside.
AI will also be at the heart of any security system, identifying physical or network intrusions, weaknesses, and tracking people within the facility. Unlike conventional antivirus models that pattern-match to identify threats, AI can use multiple sources of sensors, network traffic and video sources to provide a constantly adapting defensive posture to ensure uninterrupted running.
These developments might be seen as the prelude to a dystopian future, but what functions will be given over to AI and those powers that senior systems engineers retain will be critical in how future data centers will run.
The challenges
If the learning curve for AI data center deployment wasn't steep enough, there are a few other factors to consider that might prove highly challenging.
The biggest one is the speed and capacity of Internet backbone infrastructures, and how they don't remotely address the scale of AI data sets or even hyper-scale data.
A single node within an AI data center might have 250PB (petabytes) in it, all generated by the AI processing model, and the data center might potentially have hundreds of these. If that data needed to be relocated to a new facility, perhaps on the other side of the continent, how long would it take to move it over the Internet? We are literally talking months.
Ironically, it would be faster to unbolt the hardware from the floor, put it on a truck and carry it to the new location than to network the data.
In short, the Internet will need to become a magnitude faster than it is now so that an AI dataset can be developed in a prototyping environment and sent to its deployment site quickly and efficiently.
Some help will come from allowing AI to manage the networks, where it can tune the available bandwidth to extract the most from it using and tune networks with intent-based network (IBN) methodologies. However, in short order, these backbones must be much more comprehensive, and possible alternative routes more numerous.
Therefore, the changes that AI will bring about inside data centers will be unprecedented but maybe minor compared to the impact of these radical components within the extended global digital environment.